The role of sentiment analysis in understanding customer behavior in eCommerce
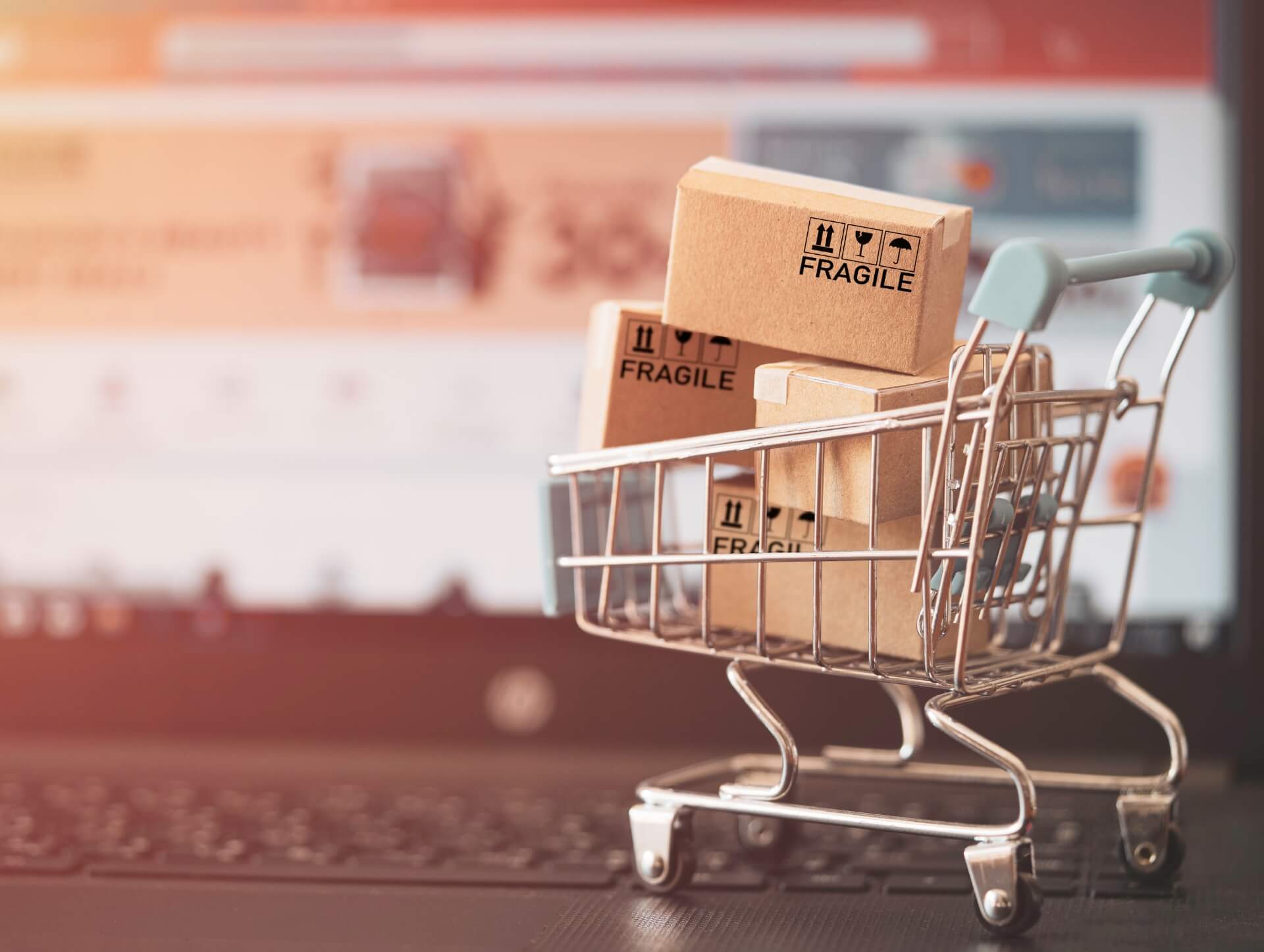
06/09/2023
The eCommerce industry has witnessed tremendous growth over the past decade, with more and more businesses transitioning to online platforms to reach a wider audience and maximize their revenue. With this surge in online shopping, understanding customer behavior has become crucial for businesses to stay competitive and provide a personalized shopping experience. One effective way to gain insights into customer behavior is through sentiment analysis.
What is Sentiment Analysis?
Sentiment analysis, also known as opinion mining, is a process of analyzing and understanding the emotions, opinions, and attitudes expressed in customer feedback, reviews, social media posts, and other forms of textual data. It involves using natural language processing (NLP) and machine learning techniques to classify the sentiment of the text as positive, negative, or neutral.
By analyzing customer sentiment, businesses can gain valuable insights into how customers perceive their products, services, and brand. This information can help them identify areas for improvement, make data-driven decisions, and enhance the overall customer experience.
The Importance of Sentiment Analysis in eCommerce
Understanding customer sentiment is crucial in the highly competitive eCommerce landscape. Here are some key reasons why sentiment analysis is important for eCommerce businesses:
1. Customer Feedback Analysis
Customer feedback is a goldmine of insights for businesses. By analyzing customer reviews and feedback, businesses can identify common pain points, product issues, and areas for improvement. Sentiment analysis allows businesses to categorize feedback as positive, negative, or neutral, making it easier to prioritize and address customer concerns.
For example, suppose an eCommerce store selling electronics receives multiple negative reviews about a specific product's durability. By analyzing the sentiment of these reviews, the store can identify the issue and take corrective actions such as improving the product quality or providing better customer support.
2. Personalized Recommendations
One of the key advantages of sentiment analysis in eCommerce is the ability to provide personalized product recommendations based on customer preferences. By analyzing the sentiment of past purchases, reviews, and social media interactions, businesses can understand the individual preferences and interests of customers.
For instance, if a customer has left positive reviews for several skincare products, an eCommerce store can use sentiment analysis to recommend similar products or offer personalized discounts on skincare items. This personalization not only enhances the shopping experience but also increases the chances of conversion and customer satisfaction.
3. Brand Monitoring and Reputation Management
In the age of social media, brand reputation can make or break a business. Sentiment analysis allows businesses to monitor and analyze customer sentiment on social media platforms and review sites. By tracking mentions of their brand and products, businesses can assess the overall sentiment associated with their brand.
If there is a sudden surge in negative sentiment, businesses can take immediate actions to address the issue, prevent potential damage to their reputation, and mitigate any negative impact on sales. On the other hand, if the sentiment is overwhelmingly positive, businesses can leverage it to enhance their marketing campaigns and build brand loyalty.
4. Competitor Analysis
Understanding customer sentiment not only helps businesses improve their own products and services but also provides insights into how their competitors are performing. By analyzing customer sentiment towards competitor brands and products, businesses can identify gaps in the market and gain a competitive advantage.
For example, if sentiment analysis reveals that customers are consistently mentioning a specific feature missing in competitor products, an eCommerce store can focus on incorporating that feature in their own products to cater to customer demands and gain a larger market share.
How Sentiment Analysis Works in eCommerce
Sentiment analysis in eCommerce involves several steps, including data collection, preprocessing, and sentiment classification. Here is a high-level overview of the process:
1. Data Collection
The first step in sentiment analysis is collecting relevant data. This can include customer reviews, feedback, social media posts, survey responses, and any other textual data that contains customer sentiment. Data can be collected manually or through automated methods such as web scraping or API integration.
2. Preprocessing
Once the data is collected, it needs to be preprocessed to remove any noise or irrelevant information. This involves tasks such as removing punctuation, converting text to lowercase, removing stop words, and stemming or lemmatizing words to their base form.
Preprocessing is important to ensure that the sentiment analysis model can accurately classify the sentiment without being influenced by irrelevant factors.
3. Sentiment Classification
The final step is sentiment classification, where machine learning algorithms are used to classify the sentiment of the text. This can be done using various techniques such as rule-based methods, supervised learning algorithms, or deep learning models.
Rule-based methods involve defining a set of rules or patterns to classify the sentiment. For example, if a review contains words like "great," "excellent," or "amazing," it can be classified as positive. While rule-based methods are simple to implement, they may not generalize well to different types of text.
Supervised learning algorithms require a labeled dataset where each text sample is annotated with its sentiment. The algorithm learns from this labeled data to classify new, unseen text samples. This approach can be more accurate but requires a substantial amount of labeled data for training.
Deep learning models, such as recurrent neural networks (RNNs) or transformers, have shown promising results in sentiment analysis. These models can learn the underlying patterns and dependencies in the text data, allowing for more accurate sentiment classification.
Challenges in Sentiment Analysis
While sentiment analysis can provide valuable insights, it is not without its challenges. Here are some common challenges faced in sentiment analysis:
1. Contextual Understanding
Sentiment analysis models often struggle with understanding the context and sarcasm in textual data. For example, a sentence like "The customer service was so good, it felt like a dream!" may be classified as positive by a model that fails to understand the sarcasm.
To overcome this challenge, advanced NLP techniques and models are being developed that can better capture the nuances of language and context.
2. Domain-specific Language
Sentiment analysis models trained on general datasets may not perform well when applied to domain-specific language. For example, if a model is trained on movie reviews, it may not accurately classify the sentiment of product reviews.
To address this challenge, domain-specific training data is required to fine-tune the sentiment analysis model for a particular industry or domain.
3. Handling Negations and Modifiers
Negations and modifiers can significantly affect the sentiment of a sentence. For example, the sentence "The product is not good" conveys a negative sentiment, even though the word "good" is present.
Sentiment analysis models need to be able to capture the impact of negations and modifiers on the overall sentiment of the text.
4. Data Imbalance
In sentiment analysis, datasets often suffer from class imbalance, where one sentiment class (usually neutral) is significantly more prevalent than the others. This can lead to biased models that perform poorly on minority classes.
Techniques such as oversampling, undersampling, or using weighted loss functions can help address the issue of data imbalance and improve the model's performance.
Conclusion
Sentiment analysis plays a crucial role in understanding customer behavior in eCommerce. By analyzing customer sentiment, businesses can gain deep insights into customer preferences, pain points, and brand perception. This information can be used to improve products and services, provide personalized recommendations, and enhance the overall customer experience.
While sentiment analysis has its challenges, advancements in NLP and machine learning techniques are continuously improving the accuracy and reliability of sentiment analysis models. As eCommerce continues to evolve, businesses that harness the power of sentiment analysis will be better equipped to meet customer expectations and drive success in the digital marketplace.
Contact us
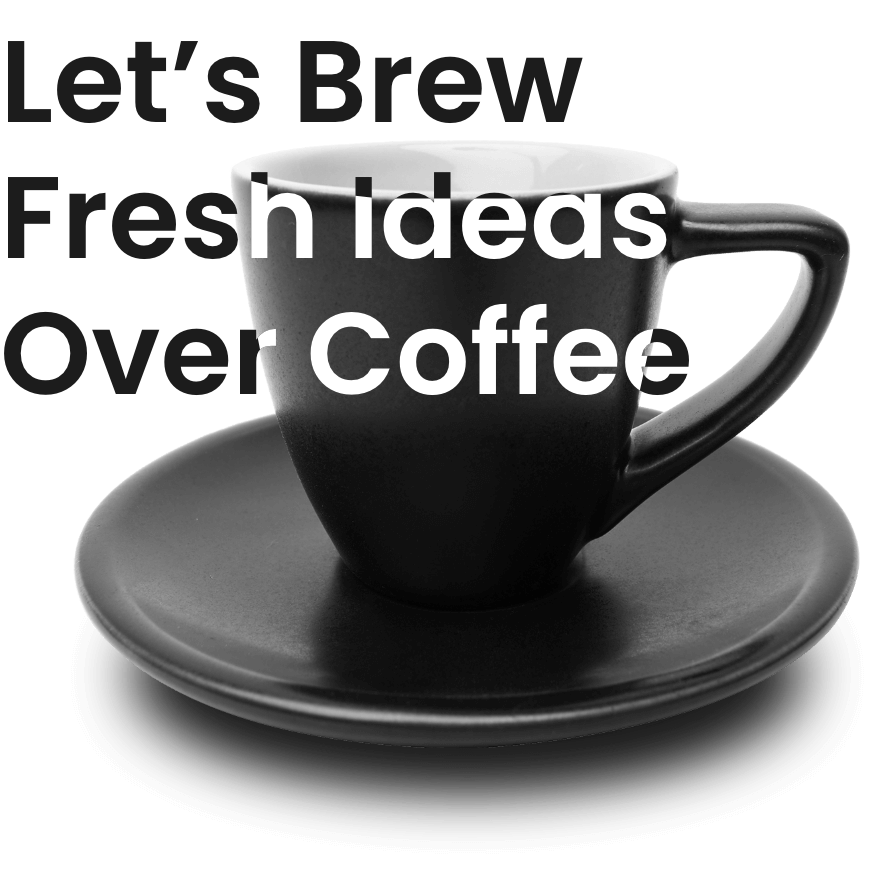
Spanning 8 cities worldwide and with partners in 100 more, we’re your local yet global agency.
Fancy a coffee, virtual or physical? It’s on us – let’s connect!