Case study: Successful implementation of MDM for enterprise scalability
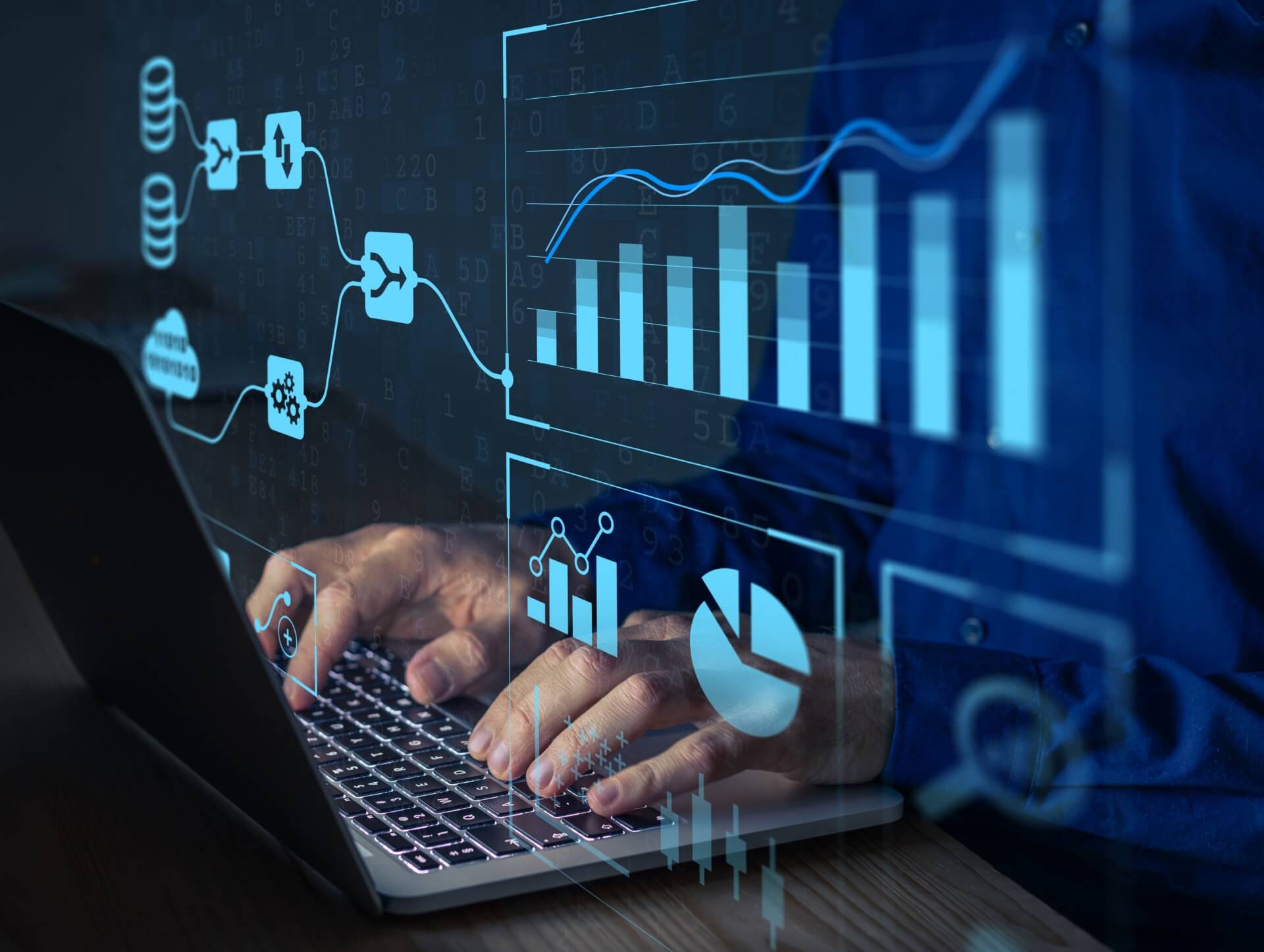
06/09/2023
Master Data Management (MDM) is a comprehensive approach to managing and harmonizing the critical data assets of an organization. It involves creating a single, accurate, and consistent version of master data across different systems and applications. MDM enables businesses to improve data quality, enhance operational efficiency, and make better-informed decisions.
In this case study, we will explore the successful implementation of MDM for enterprise scalability. We will discuss the benefits of MDM, MDM strategies, data governance, MDM tools and technologies, challenges, and more.
The Benefits of Master Data Management
Implementing a robust MDM solution offers numerous benefits for organizations:
- Improved Data Quality: MDM ensures that data is accurate, consistent, and up-to-date. It eliminates data duplication and inconsistencies, leading to better data quality.
- Enhanced Operational Efficiency: MDM streamlines data processes and workflows, reducing manual effort and improving overall operational efficiency.
- Better Decision Making: With reliable and trustworthy data, organizations can make better-informed decisions, leading to improved business outcomes.
- Regulatory Compliance: MDM helps organizations comply with data privacy regulations and ensures data governance and security throughout the data lifecycle.
- Increased Customer Satisfaction: By having a holistic view of customer data, organizations can provide personalized and targeted experiences, leading to higher customer satisfaction.
- Cost Savings: MDM eliminates data redundancy and reduces data management costs by centralizing data and eliminating data silos.
MDM Strategies for Successful Implementation
Implementing MDM requires a well-defined strategy to ensure successful adoption and scalability:
- Define Clear Objectives: Clearly define the goals and objectives of the MDM initiative. Identify the critical data domains and determine the data governance requirements.
- Engage Stakeholders: Involve key stakeholders from different departments and business units to ensure alignment and buy-in. Establish a governance board to oversee the MDM implementation.
- Assess Data Quality: Conduct a thorough assessment of existing data quality to identify data issues and gaps. Develop data cleansing and enrichment strategies to improve data quality.
- Select the Right MDM Platform: Choose an MDM platform that aligns with your organization's requirements and supports your data management goals. Consider factors such as scalability, data integration capabilities, and ease of use.
- Design Master Data Model: Develop a comprehensive master data model that captures all the critical data entities, attributes, and relationships. Define data hierarchies and taxonomies for effective data management.
- Implement Data Governance: Establish data governance policies, processes, and workflows to ensure data quality, security, and compliance. Define roles and responsibilities for data stewardship and data ownership.
- Integrate with Existing Systems: Integrate the MDM platform with existing systems and applications to ensure seamless data flow and synchronization. Implement data integration best practices to avoid data inconsistencies.
- Train and Educate Users: Provide comprehensive training and education to users on the MDM platform and data governance processes. Foster a culture of data stewardship and data ownership throughout the organization.
- Monitor and Measure Success: Continuously monitor and measure the success of the MDM implementation. Track key performance indicators (KPIs) such as data quality, data accuracy, and user adoption.
- Iterate and Improve: Regularly review and refine the MDM strategy based on feedback and changing business requirements. Identify areas for improvement and implement enhancements to maximize the value of MDM.
Data Governance in MDM
Data governance is a critical component of MDM that ensures the availability, integrity, and security of data throughout its lifecycle. It involves defining data policies, processes, and standards to govern data quality, data security, and data compliance. Effective data governance in MDM helps organizations:
- Establish Data Ownership: Define clear data ownership roles and responsibilities to ensure accountability and stewardship of master data.
- Ensure Data Quality: Implement data quality management processes to cleanse, enrich, and validate master data. Define data quality metrics and KPIs to measure and monitor data quality.
- Enforce Data Security: Implement data security measures to protect sensitive master data from unauthorized access and data breaches. Define data access controls and encryption policies.
- Comply with Regulations: Ensure compliance with data privacy regulations such as GDPR and CCPA. Implement processes to handle data subject requests and consent management.
- Enable Data Integration: Establish data integration processes and standards to ensure seamless data flow across systems and applications. Implement data integration tools and technologies for efficient data synchronization.
- Facilitate Change Management: Define change management processes to manage changes to master data. Implement version control and change tracking mechanisms to maintain data integrity.
MDM Tools and Technologies
There are various MDM tools and technologies available in the market to support the implementation of an MDM strategy:
- Commercial MDM Platforms: Commercial MDM platforms provide comprehensive features and functionalities for managing master data. They offer data modeling, data integration, data quality, and data governance capabilities.
- Open Source MDM Solutions: Open source MDM solutions offer flexibility and cost-effectiveness. They provide basic MDM capabilities and can be customized and extended as per specific requirements.
- Cloud-based MDM Platforms: Cloud-based MDM platforms offer scalability, agility, and cost savings. They enable organizations to leverage the benefits of the cloud for MDM implementation.
- Data Integration Tools: Data integration tools such as ETL (Extract, Transform, Load) tools and API integration platforms are essential for integrating master data with other systems and applications.
- Data Quality Tools: Data quality tools help identify and resolve data quality issues. They provide data cleansing, data enrichment, and data validation capabilities.
- Data Governance Tools: Data governance tools assist in establishing data governance policies and workflows. They provide functionalities for data stewardship, metadata management, and data compliance.
Challenges in MDM
Implementing MDM can pose several challenges for organizations:
- Data Complexity: Organizations often have complex data landscapes with multiple systems and applications. Integrating and harmonizing data from disparate sources can be challenging.
- Data Quality Issues: Poor data quality is a common challenge in MDM. Data duplication, inconsistencies, and inaccuracies can hinder the effectiveness of MDM initiatives.
- Data Governance Adoption: Establishing a data governance framework and driving adoption across the organization can be challenging. It requires change management and cultural transformation.
- Data Integration Complexity: Integrating master data with existing systems and applications can be complex. Data integration challenges can arise due to data formats, data mappings, and data synchronization.
- Organizational Silos: Organizational silos and departmental barriers can hinder the success of MDM initiatives. Collaboration and communication across departments are essential for effective MDM.
- Master Data Ownership: Defining clear data ownership roles and responsibilities can be challenging. Lack of data ownership can result in data quality issues and data governance gaps.
- Legacy Systems: Legacy systems and outdated technology infrastructure can pose challenges for MDM implementation. Legacy systems may lack the necessary capabilities for seamless data integration.
Case Study: Successful Implementation of MDM for Enterprise Scalability
To illustrate the successful implementation of MDM for enterprise scalability, let's consider the case of a global retail organization.
Challenge: The organization had multiple systems and applications across different regions, resulting in data duplication and inconsistencies. They faced challenges in obtaining a single, accurate view of customer data, which impacted their marketing campaigns, customer service, and overall customer experience.
Solution: The organization implemented a cloud-based MDM platform to centralize and manage customer master data. They followed a phased approach for implementation:
- Assessment: The organization conducted a comprehensive assessment of their existing data quality and data governance practices. They identified data issues and gaps, and defined data quality metrics and data governance policies.
- Platform Selection: After evaluating different MDM platforms, the organization selected a cloud-based MDM platform that offered scalability, data integration capabilities, and ease of use.
- Data Integration: They integrated the MDM platform with their existing systems and applications using data integration tools. They mapped data fields and established data synchronization processes.
- Data Cleansing and Enrichment: The organization implemented data cleansing and enrichment processes to improve the quality of customer data. They removed duplicates, standardized data formats, and enriched customer profiles with additional information.
- Data Governance: They established a data governance framework with defined roles, responsibilities, and workflows. Data stewards were assigned to ensure data quality and compliance with data governance policies.
- User Training: The organization provided comprehensive training to users on the MDM platform and data governance processes. They emphasized the importance of data stewardship and data ownership.
- Continuous Improvement: The organization continuously monitored data quality and user adoption. They collected feedback from users and made iterative improvements to the MDM strategy.
Results: The successful implementation of MDM had significant benefits for the organization:
- Single View of Customer: The organization achieved a single, accurate view of customer data across regions and systems. This enabled personalized marketing campaigns, improved customer service, and enhanced customer experience.
- Data Quality Improvement: Data quality significantly improved, with reduced data duplication and inconsistencies. This led to better decision-making and improved operational efficiency.
- Cost Savings: The organization achieved cost savings by eliminating data redundancy and streamlining data processes. They were able to retire legacy systems and reduce data management costs.
- Compliance and Security: The organization ensured compliance with data privacy regulations and implemented robust data security measures. They established data governance practices to maintain data integrity and security.
- Scalability: The cloud-based MDM platform offered scalability, enabling the organization to handle increasing volumes of customer data. They were able to expand their MDM initiative to include other data domains.
Conclusion
Implementing MDM for enterprise scalability requires a well-defined strategy, effective data governance, and the right tools and technologies. Organizations can achieve numerous benefits by implementing an MDM solution, including improved data quality, enhanced operational efficiency, better decision-making, and increased customer satisfaction. However, organizations should be aware of the challenges associated with MDM and take a phased approach to implementation. By following best practices, organizations can successfully implement MDM and realize its full potential for enterprise scalability.
Contact us
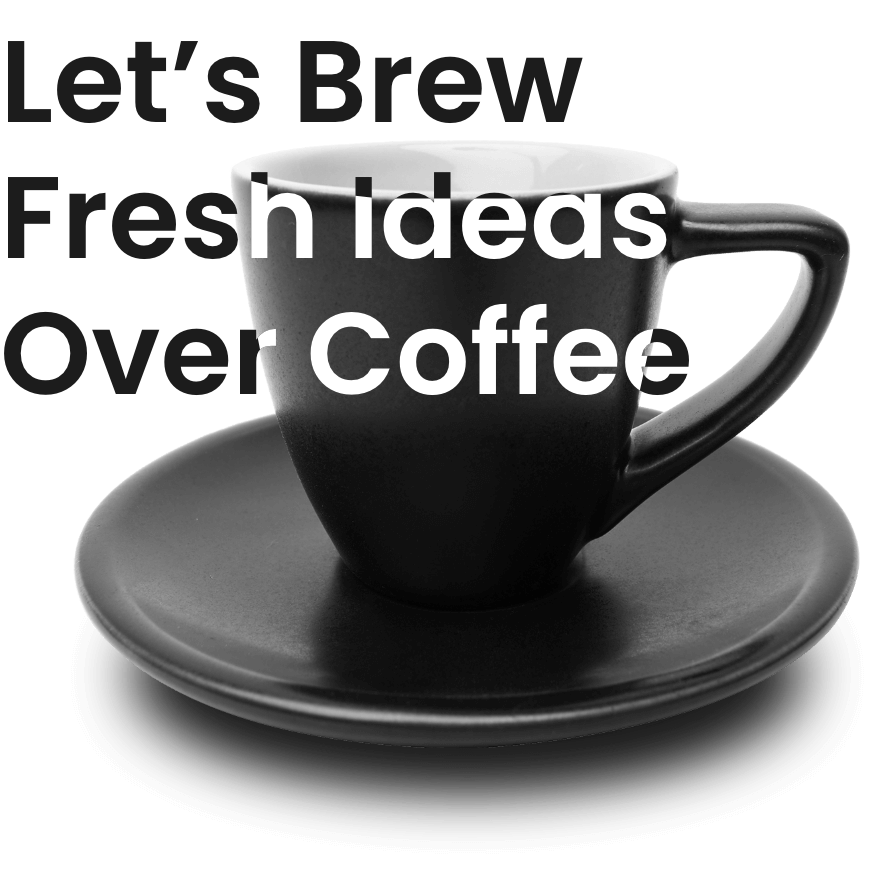
Spanning 8 cities worldwide and with partners in 100 more, we’re your local yet global agency.
Fancy a coffee, virtual or physical? It’s on us – let’s connect!