The impact of personalization algorithms on eCommerce analytics
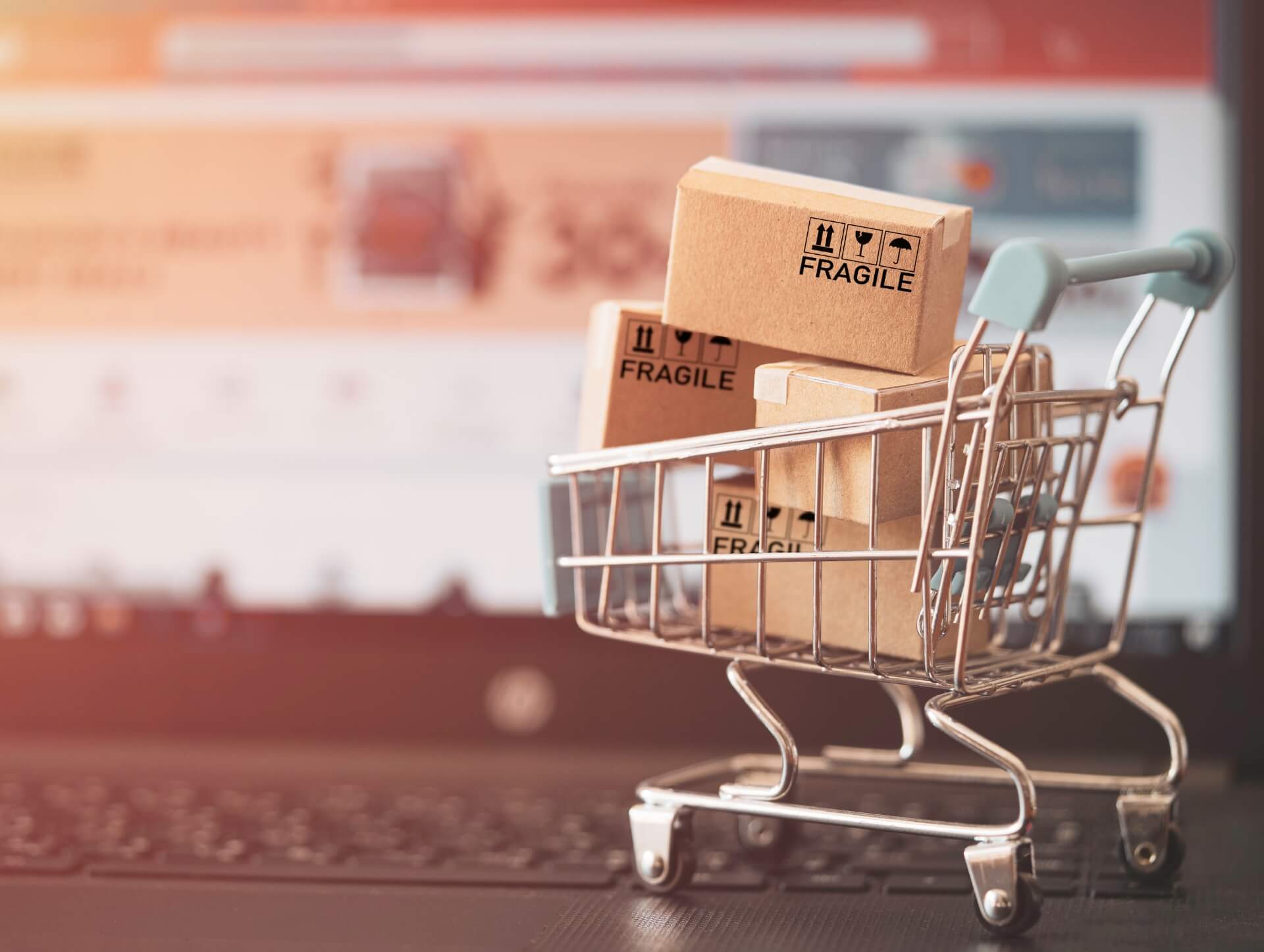
06/09/2023
In the world of eCommerce, data is king. Every click, every purchase, and every interaction generates valuable information that can be used to optimize the user experience and drive sales. However, with the rise of personalization algorithms, the way we analyze and interpret this data has changed.
What are Personalization Algorithms?
Personalization algorithms are complex mathematical models that use data collected from users to create personalized experiences. These algorithms analyze a wide range of factors, such as past purchase history, browsing behavior, demographics, and more, to deliver customized content and recommendations to each individual user.
The Impact on eCommerce Analytics
Personalization algorithms have a significant impact on eCommerce analytics, as they introduce new variables and considerations that must be taken into account when analyzing and interpreting data. Here are some key ways in which personalization algorithms affect eCommerce analytics:
1. Data Volume and Variety
Personalization algorithms generate a massive amount of data. From tracking user behavior to analyzing purchase patterns, the amount of information that needs to be processed and analyzed can be overwhelming. eCommerce analytics teams need to have the necessary infrastructure and tools in place to handle this influx of data and extract actionable insights.
2. Data Accuracy and Quality
Personalization algorithms heavily rely on accurate and high-quality data. Any inaccuracies or inconsistencies in the data can lead to flawed recommendations and personalized experiences. It is crucial for eCommerce businesses to have robust data collection processes in place and to regularly monitor and cleanse their data to ensure its accuracy and reliability.
3. Customer Segmentation
Personalization algorithms allow for more granular customer segmentation. Instead of relying on broad categories and segments, eCommerce businesses can now create highly targeted segments based on specific behaviors and preferences. This level of segmentation enables businesses to deliver more relevant and personalized experiences to their customers, but it also means that eCommerce analytics teams need to redefine their segmentation strategies and approaches.
4. Performance Measurement
With the introduction of personalization algorithms, traditional performance metrics may no longer be sufficient. Metrics such as conversion rate and average order value may not accurately reflect the impact of personalized experiences on customer behavior. eCommerce analytics teams need to develop new ways of measuring and evaluating performance to account for the unique challenges and opportunities presented by personalization algorithms.
Best Practices for Analyzing Personalized Data
While personalization algorithms introduce new complexities to eCommerce analytics, there are several best practices that businesses can follow to effectively analyze personalized data:
1. Define Clear Objectives
Before diving into the data, it is essential to define clear objectives and key performance indicators (KPIs). What specific outcomes are you looking to achieve through personalization? Are you aiming to increase conversion rates, boost average order value, or improve customer satisfaction? By clearly defining your objectives, you can focus your analysis on the metrics that matter most to your business.
2. Collect and Cleanse Data
Accurate and reliable data is the foundation of effective analysis. Ensure that you have robust data collection processes in place and regularly monitor and cleanse your data to remove any inaccuracies or inconsistencies. This will help you maintain the integrity of your analysis and ensure that your insights are based on reliable information.
3. Use Advanced Analytics Techniques
Traditional analytics techniques may not be sufficient when analyzing personalized data. Consider leveraging advanced analytics techniques such as machine learning and predictive modeling to uncover patterns and insights that traditional methods may overlook. These techniques can help you identify hidden opportunities and drive more impactful personalization strategies.
4. Test and Iterate
Personalization is an iterative process. Continuously test and evaluate your personalized experiences to understand what works and what doesn't. A/B testing and multivariate testing can help you optimize your personalization algorithms and ensure that you are delivering the most effective experiences to your customers.
Conclusion
Personalization algorithms have revolutionized the way we analyze and interpret eCommerce data. By leveraging the power of data and machine learning, businesses can create highly personalized experiences that drive customer engagement and boost sales. However, it is crucial for eCommerce analytics teams to adapt their strategies and approaches to account for the unique challenges and opportunities presented by personalization algorithms. By following best practices and staying ahead of the curve, businesses can unlock the full potential of personalized data and drive their eCommerce success.
Contact us
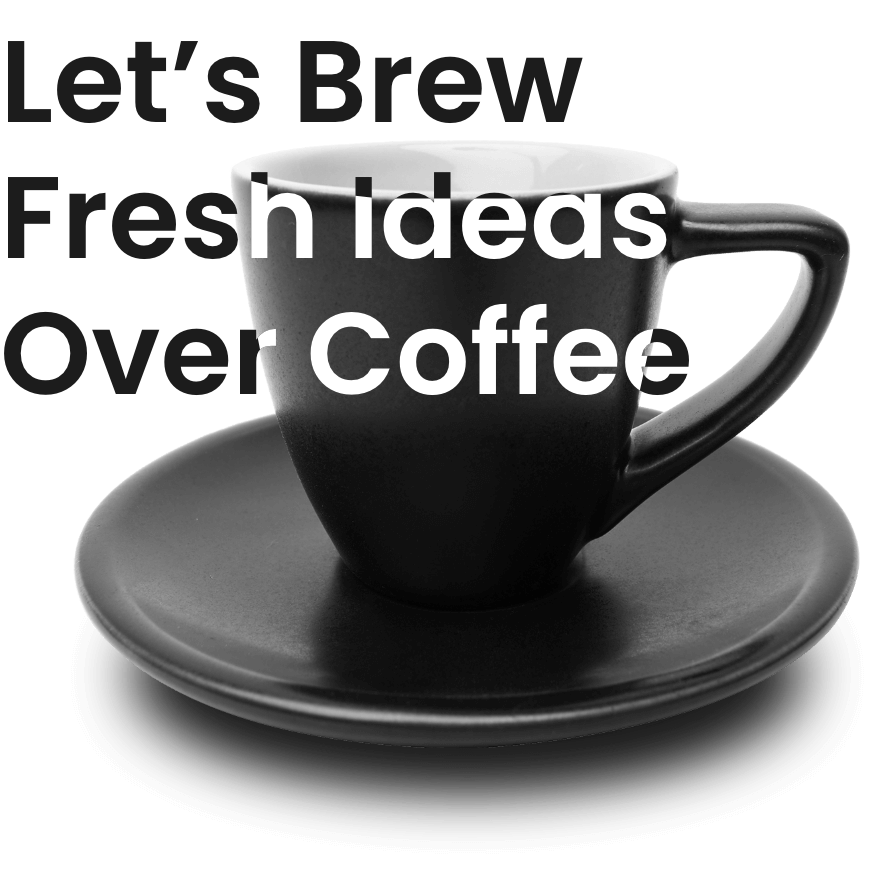
Spanning 8 cities worldwide and with partners in 100 more, we’re your local yet global agency.
Fancy a coffee, virtual or physical? It’s on us – let’s connect!