Data integration and data mapping in MDM
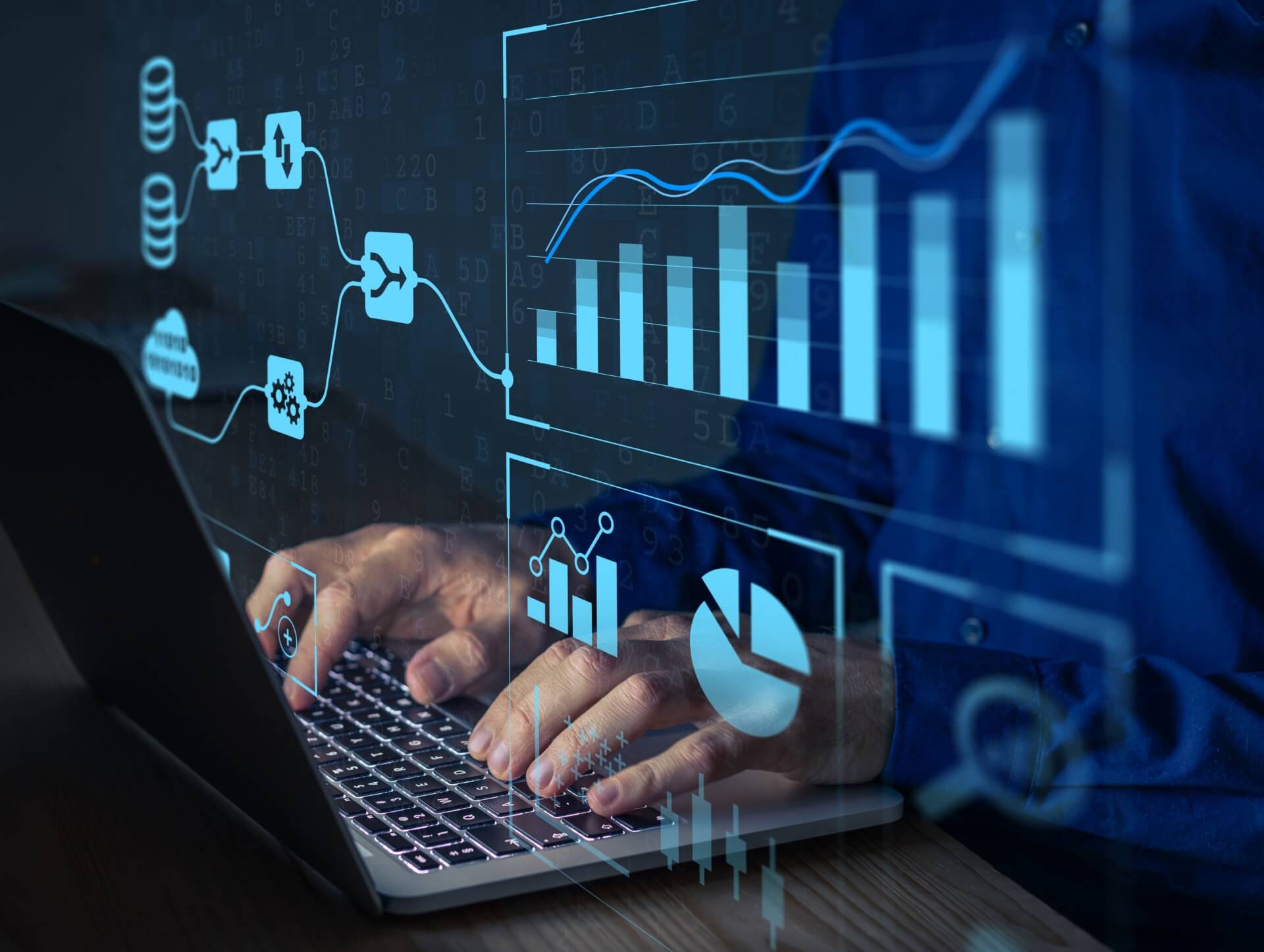
06/09/2023
Data integration and data mapping are critical components of Master Data Management (MDM) that ensure effective management and utilization of data within an organization. MDM focuses on creating and maintaining a single, trusted, and accurate version of master data, such as customer, product, and supplier data, across different systems and applications. This article explores the importance of data integration and data mapping in MDM, the challenges involved, and best practices for successful implementation.
Data Integration in MDM
Data integration refers to the process of combining data from various sources and systems into a unified view. In the context of MDM, data integration involves consolidating and harmonizing data from disparate systems and applications to create a single, consistent, and accurate version of master data. This ensures that all business functions and processes have access to reliable and up-to-date information.
There are different approaches to data integration in MDM, including:
1. Batch Integration
In batch integration, data is extracted from source systems at regular intervals and loaded into the MDM system for processing. This approach is suitable for scenarios where real-time data synchronization is not required.
2. Real-time Integration
In real-time integration, data is synchronized between source systems and the MDM system in real-time or near-real-time. This approach ensures that changes made in source systems are immediately reflected in the MDM system, enabling up-to-date and accurate master data.
3. Hybrid Integration
A hybrid integration approach combines batch and real-time integration methods. It allows for both scheduled batch updates and real-time synchronization, depending on the specific requirements of the organization.
Regardless of the integration approach chosen, data integration in MDM involves several key steps:
Data Extraction
Data is extracted from source systems, which can include databases, applications, and files. The extraction process involves identifying the relevant data elements and transforming them into a standardized format for further processing.
Data Transformation
Extracted data is transformed into a common format that adheres to the data model of the MDM system. This step includes data cleansing, normalization, and enrichment to ensure consistency and accuracy.
Data Loading
The transformed data is then loaded into the MDM system, where it undergoes validation, matching, and merging with existing master data. This step ensures that only high-quality and consistent data is stored in the MDM system.
Data Mapping in MDM
Data mapping is the process of establishing relationships and mappings between data elements in different systems. In the context of MDM, data mapping is crucial for aligning and reconciling data from various sources to create a unified and comprehensive view of master data.
Key aspects of data mapping in MDM include:
1. Field Mapping
Field mapping involves mapping the data fields or attributes of source systems to the corresponding fields in the MDM system. This ensures that data from different sources can be integrated and consolidated accurately.
2. Data Transformation
Data transformation is often required during the data mapping process to convert data from one format to another or to apply business rules and logic. This step ensures that data from different sources is standardized and consistent.
3. Data Matching
Data matching is an essential part of data mapping in MDM. It involves comparing and identifying similar or duplicate records across different systems. This helps in identifying the best record or creating a composite record with the most accurate and complete information.
4. Data Linkages
Data linkages establish relationships between different data elements within the MDM system. This includes linking customer data with product data, supplier data, and other related data entities. These linkages enable a holistic view of master data and support data-driven decision making.
Benefits of Data Integration and Data Mapping in MDM
Implementing robust data integration and data mapping processes in MDM can yield several benefits for organizations:
1. Improved Data Quality
By integrating and mapping data from various sources, organizations can ensure that the master data stored in the MDM system is accurate, consistent, and complete. This improves the overall quality and reliability of data used for business operations, analysis, and decision making.
2. Enhanced Data Governance
Data integration and data mapping are key components of effective data governance in MDM. They enable organizations to establish and enforce data standards, policies, and rules across different systems and applications. This ensures compliance with regulatory requirements and improves data security and privacy.
3. Increased Operational Efficiency
By centralizing and standardizing master data, organizations can streamline business processes and eliminate duplicate or redundant data. This leads to improved operational efficiency, reduced errors, and faster data access and retrieval.
4. Better Customer Experience
Accurate and up-to-date customer data is essential for delivering personalized and seamless customer experiences. Through data integration and mapping, organizations can create a 360-degree view of customer data, enabling targeted marketing, personalized offers, and improved customer service.
5. Data-driven Decision Making
Integrated and mapped master data provides a foundation for data analytics and business intelligence. Organizations can leverage this data to gain valuable insights, identify trends, and make informed decisions based on reliable and comprehensive information.
Challenges in Data Integration and Data Mapping
While data integration and data mapping are crucial for successful MDM, organizations often face several challenges during implementation:
1. Data Complexity
Organizations deal with a vast amount of data from various sources, each with its own structure and format. Mapping and integrating this complex data landscape can be challenging, requiring careful planning, data profiling, and data modeling.
2. Data Quality Management
Poor data quality can hinder the effectiveness of data integration and mapping processes. Organizations must invest in data cleansing, validation, and enrichment to ensure that only high-quality data is integrated into the MDM system.
3. Data Governance
Data governance plays a critical role in data integration and mapping. Establishing data governance policies, defining data ownership, and ensuring compliance with regulations can be complex and require cross-functional collaboration.
4. Legacy Systems
Integrating data from legacy systems that may have outdated technology and data structures can pose challenges. Organizations must invest in legacy system modernization or find alternative integration methods to ensure seamless data flow.
Best Practices for Effective Data Integration and Data Mapping in MDM
To overcome the challenges and ensure successful data integration and data mapping in MDM, organizations should follow these best practices:
1. Define Clear Data Integration and Mapping Goals
Organizations should clearly define their data integration and mapping goals and align them with their overall MDM strategy. This includes identifying the data sources, determining the scope of integration, and establishing the desired outcome.
2. Conduct Data Profiling and Analysis
Prior to integration and mapping, organizations should conduct data profiling and analysis to understand the structure, quality, and complexity of the data. This helps in identifying potential challenges and designing appropriate integration and mapping strategies.
3. Establish Data Standards and Policies
Defining data standards and policies is crucial for ensuring consistency and accuracy during integration and mapping. Organizations should establish guidelines for data formats, naming conventions, and data validation rules to maintain data quality.
4. Invest in Data Quality Management
Data quality management should be a continuous process throughout data integration and mapping. Organizations should invest in data cleansing, validation, and enrichment to eliminate errors and inconsistencies in the integrated data.
5. Implement Data Governance Framework
Effective data governance is essential for successful data integration and mapping. Organizations should establish a data governance framework that includes clear roles and responsibilities, data ownership, and data stewardship to ensure compliance and data security.
6. Leverage MDM Tools and Technologies
Utilizing MDM tools and technologies can simplify the data integration and mapping process. These tools provide features such as data profiling, data transformation, and data matching, which streamline the integration and mapping workflows.
7. Monitor and Measure Data Integration and Mapping
Organizations should regularly monitor and measure the effectiveness of data integration and mapping processes. This includes tracking data quality metrics, identifying data inconsistencies, and continuously improving the integration and mapping strategies.
Conclusion
Data integration and data mapping are critical components of MDM that enable organizations to create a single, trusted, and accurate version of master data. By integrating and mapping data from various sources, organizations can improve data quality, enhance data governance, increase operational efficiency, and enable data-driven decision making. However, organizations must also address challenges such as data complexity, data quality management, and legacy systems to ensure successful implementation. By following best practices and leveraging MDM tools and technologies, organizations can overcome these challenges and achieve the benefits of effective data integration and data mapping in MDM.
Contact us
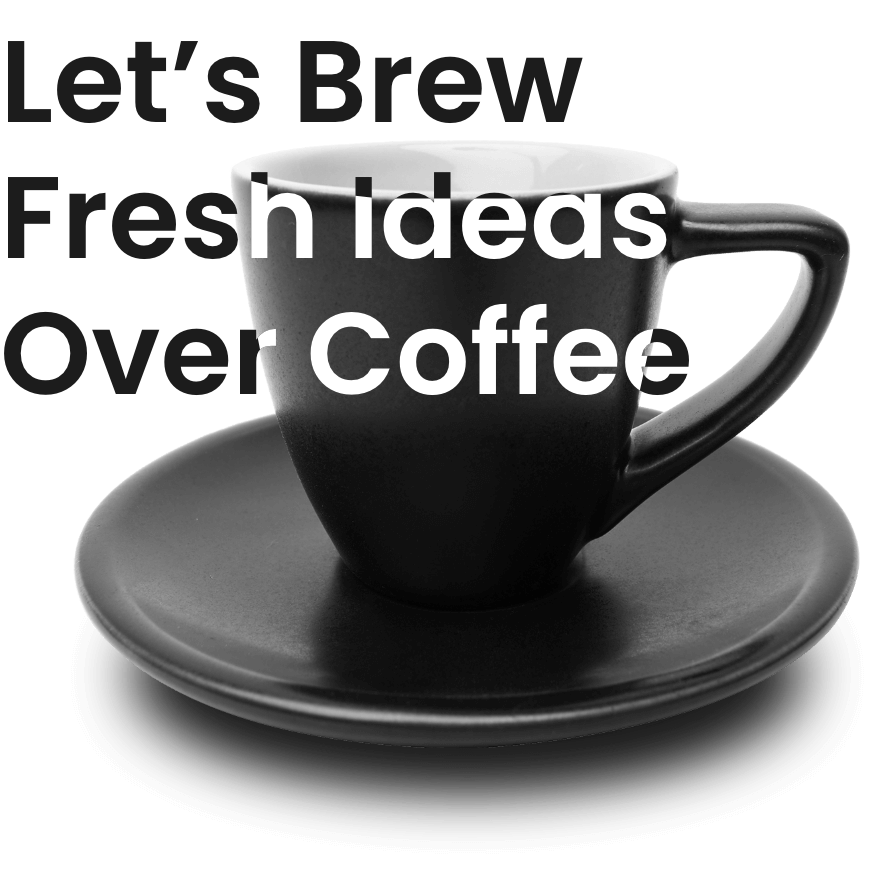
Spanning 8 cities worldwide and with partners in 100 more, we’re your local yet global agency.
Fancy a coffee, virtual or physical? It’s on us – let’s connect!