The impact of machine learning on fraud detection in ecommerce development services
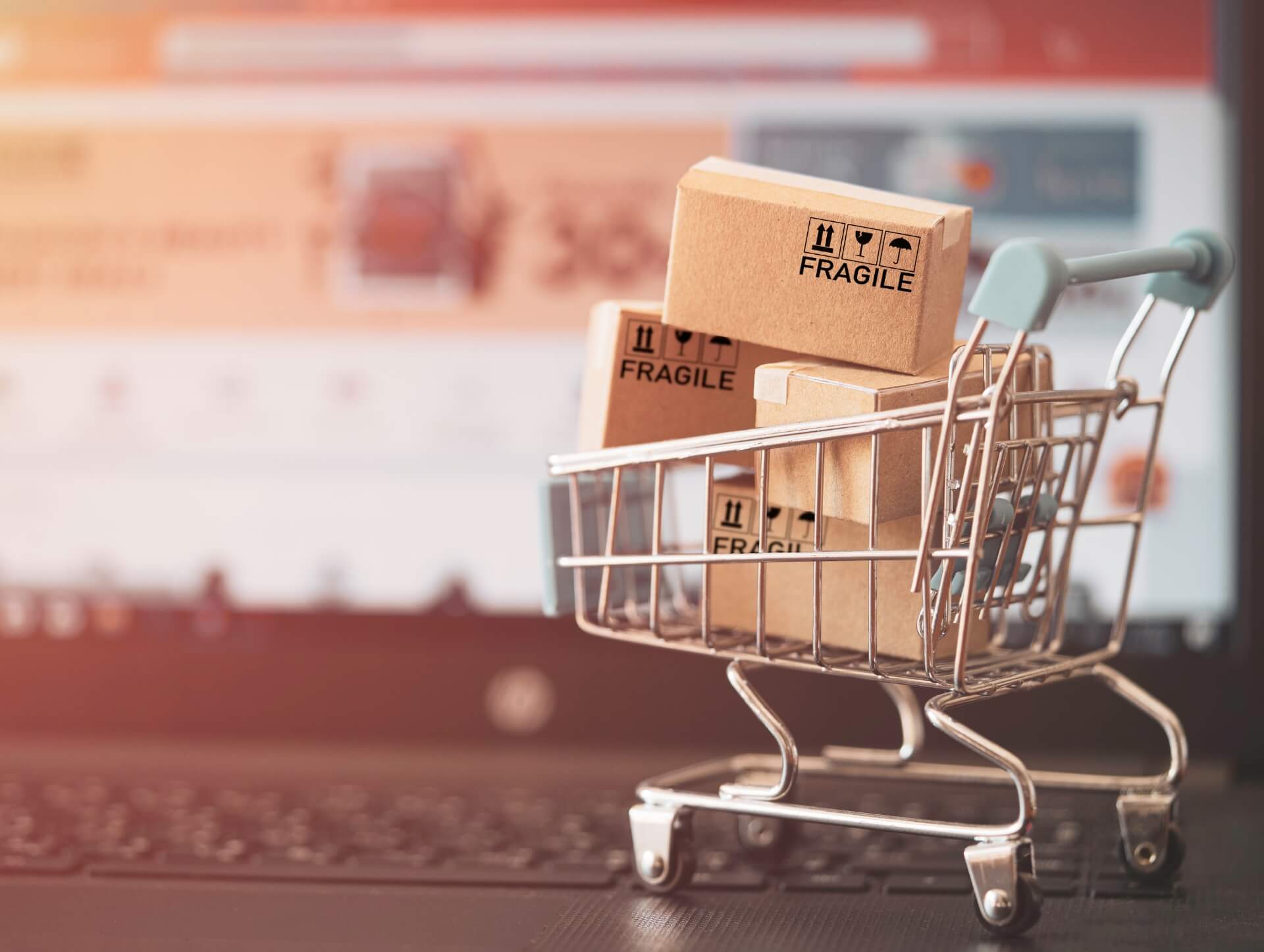
06/09/2023
Ecommerce development services have revolutionized the way businesses operate in the digital age. With the rise of online shopping, businesses are constantly looking for ways to provide a seamless and secure shopping experience for their customers. However, with the increase in online transactions, the risk of fraud has also grown exponentially. This is where machine learning comes into play. Machine learning algorithms have the potential to detect and prevent fraud in real-time, ensuring the safety and security of ecommerce platforms. In this article, we will explore the impact of machine learning on fraud detection in ecommerce development services and how it is changing the landscape of fraud prevention in the industry.
The Need for Fraud Detection in Ecommerce
Fraudulent activities in ecommerce can have severe consequences for businesses, including financial losses, damage to reputation, and loss of customer trust. Traditional fraud detection methods often rely on manual reviews and rule-based systems, which are time-consuming, inefficient, and prone to errors. With the increasing complexity and sophistication of fraud techniques, businesses need more advanced and automated solutions to stay one step ahead of fraudsters. Machine learning offers a promising solution by leveraging data and algorithms to detect patterns and anomalies indicative of fraudulent activities.
How Machine Learning Works in Fraud Detection
Machine learning algorithms learn from historical data to identify patterns and make accurate predictions. In the context of fraud detection, these algorithms analyze large volumes of transactional data to detect fraudulent patterns and behaviors. Here is a step-by-step process of how machine learning works in fraud detection:
Data Collection and Preprocessing
The first step in the machine learning process is to collect relevant data. In the case of fraud detection, this includes transactional data, user information, and other relevant data points. Once the data is collected, it needs to be preprocessed to remove any inconsistencies or errors. This step involves cleaning the data, handling missing values, and transforming the data into a suitable format for analysis.
Feature Extraction
After preprocessing the data, the next step is to extract relevant features that can be used to train the machine learning model. These features can include transaction amounts, time of day, location, user behavior, and more. Feature extraction is crucial in identifying patterns that distinguish fraudulent activities from legitimate ones.
Model Training
Once the features are extracted, the machine learning model is trained using labeled data. Labeled data consists of historical transactions that are labeled as either fraudulent or legitimate. The model learns from these labeled examples to identify patterns and make predictions on new, unseen data. The training process involves selecting an appropriate machine learning algorithm, tuning its parameters, and evaluating its performance.
Real-Time Fraud Detection
After the model is trained, it can be deployed to detect fraud in real-time. As new transactions occur, the model analyzes the data and assigns a fraud score to each transaction. Based on this score, businesses can take appropriate actions, such as flagging suspicious transactions for manual review or blocking them outright. The model can continuously learn and adapt to new fraud techniques, improving its accuracy over time.
The Benefits of Machine Learning in Fraud Detection
Machine learning offers several benefits when it comes to fraud detection in ecommerce development services:
Improved Accuracy
Machine learning algorithms can analyze large volumes of data and detect complex patterns that may not be apparent to humans. This leads to improved accuracy in detecting and preventing fraud, minimizing false positives and false negatives.
Real-Time Detection
Machine learning models can analyze transactions in real-time, providing immediate feedback and allowing businesses to take prompt action. This is crucial in preventing fraudulent activities before they cause significant damage.
Scalability
Machine learning algorithms can handle large amounts of data and scale to meet the needs of growing ecommerce platforms. As the volume of transactions increases, machine learning models can seamlessly adapt and continue to provide accurate fraud detection.
Adaptability
Machine learning models can continuously learn and adapt to new fraud techniques. They can detect emerging patterns and adjust their algorithms accordingly, ensuring that businesses stay ahead of fraudsters.
Challenges and Limitations of Machine Learning in Fraud Detection
While machine learning has shown great promise in fraud detection, there are also challenges and limitations that need to be considered:
Imbalanced Data
In fraud detection, the number of fraudulent transactions is often significantly lower than legitimate transactions. This leads to imbalanced data, where the machine learning model may be biased towards the majority class. Special techniques, such as oversampling or undersampling, need to be applied to address this issue.
Adversarial Attacks
Fraudsters are constantly evolving their techniques to bypass fraud detection systems. They may intentionally manipulate their behavior to appear legitimate and avoid detection. Machine learning models need to be robust against adversarial attacks and be able to detect subtle anomalies that may indicate fraudulent activities.
Privacy Concerns
Machine learning models require access to sensitive customer data to make accurate predictions. This raises privacy concerns and requires businesses to implement robust security measures to protect customer information.
Model Interpretability
Machine learning models, especially complex ones like deep neural networks, can be difficult to interpret. This lack of interpretability makes it challenging to understand how the model arrived at a particular decision, which may be crucial in fraud detection scenarios.
Conclusion
Machine learning has the potential to revolutionize fraud detection in ecommerce development services. By leveraging data and algorithms, machine learning models can analyze large volumes of transactions and detect fraudulent patterns in real-time. The benefits of machine learning in fraud detection include improved accuracy, real-time detection, scalability, and adaptability. However, there are also challenges and limitations that need to be addressed, such as imbalanced data, adversarial attacks, privacy concerns, and model interpretability. Overall, machine learning is changing the landscape of fraud prevention in the ecommerce industry, providing businesses with the tools they need to protect their platforms and customers from fraudulent activities.
Contact us
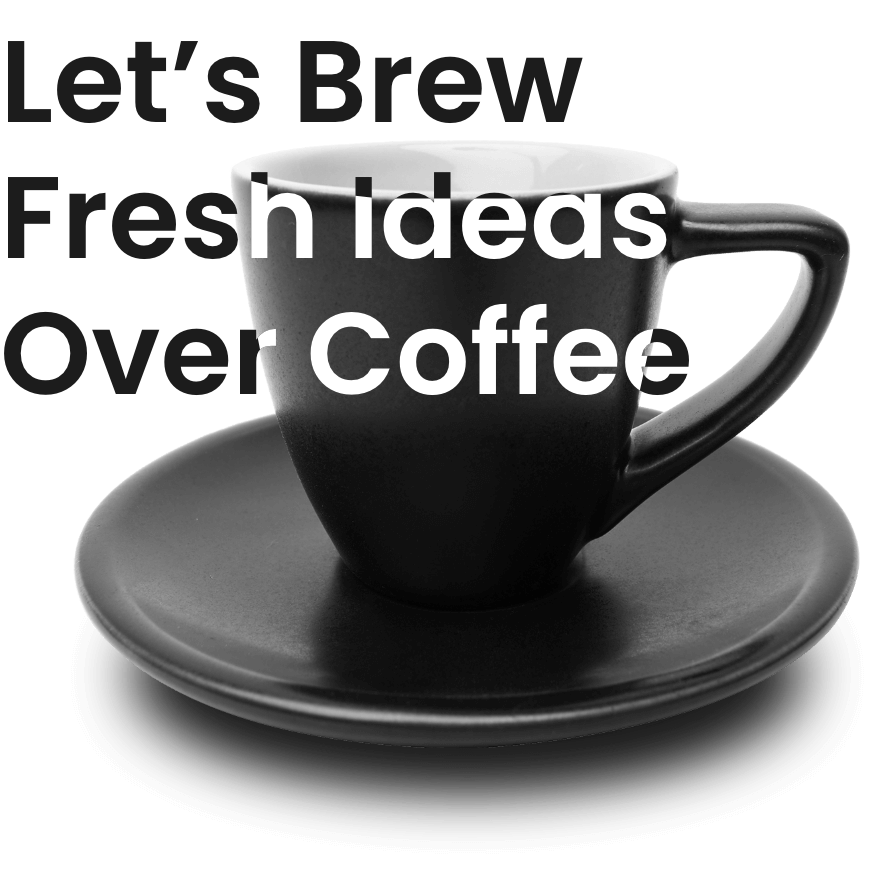
Spanning 8 cities worldwide and with partners in 100 more, we’re your local yet global agency.
Fancy a coffee, virtual or physical? It’s on us – let’s connect!