Best practices for data validation in MDM implementation
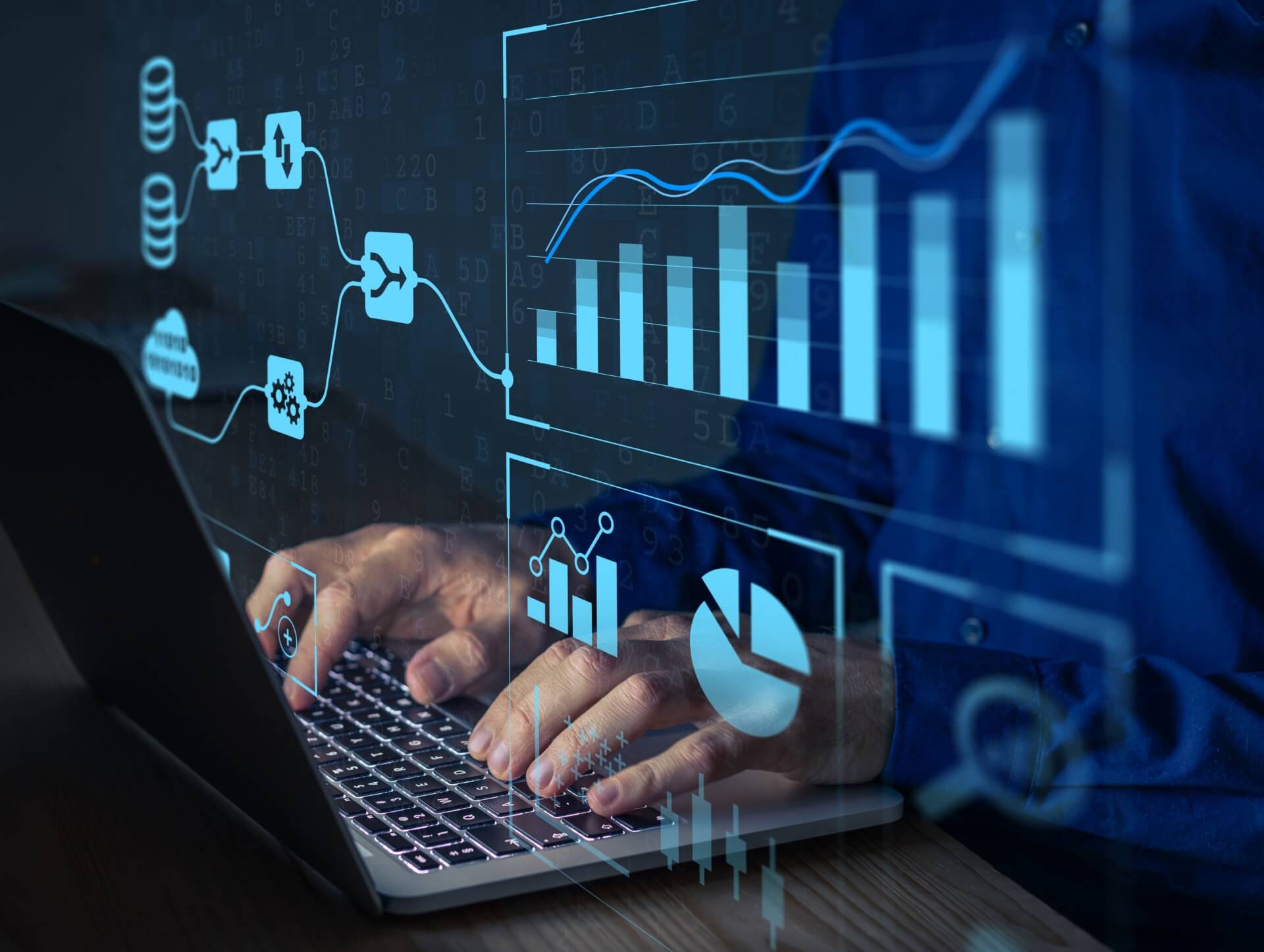
06/09/2023
Master Data Management (MDM) is a critical component of any organization's data management strategy. It involves the processes, tools, and technologies used to ensure the accuracy, consistency, and completeness of an organization's master data. Master data includes the core data entities that are essential for the operations of a business, such as customer data, product data, and employee data.
Implementing MDM can bring numerous benefits to an organization, including improved data quality, increased operational efficiency, enhanced decision-making, and better customer experience. However, to fully leverage these benefits, it is crucial to have robust data validation practices in place.
Why Data Validation is Important in MDM
Data validation is the process of ensuring that data is accurate, consistent, and reliable. In the context of MDM, data validation plays a vital role in maintaining the integrity of master data and ensuring that it meets the required quality standards. Here are some reasons why data validation is important in MDM:
- Ensures data accuracy: By validating the data before it is entered into the MDM system, organizations can minimize the risk of errors and inconsistencies in the master data.
- Improves data quality: Data validation helps identify and correct any data quality issues, such as missing or incorrect values, duplicate records, or inconsistent formats.
- Supports data governance: Data validation is an essential component of data governance in MDM. It helps enforce data standards, policies, and rules, ensuring that the master data is aligned with the overall data governance framework.
- Enhances decision-making: Accurate and reliable master data is crucial for making informed business decisions. Data validation ensures that the master data is trustworthy, enabling organizations to make better decisions based on accurate information.
- Improves customer experience: In MDM, customer master data plays a key role in providing personalized and consistent customer experiences. Data validation helps ensure that customer data is accurate and up-to-date, enabling organizations to deliver better customer service.
Best Practices for Data Validation in MDM Implementation
Implementing effective data validation practices in MDM requires a structured approach and adherence to best practices. Here are some key best practices to consider:
1. Define Data Quality Metrics
Start by defining the data quality metrics that are important for your organization. These metrics will serve as benchmarks to measure the quality of the master data. Examples of data quality metrics include data completeness, data accuracy, data consistency, and data timeliness.
2. Establish Data Validation Rules
Once the data quality metrics are defined, establish data validation rules that align with these metrics. Data validation rules define the criteria that data must meet to be considered valid. For example, a data validation rule for customer data might require that the customer's email address is in a valid email format.
3. Implement Data Validation Checks
Implement data validation checks at various stages of the MDM implementation process. These checks can be performed during data entry, data integration, and data migration processes. Data validation checks can be automated using MDM tools and technologies, which help identify and flag any data that does not meet the defined validation rules.
4. Conduct Data Cleansing
Data cleansing is the process of identifying and correcting any data quality issues in the master data. It involves activities such as removing duplicate records, standardizing data formats, and resolving inconsistencies. Data cleansing should be performed regularly to ensure the ongoing quality of the master data.
Challenges in Data Validation in MDM
While data validation is crucial in MDM, it can also pose certain challenges. Here are some common challenges organizations may face when implementing data validation in MDM:
- Data quality issues: Poor data quality can make data validation more challenging. Organizations may need to invest time and resources in data cleansing activities to address existing data quality issues.
- Data integration complexities: Data validation becomes more complex when integrating data from multiple sources, as each source may have different data formats and standards. Organizations need to define consistent validation rules and mappings to ensure data integrity.
- Data governance and ownership: Data validation requires clear data governance and ownership to define and enforce data standards and rules. Lack of proper data governance can result in inconsistent data validation practices.
- Scalability: As the volume of master data grows, data validation processes need to scale accordingly. Organizations should implement scalable data validation solutions to handle large volumes of data efficiently.
Conclusion
Data validation is a critical component of MDM implementation. By following best practices for data validation, organizations can ensure the accuracy, consistency, and reliability of their master data. This, in turn, leads to improved data quality, enhanced decision-making, and better customer experiences. While data validation may come with its own set of challenges, organizations can overcome them by investing in the right tools, technologies, and processes.
References
- "Master Data Management: Strategy, Organization, and Technologies for Data Governance" by Alex Berson and Larry Dubov
- "Master Data Management and Data Governance" by Alex Berson, Larry Dubov, and V. Lam
- "Master Data Management in Practice: Achieving True Customer MDM" by Dalton Cervo and Mark Allen
Contact us
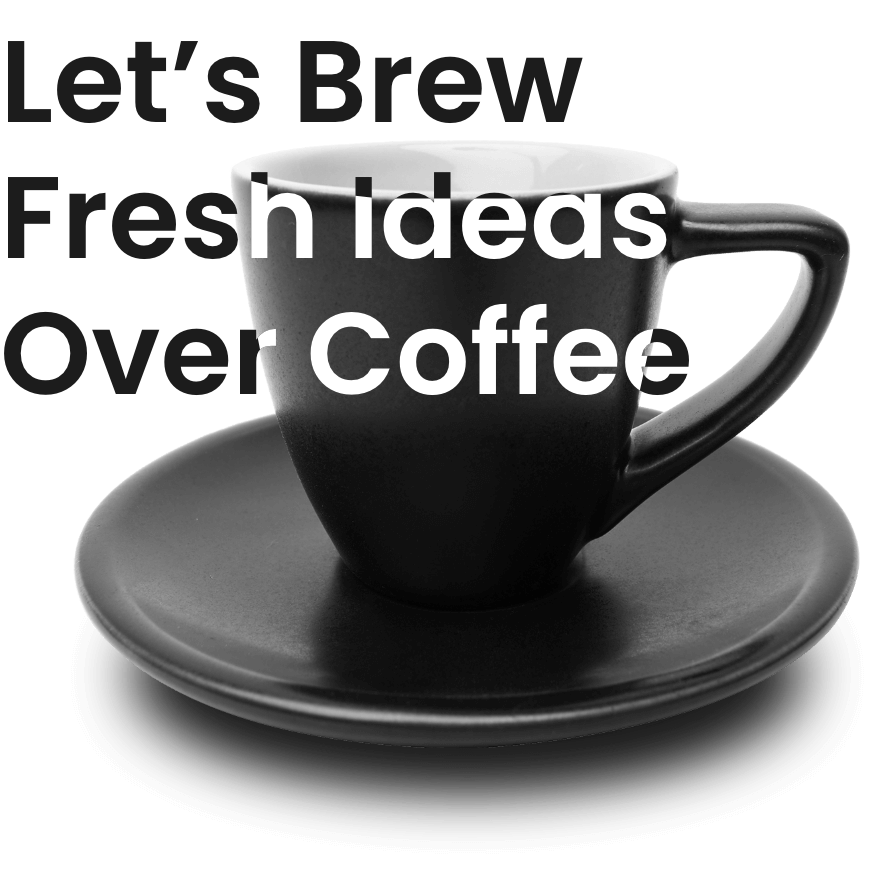
Spanning 8 cities worldwide and with partners in 100 more, we’re your local yet global agency.
Fancy a coffee, virtual or physical? It’s on us – let’s connect!