Beyond Imagination: How Generative AI is Reshaping Virtual Worlds
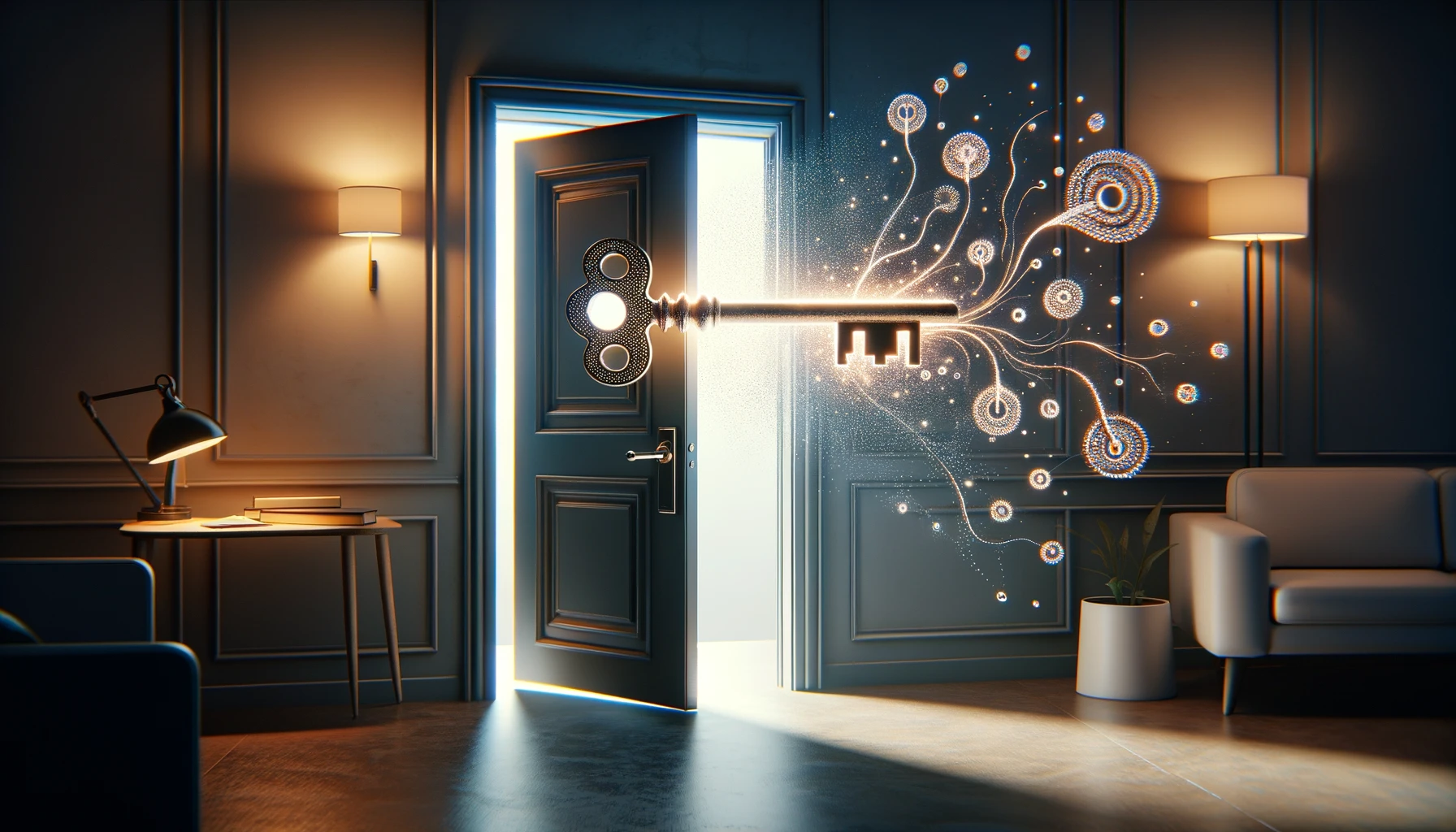
13/02/2024
Introduction to Generative AI
In the ever-evolving landscape of artificial intelligence (AI), Generative AI stands out as a transformative force driving innovation and creativity. Unlike traditional AI approaches focused on problem-solving and decision-making, Generative AI is dedicated to the creation of new content across various domains, including images, text, music, and even virtual environments. Its emergence marks a significant departure from conventional AI methodologies, offering new avenues for artistic expression, design innovation, and problem-solving.
As interest in AI and machine learning technologies continues to surge, fueled by advancements in computational power, availability of big data, and breakthroughs in deep learning algorithms, Generative AI has garnered widespread attention for its potential to unlock new possibilities in human-machine interaction and creative expression. From generating realistic images indistinguishable from photographs to composing music with human-like fluency and emotion, Generative AI is reshaping our perception of what machines are capable of achieving.
The Birth of Generative AI
The roots of Generative AI can be traced back to the foundational concepts laid out by pioneering mathematician and computer scientist Alan Turing in the mid-20th century. Turing's seminal work on computational theory and the concept of "universal machines" laid the groundwork for modern AI research and development. However, it wasn't until the advent of neural networks and deep learning techniques in the late 20th and early 21st centuries that Generative AI began to take shape as a distinct field of study.
Key milestones in the evolution of Generative AI include the introduction of Generative Adversarial Networks (GANs) and Variational Autoencoders (VAEs) in the early 2010s. GANs, proposed by Ian Goodfellow and his colleagues in 2014, consist of two neural networks – a generator and a discriminator – engaged in a competitive game where the generator aims to produce realistic data samples while the discriminator learns to distinguish between real and generated data. This adversarial training process enables GANs to generate highly realistic and diverse outputs, ranging from images and videos to music and text.
Similarly, VAEs, introduced around the same time, leverage probabilistic models and variational inference techniques to learn latent representations of data and generate new samples from the learned distribution. By encoding input data into a lower-dimensional latent space and decoding it back into the original data space, VAEs enable the generation of novel and diverse outputs while preserving the underlying structure of the data.
Core Technologies and Techniques
At the heart of Generative AI lie a diverse set of technologies and techniques, each tailored to specific tasks and applications. GANs, with their adversarial training framework, excel at generating realistic and high-fidelity data samples across various modalities, including images, videos, and audio. By leveraging the power of deep neural networks and game-theoretic principles, GANs have revolutionized image synthesis, enabling the creation of photorealistic images and videos that are indistinguishable from their real-world counterparts.
In contrast, VAEs offer a probabilistic approach to generative modeling, capturing the underlying distribution of data and enabling the generation of novel samples through latent space interpolation and sampling. By learning a low-dimensional representation of the input data, VAEs facilitate data compression, denoising, and generation, making them well-suited for tasks such as image reconstruction, anomaly detection, and data augmentation.
More recently, transformer architectures, such as the Transformer model introduced by Vaswani et al. in 2017, have emerged as a powerful framework for natural language processing (NLP) tasks. By leveraging self-attention mechanisms and multi-layered architectures, transformers excel at capturing long-range dependencies in sequential data and generating coherent and contextually relevant text. Models like OpenAI's GPT (Generative Pre-trained Transformer) have demonstrated remarkable capabilities in text generation, language translation, and dialogue generation, paving the way for new applications in conversational AI, content generation, and human-machine interaction.
Generative AI Across Industries
Generative AI holds immense promise across a wide range of industries, offering innovative solutions to complex problems and driving advancements in healthcare, entertainment, finance, art, and design.
In healthcare, Generative AI is revolutionizing drug discovery and personalized medicine, enabling researchers to generate novel molecular structures, predict drug interactions, and design targeted therapies for individual patients. By leveraging generative models to simulate molecular interactions and predict biological activity, researchers can accelerate the drug development process and identify promising candidates for clinical trials.
Moreover, Generative AI is transforming medical imaging analysis, where it assists radiologists in detecting and diagnosing diseases from imaging data such as X-rays, MRIs, and CT scans. By generating synthetic images and augmenting existing datasets, Generative AI enables more robust and accurate machine learning models for medical image interpretation, leading to improved diagnostic accuracy and patient outcomes.
In the entertainment industry, Generative AI is pushing the boundaries of creativity and storytelling, enabling filmmakers and game developers to generate realistic characters, environments, and visual effects with unprecedented ease and realism. By leveraging generative models to automate the content creation process, creators can iterate on design ideas, explore new artistic styles, and deliver immersive experiences to audiences.
Furthermore, Generative AI is driving innovation in finance, where it is applied in fraud detection, algorithmic trading, and risk management. By analyzing vast amounts of financial data and generating predictive models, generative algorithms can identify fraudulent transactions, optimize trading strategies, and assess various risks, including credit risk, market risk, and operational risk.
In the realm of art and design, Generative AI is empowering artists and designers to explore new creative possibilities and push the boundaries of traditional artistic practices. From generating digital art and interactive installations to designing bespoke fashion pieces and architectural structures, Generative AI offers a versatile tool for artistic expression and design innovation.
Advancements and Breakthroughs
In recent years, Generative AI has witnessed remarkable advancements and breakthroughs, driven by ongoing research and development efforts in academia and industry.
One notable advancement is the continuous improvement and refinement of Generative Pre-trained Transformer (GPT) models, developed by OpenAI. GPT models, based on the transformer architecture, have demonstrated impressive capabilities in natural language processing tasks, including text generation, language translation, and dialogue generation. With each new iteration, GPT models have achieved state-of-the-art performance on benchmark datasets and expanded their capabilities to support a wide range of applications in conversational AI, content generation, and human-machine interaction.
Another breakthrough is the development of models like DALL·E, introduced by OpenAI in 2021, which extends the capabilities of Generative AI beyond image synthesis to image generation from textual descriptions. By training on large-scale datasets of images and corresponding text descriptions, DALL·E can generate highly detailed and contextually relevant images based on textual prompts, opening up new possibilities for creative expression and content creation.
Moreover, Generative AI is making significant strides in music generation, where it is utilized to compose original melodies, harmonies, and rhythms that exhibit diverse styles and genres. By learning from vast collections of musical data, generative models can capture the underlying patterns and structures of music and generate new compositions that push the boundaries of traditional music theory and composition techniques.
Facts and Figures
The global AI market is projected to reach $190.61 billion by 2025, with Generative AI technologies playing a significant role in driving market growth and innovation. Investment in AI startups has surged in recent years, with venture capital funding flowing into companies developing Generative AI technologies and applications. Moreover, adoption rates of Generative
AI technologies are on the rise across various industries, as organizations recognize the transformative potential of AI-driven innovation and seek to gain a competitive edge in their respective markets.
Ethical and Societal Considerations
Despite its transformative potential, Generative AI also raises ethical and societal concerns that must be addressed to ensure responsible development and deployment. One of the key challenges is the potential for bias and unfairness in AI-generated content, as generative models may inadvertently perpetuate and amplify biases present in the training data. For example, biased representations of gender, race, and ethnicity in training data can lead to the generation of discriminatory or offensive content, perpetuating harmful stereotypes and inequalities.
Moreover, Generative AI raises concerns about the proliferation of deepfakes and misinformation, as malicious actors may exploit generative models to create realistic but fabricated content for deceptive purposes. Deepfake technology, which uses Generative AI algorithms to superimpose one person's face onto another person's body in videos, poses a significant threat to privacy, security, and trust in digital media.
Furthermore, there is a growing debate around copyright and creativity in AI-generated art and literature, as questions arise about the ownership and attribution of content created by generative models. While AI-generated works may exhibit creativity and originality, the question of whether they qualify as intellectual property and who holds the rights to such works remains a subject of legal and philosophical debate.
The Future of Generative AI
Looking ahead, the future of Generative AI promises continued innovation and disruption across various industries, as researchers and developers push the boundaries of what's possible with AI-driven creativity and intelligence. With advancements in deep learning algorithms, computational infrastructure, and data availability, Generative AI is poised to unlock new applications and use cases that were previously unimaginable.
One potential direction for Generative AI is the integration of multimodal capabilities, where models can generate content across multiple modalities, such as images, text, and audio, in a coherent and contextually relevant manner. Multimodal Generative AI models have the potential to revolutionize human-machine interaction, enabling more intuitive and natural communication between users and AI systems.
Moreover, Generative AI is expected to play a crucial role in addressing pressing societal challenges, such as climate change, healthcare disparities, and global poverty. By harnessing the power of AI-driven creativity and innovation, researchers and policymakers can develop new solutions and interventions that leverage Generative AI technologies to improve the quality of life for people around the world.
However, realizing the full potential of Generative AI requires careful consideration of ethical, legal, and societal implications, as well as the development of robust regulations and guidelines to ensure responsible development and deployment. By fostering interdisciplinary collaboration, promoting transparency and accountability, and prioritizing human-centric design principles, we can harness the transformative power of Generative AI to create a more inclusive, equitable, and sustainable future for all.
How to Get Involved
For individuals interested in getting involved in the exciting field of Generative AI, there are numerous resources, communities, and opportunities available to learn, collaborate, and contribute to the advancement of the field.
Online platforms such as Coursera, Udacity, and edX offer a wide range of courses and tutorials on AI and machine learning, including specialized courses on Generative AI techniques and applications. Additionally, open-source libraries and frameworks such as TensorFlow, PyTorch, and Hugging Face provide tools and resources for experimenting with Generative AI algorithms and building custom models for specific tasks.
Furthermore, joining online communities and forums, such as Reddit's r/MachineLearning and OpenAI's community forum, enables individuals to connect with peers, share knowledge and insights, and collaborate on research projects and competitions. Participating in hackathons, workshops, and conferences focused on Generative AI provides opportunities to showcase skills, network with industry professionals, and stay updated on the latest trends and advancements in the field.
For students and early-career professionals, pursuing advanced studies in AI, machine learning, and related disciplines opens doors to exciting opportunities in research, development, and innovation. Many universities and research institutions offer graduate programs and internships in AI and machine learning, providing hands-on experience and mentorship from leading experts in the field.
By actively engaging with the AI community, staying curious and informed, and seizing opportunities to learn and collaborate, individuals can make meaningful contributions to the advancement of Generative AI and help shape its future trajectory.
Conclusion
In conclusion, Generative AI represents a paradigm shift in artificial intelligence technology, offering new opportunities for creativity, innovation, and problem-solving across various domains. From generating realistic images and text to composing music and designing virtual worlds, Generative AI enables us to explore new frontiers of human-machine collaboration and expression.
However, realizing the full potential of Generative AI requires careful consideration of ethical, legal, and societal implications, as well as the development of robust regulations and guidelines to ensure responsible development and deployment. By fostering interdisciplinary collaboration, promoting transparency and accountability, and prioritizing human-centric design principles, we can harness the transformative power of Generative AI to create a more inclusive, equitable, and sustainable future for all.
Contact us
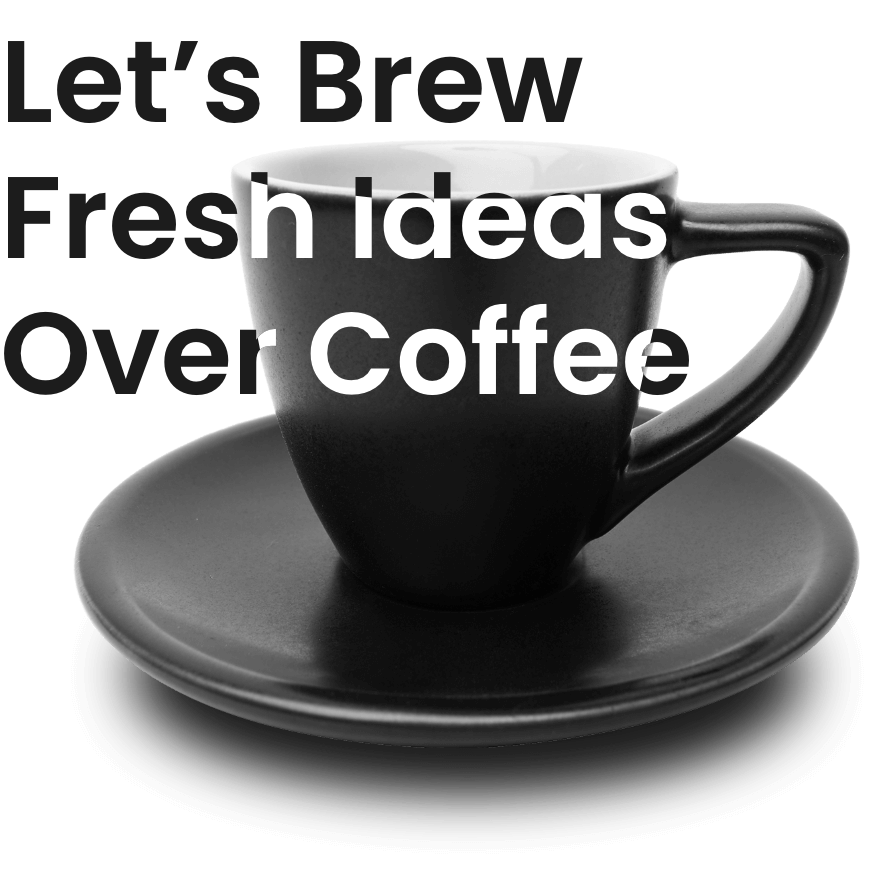
Spanning 8 cities worldwide and with partners in 100 more, we’re your local yet global agency.
Fancy a coffee, virtual or physical? It’s on us – let’s connect!