Best practices for implementing data governance in MDM
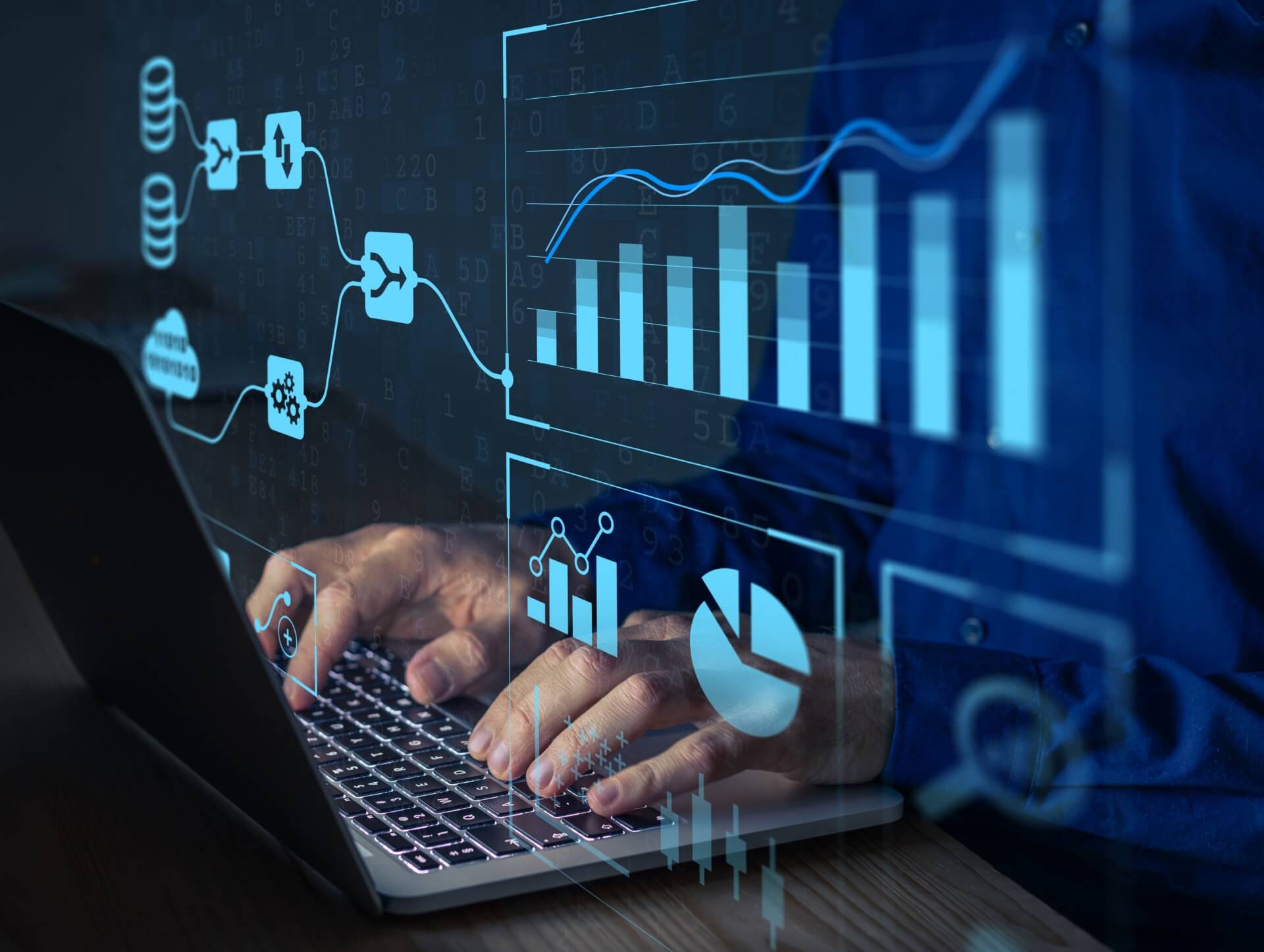
06/09/2023
Master Data Management (MDM) is a critical component of any organization's data strategy. It involves the management of master data, which is the core data that defines the business entities, such as customers, products, and suppliers, across the enterprise. Implementing data governance in MDM is essential to ensure the accuracy, consistency, and integrity of master data. This article explores the best practices for implementing data governance in MDM and highlights the benefits it brings to organizations.
Benefits of Data Governance in MDM
Data governance in MDM offers several benefits to organizations:
- Improved data quality: By implementing data governance in MDM, organizations can ensure that master data is accurate, consistent, and complete. This leads to improved data quality and reliability for decision-making.
- Enhanced data security: Data governance in MDM helps organizations establish data security policies and procedures to protect sensitive master data. This ensures compliance with data protection regulations and mitigates the risk of data breaches.
- Efficient data integration: With data governance in MDM, organizations can establish standardized data integration processes and rules. This simplifies the integration of master data from various sources and enables a holistic view of the business entities.
- Better decision-making: By ensuring the accuracy and consistency of master data, data governance in MDM enables organizations to make informed decisions based on reliable data. This leads to improved business outcomes and performance.
- Improved customer experience: Data governance in MDM allows organizations to maintain a single, unified view of customer data. This enables personalized and targeted interactions, leading to an enhanced customer experience.
Implementing Data Governance in MDM
Implementing data governance in MDM requires a systematic approach and adherence to best practices. Here are some key steps to consider:
1. Define Data Governance Policies
Start by defining data governance policies that outline the objectives, scope, and responsibilities of data governance in MDM. These policies should be aligned with the organization's overall data strategy and business goals. Clearly define the roles and responsibilities of data stewards and data custodians who will be responsible for enforcing these policies.
2. Establish Data Governance Framework
Establish a data governance framework that provides the structure and processes for implementing data governance in MDM. This framework should include the following components:
- Data governance council: Form a data governance council comprising key stakeholders from various business units. This council will be responsible for setting the strategic direction of data governance in MDM and resolving any conflicts or issues that arise.
- Data governance policies and procedures: Develop and document data governance policies and procedures that define how master data will be managed, maintained, and governed. These policies should address data quality management, data security, data integration, and data compliance.
- Data governance tools and technologies: Invest in suitable data governance tools and technologies that can support the implementation of data governance in MDM. These tools should facilitate data profiling, data cleansing, and data lineage tracking.
3. Establish Data Governance Processes
Establish data governance processes that ensure the effective management and governance of master data. These processes should cover the following areas:
- Data quality management: Define processes for assessing, monitoring, and improving the quality of master data. This includes data profiling, data cleansing, and data validation activities.
- Data security and compliance: Establish processes for ensuring the security and compliance of master data. This includes data access controls, data encryption, and data privacy measures.
- Data integration: Develop processes for integrating master data from various sources. This involves data mapping, data transformation, and data consolidation activities.
- Data lifecycle management: Implement processes for managing the lifecycle of master data, from creation to archival. This includes data versioning, data retention, and data purging activities.
4. Implement Data Governance Tools and Technologies
Invest in suitable data governance tools and technologies that can support the implementation of data governance in MDM. These tools should provide capabilities for:
- Data profiling: Use tools that can analyze the quality and integrity of master data, identifying any inconsistencies or anomalies.
- Data cleansing: Deploy tools that can cleanse and standardize master data, ensuring its accuracy and consistency.
- Data lineage tracking: Utilize tools that can track the origin and movement of master data, providing transparency and traceability.
- Data security and access controls: Implement tools that can enforce data security policies and access controls, protecting sensitive master data from unauthorized access.
- Data integration: Use tools that can facilitate the integration of master data from various sources, ensuring its consistency and completeness.
- Data governance dashboard: Deploy a data governance dashboard that provides visibility into the status and performance of data governance initiatives, enabling proactive monitoring and management.
Challenges in Implementing Data Governance in MDM
Implementing data governance in MDM can be challenging due to various factors. Here are some common challenges organizations may face:
- Lack of executive sponsorship: Without strong executive sponsorship, data governance initiatives may not receive the necessary support and resources. It is crucial to secure buy-in from senior leadership to drive successful implementation.
- Data quality issues: Inaccurate, inconsistent, and incomplete master data can hinder the effectiveness of data governance in MDM. It is essential to address data quality issues before implementing data governance processes.
- Data integration complexities: Integrating master data from disparate sources can be complex and time-consuming. Organizations need to establish robust data integration processes and ensure data compatibility across systems.
- Resistance to change: Implementing data governance in MDM often requires changes to existing processes and workflows. Resistance to change from employees can impede the adoption and success of data governance initiatives.
- Lack of data governance expertise: Organizations may lack the necessary expertise and skills to effectively implement data governance in MDM. It is important to invest in training and development to build a capable data governance team.
Case Studies: Successful MDM Implementations
Several organizations have successfully implemented data governance in MDM, leading to improved data management and business outcomes. Here are two case studies:
Case Study 1: Company XYZ
Company XYZ, a global retail organization, implemented data governance in MDM to address data quality issues and improve customer experience. They established a data governance council comprising representatives from various departments, including IT, marketing, and customer service. The council defined data governance policies and procedures, focusing on data quality management, data security, and data integration. They also implemented data governance tools and technologies, including data profiling and data cleansing tools. As a result, Company XYZ achieved a 20% improvement in data quality and saw an increase in customer satisfaction.
Case Study 2: Company ABC
Company ABC, a manufacturing company, implemented data governance in MDM to streamline its data integration processes and enhance decision-making. They established a dedicated data governance team comprising data stewards and data custodians. The team developed data governance processes, including data profiling, data security, and data integration. They also invested in data governance tools and technologies, such as data lineage tracking and data governance dashboards. Company ABC experienced a significant reduction in data integration time and improved the accuracy of data used for decision-making.
Conclusion
Data governance is a critical aspect of implementing MDM and ensuring the accuracy, consistency, and integrity of master data. By following the best practices for implementing data governance in MDM, organizations can unlock the full potential of their data assets and drive better business outcomes. It is crucial to invest in the right tools, establish robust processes, and secure executive sponsorship to achieve success in data governance and MDM.
Contact us
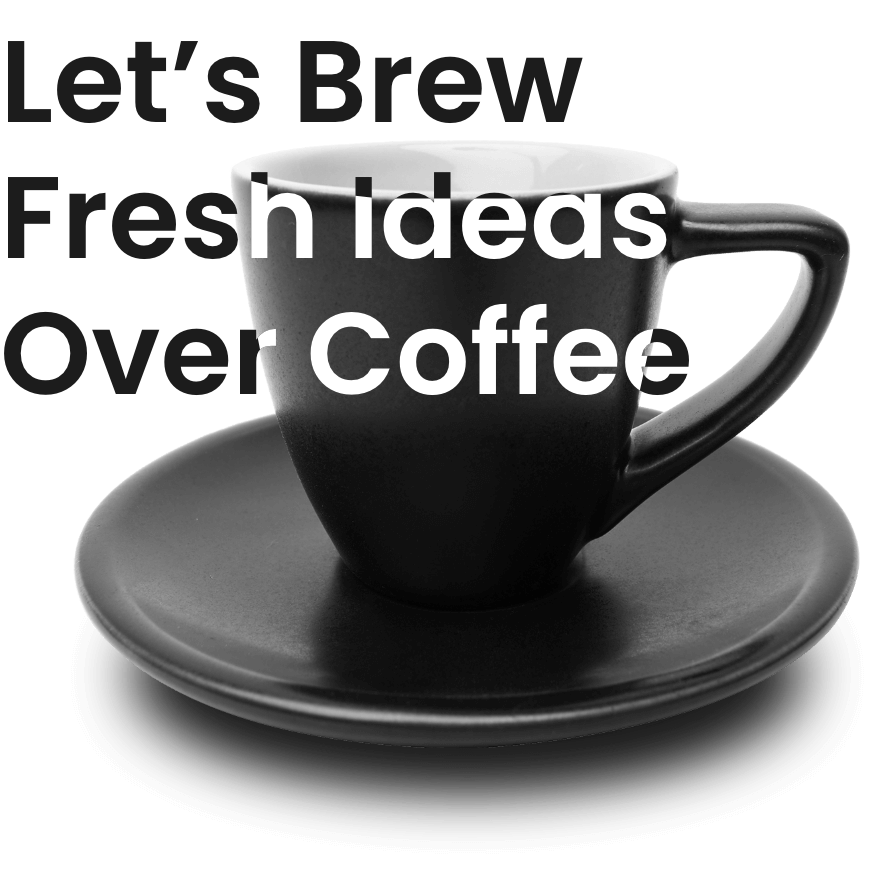
Spanning 8 cities worldwide and with partners in 100 more, we’re your local yet global agency.
Fancy a coffee, virtual or physical? It’s on us – let’s connect!