Data integration and data cleansing in MDM
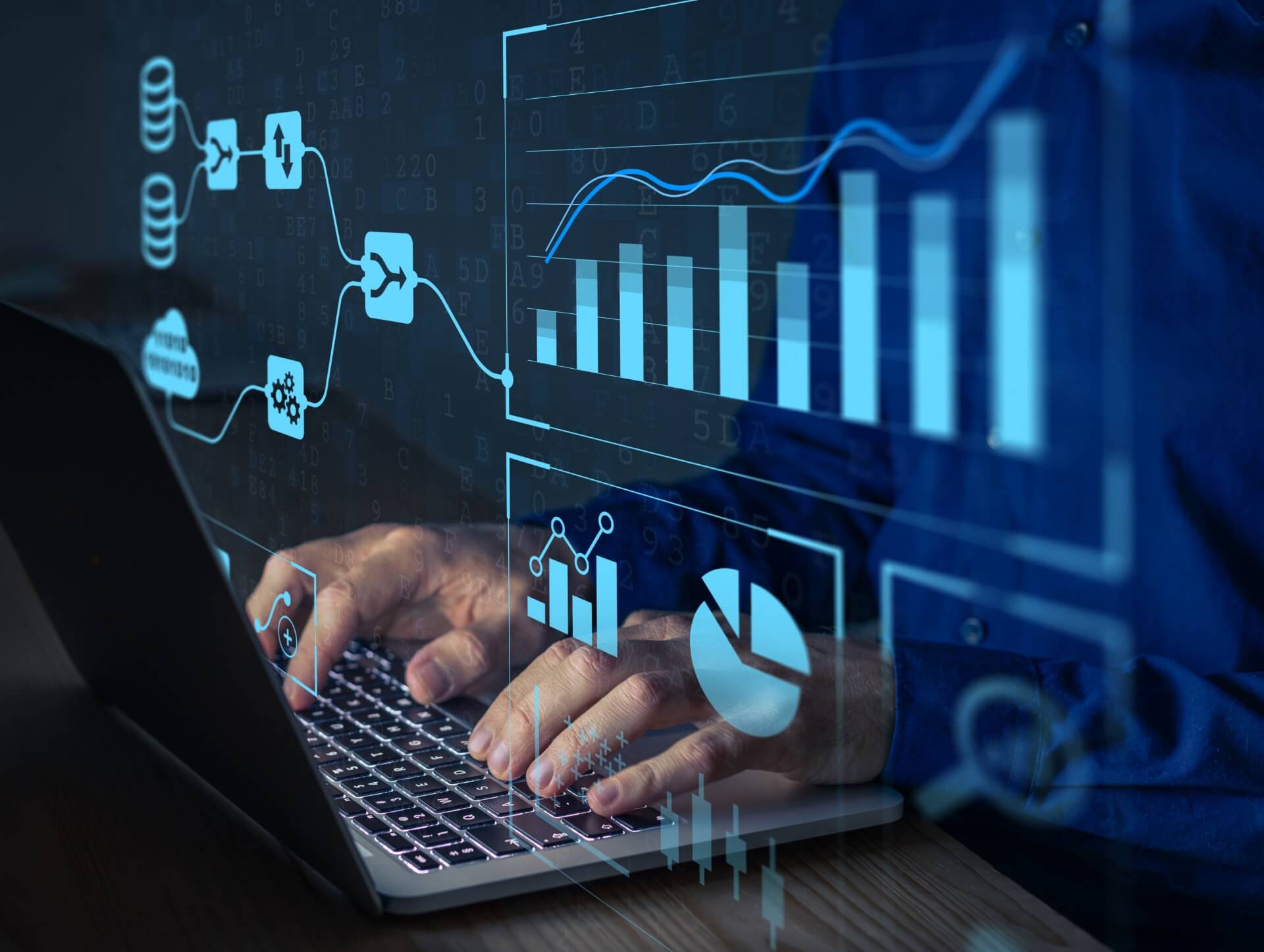
06/09/2023
Master Data Management (MDM) is a comprehensive approach to managing and integrating an organization's critical data assets. It involves creating and maintaining a single, consistent, and accurate view of master data across multiple systems and applications. MDM solutions are designed to provide a centralized platform for managing master data, ensuring data quality, and supporting data governance processes.
The Importance of Data Integration in MDM
Data integration is a crucial component of MDM, as it enables the consolidation and synchronization of master data from various sources into a single, unified view. It involves the extraction, transformation, and loading (ETL) of data from disparate systems, databases, and applications, and the mapping and transformation of the data to fit the target MDM model.
Data integration in MDM allows organizations to:
- Eliminate data silos and achieve a holistic view of master data
- Reduce data redundancy and improve data consistency
- Enhance data quality and accuracy
- Streamline business processes and decision-making
- Enable data-driven insights and analytics
Data Cleansing in MDM
Data cleansing, also known as data scrubbing or data cleansing, is the process of identifying and correcting or removing errors, inconsistencies, and inaccuracies in master data. It involves various techniques, such as data profiling, data validation, data standardization, and data enrichment, to ensure the integrity and quality of master data.
Data cleansing in MDM helps organizations:
- Improve data accuracy and reliability
- Enhance data completeness and consistency
- Ensure compliance with data governance policies and regulations
- Reduce the risk of errors and data-driven business failures
- Enable effective data analysis and reporting
Best Practices for Data Integration and Data Cleansing in MDM
Effective data integration and data cleansing in MDM require a systematic and well-defined approach. Here are some best practices to consider:
1. Define Clear Data Integration and Data Cleansing Objectives
Before embarking on data integration and data cleansing initiatives, it is essential to define clear objectives and goals. Determine what data needs to be integrated and cleansed, and what outcomes are expected from these processes. This will help guide the implementation and ensure the desired results are achieved.
2. Establish Data Governance Framework
Data governance plays a critical role in MDM, as it provides the structure and processes for managing and controlling data assets. Establish a data governance framework that defines data ownership, accountability, and stewardship. This will help ensure data integration and cleansing activities are aligned with organizational policies and standards.
3. Perform Data Profiling and Analysis
Before integrating and cleansing data, it is essential to understand the quality and characteristics of the data. Perform data profiling and analysis to identify data anomalies, inconsistencies, and errors. This will help prioritize data cleansing efforts and ensure data integration is based on accurate and reliable data.
4. Implement Data Standardization and Validation
Data standardization involves defining and enforcing consistent data formats, structures, and values across the organization. Implement data standardization rules and validation mechanisms to ensure data consistency and accuracy during integration and cleansing processes. This will help improve data quality and facilitate data mapping and transformation.
5. Automate Data Integration and Cleansing Processes
To streamline data integration and cleansing, consider implementing automated tools and technologies. Automation can help reduce manual errors, improve efficiency, and accelerate the integration and cleansing processes. Choose MDM solutions that offer built-in data integration and cleansing capabilities or integrate with third-party tools.
6. Establish Data Quality Metrics and Monitoring
Define data quality metrics and establish monitoring mechanisms to assess the effectiveness of data integration and cleansing activities. Continuously monitor data quality and track key performance indicators (KPIs) to identify areas of improvement and ensure ongoing data quality maintenance.
7. Involve Data Stewards and Subject Matter Experts
Data integration and cleansing activities should involve data stewards and subject matter experts who have a deep understanding of the data and its business context. Collaborate with these stakeholders to validate data mappings, resolve data conflicts, and ensure the accuracy and completeness of integrated and cleansed data.
Conclusion
Data integration and data cleansing are critical components of a successful MDM strategy. By integrating and cleansing data from various sources, organizations can achieve a single, accurate, and complete view of master data. This enables improved data quality, streamlined business processes, and data-driven decision-making. By following best practices, organizations can ensure the success of their data integration and cleansing initiatives and maximize the value of their MDM investments.
Contact us
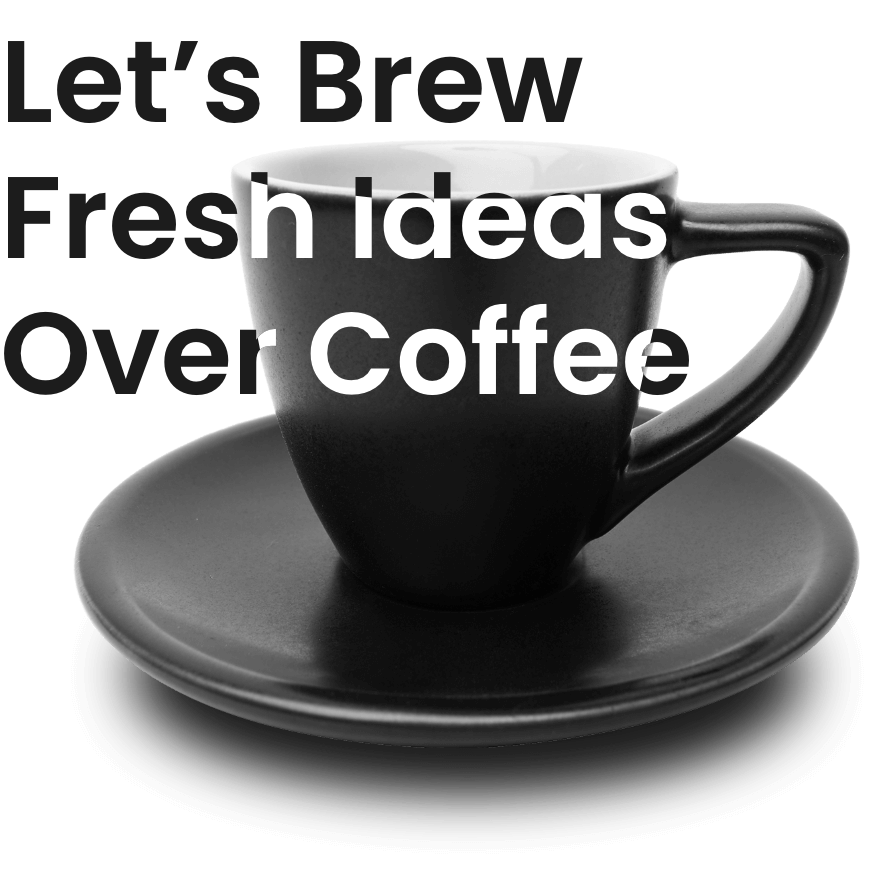
Spanning 8 cities worldwide and with partners in 100 more, we’re your local yet global agency.
Fancy a coffee, virtual or physical? It’s on us – let’s connect!