Best practices for implementing MDM for enterprise scalability
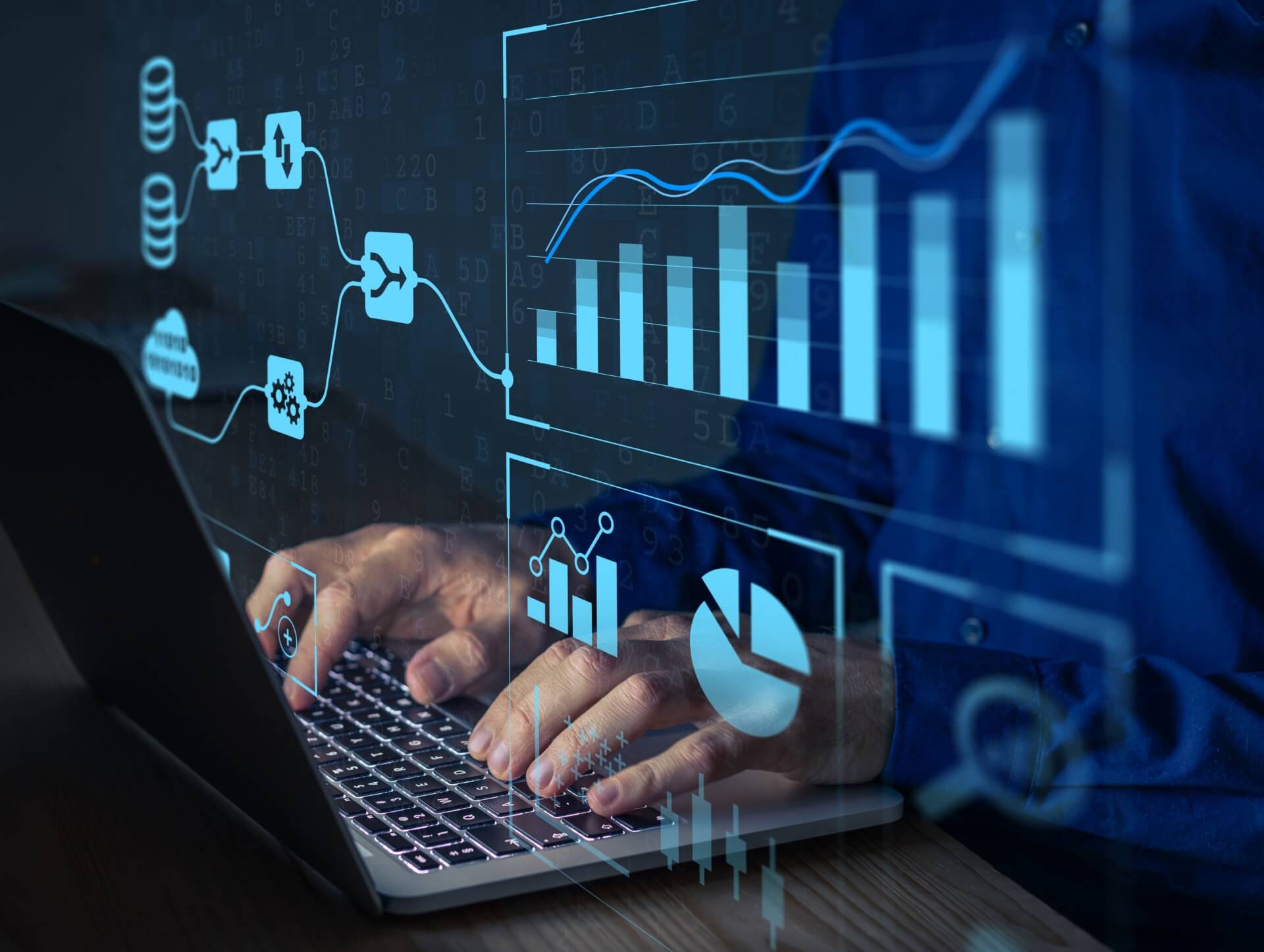
06/09/2023
Master Data Management (MDM) is a critical component of modern enterprises, enabling organizations to effectively manage and govern their master data assets. With the increasing volume, variety, and velocity of data, implementing MDM solutions has become a necessity for businesses seeking scalability and agility. In this article, we will explore the best practices for implementing MDM for enterprise scalability, covering key aspects such as data governance, data quality management, and the role of MDM in the overall data lifecycle.
MDM Strategy for Businesses
A well-defined MDM strategy is essential for businesses looking to implement MDM for enterprise scalability. The strategy should align with the organization's overall business goals and objectives, and should consider factors such as data governance, data integration, and data security. It is important to involve key stakeholders from different departments to ensure a holistic approach and buy-in from all relevant parties.
Benefits of Master Data Management
Implementing MDM offers several benefits for enterprises:
- Improved data quality: MDM ensures that master data is accurate, consistent, and up-to-date, leading to better decision-making and operational efficiency.
- Enhanced data governance: MDM provides a framework for establishing and enforcing data governance policies, ensuring compliance with regulations and standards.
- Increased data integration: MDM enables seamless integration of data from multiple sources, allowing organizations to gain a unified view of their data assets.
- Streamlined business processes: MDM helps streamline business processes by eliminating data silos and ensuring data consistency across the organization.
- Improved customer experience: MDM enables organizations to have a single, accurate view of their customers, leading to improved customer service and personalized experiences.
MDM Implementation Process
The implementation process for MDM involves several key steps:
- Define business requirements: Understand the specific business requirements and objectives that the MDM solution should address.
- Identify master data domains: Identify the key master data domains that need to be managed and governed by the MDM solution.
- Assess data quality: Evaluate the quality of existing master data and identify any data quality issues that need to be resolved.
- Select an MDM solution: Choose an MDM solution that aligns with the organization's requirements and goals.
- Design data model: Design a comprehensive data model that defines the structure and relationships of the master data.
- Implement data integration: Implement data integration processes to ensure seamless data flow between different systems and applications.
- Establish data governance: Define data governance policies and processes to ensure the ongoing management and governance of master data.
- Test and validate: Thoroughly test the MDM solution to ensure its functionality and accuracy.
- Deploy and monitor: Deploy the MDM solution and continuously monitor its performance and effectiveness.
Data Governance in MDM
Data governance plays a crucial role in the success of MDM implementations. It involves the establishment of policies, processes, and controls to ensure the proper management and governance of master data throughout its lifecycle. Key components of data governance in MDM include:
- Data stewardship: Assigning data stewards who are responsible for the ongoing management and governance of master data.
- Data quality management: Implementing data quality processes to ensure the accuracy, completeness, and consistency of master data.
- Data security: Ensuring the security of master data by implementing appropriate access controls and data protection measures.
- Data compliance: Ensuring compliance with relevant regulations and standards, such as GDPR or HIPAA, in the management of master data.
- Metadata management: Managing metadata to provide context and meaning to master data, enabling better data understanding and analysis.
Data Quality Management in MDM
Data quality management is a critical aspect of MDM implementations, as it ensures the accuracy, completeness, and consistency of master data. Key practices for data quality management in MDM include:
- Data profiling: Analyzing the quality of master data to identify any anomalies or issues that need to be addressed.
- Data cleansing: Removing or correcting any errors, duplicates, or inconsistencies in master data.
- Data standardization: Applying standardization rules to ensure consistent formats and values across the master data.
- Data validation: Implementing validation rules to ensure that master data meets predefined criteria and is fit for purpose.
- Data monitoring: Continuously monitoring the quality of master data to identify and resolve any issues in a timely manner.
Master Data Modeling
Master data modeling involves designing a comprehensive data model that defines the structure and relationships of the master data. Key considerations for master data modeling include:
- Identifying master data entities: Identify the key entities that need to be managed and governed by the MDM solution, such as customers, products, or employees.
- Defining attributes: Define the attributes or properties of each master data entity, such as name, address, or contact information.
- Establishing relationships: Establish relationships between master data entities to capture the dependencies and associations between them.
- Ensuring scalability: Design the data model in a way that allows for future scalability and flexibility, accommodating changing business needs and requirements.
MDM and Data Security
Data security is a critical consideration in MDM implementations, as master data often contains sensitive and confidential information. Key practices for ensuring data security in MDM include:
- Access controls: Implementing appropriate access controls to ensure that only authorized users have access to master data.
- Data encryption: Encrypting master data to protect it from unauthorized access or disclosure.
- Data masking: Masking or anonymizing sensitive data to ensure privacy and confidentiality.
- Auditing and monitoring: Implementing auditing and monitoring mechanisms to track and detect any unauthorized access or activity.
- Disaster recovery: Implementing robust disaster recovery measures to ensure the availability and integrity of master data in case of any unforeseen events.
MDM and Data Analytics
MDM and data analytics go hand in hand, as MDM provides the foundation for accurate and reliable data for analytics purposes. By implementing MDM, organizations can:
- Gain a single source of truth: MDM ensures that master data is accurate and consistent, providing a reliable foundation for data analytics.
- Enable better data analysis: With clean and reliable master data, organizations can perform more accurate and meaningful data analysis, leading to better insights and decision-making.
- Facilitate data-driven decision-making: MDM enables organizations to make data-driven decisions based on accurate and trustworthy data.
- Improve data visualization: By integrating MDM with data visualization tools, organizations can create meaningful and intuitive visualizations of their master data.
Challenges in MDM
Implementing MDM for enterprise scalability can pose several challenges for organizations. Some of the common challenges include:
- Data complexity: Dealing with the increasing volume, variety, and velocity of data can be challenging, requiring robust data management and integration capabilities.
- Data quality issues: Ensuring data quality and resolving data quality issues can be time-consuming and resource-intensive.
- Organizational alignment: Gaining alignment and buy-in from different departments and stakeholders can be challenging, as MDM often requires changes to existing processes and workflows.
- Legacy systems integration: Integrating MDM with legacy systems and applications can be complex, requiring careful planning and execution.
- Data governance and ownership: Establishing clear data governance policies and defining data ownership can be challenging, especially in organizations with complex structures and distributed responsibilities.
MDM Case Studies
Several organizations have successfully implemented MDM for enterprise scalability. Here are a few case studies:
- Company A: Company A, a global manufacturing company, implemented an MDM solution to streamline its product data management processes. By centralizing and governing its product data, the company was able to improve data quality, reduce time-to-market, and enhance its overall product lifecycle management.
- Company B: Company B, a financial services firm, implemented an MDM solution to manage its customer data across multiple channels and systems. By having a single, accurate view of its customers, the company was able to improve customer service, personalize marketing campaigns, and increase customer satisfaction.
- Company C: Company C, a healthcare organization, implemented an MDM solution to manage its patient data across different departments and systems. By ensuring the accuracy and consistency of patient data, the organization was able to improve clinical decision-making, enhance patient care, and comply with regulatory requirements.
Conclusion
Implementing MDM for enterprise scalability is crucial for organizations looking to effectively manage and govern their master data assets. By following best practices such as defining a clear MDM strategy, implementing robust data governance and data quality management processes, and considering the role of MDM in the overall data lifecycle, organizations can maximize the benefits of MDM and achieve scalability and agility in their data management practices.
Contact us
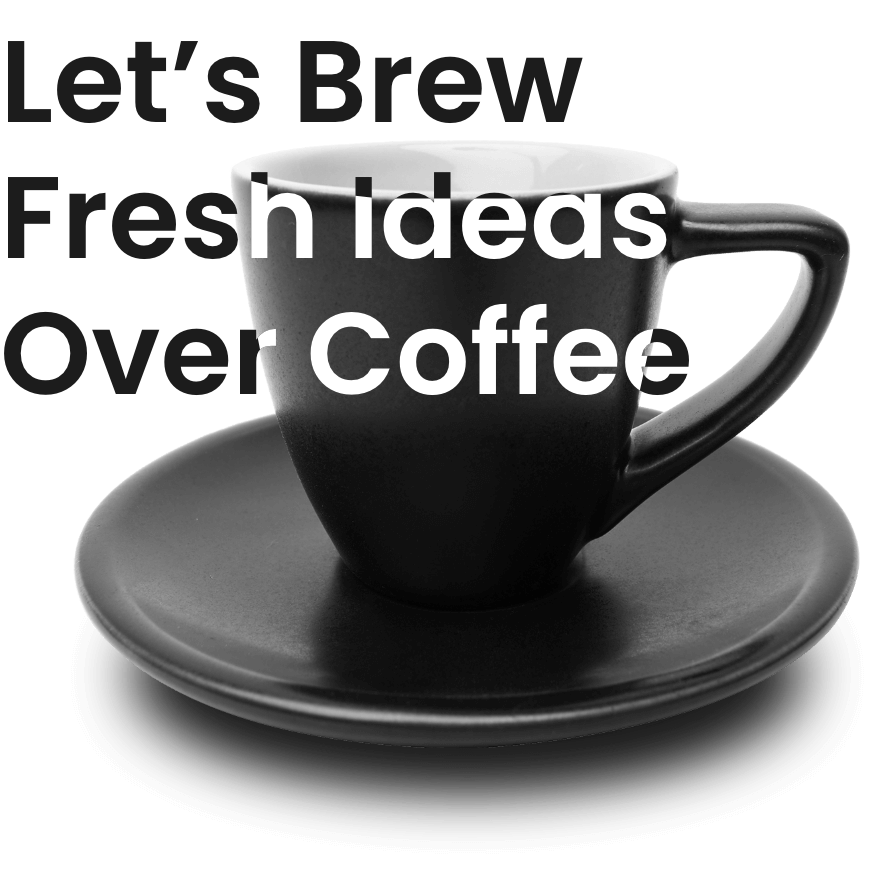
Spanning 8 cities worldwide and with partners in 100 more, we’re your local yet global agency.
Fancy a coffee, virtual or physical? It’s on us – let’s connect!