Data integration and data stewardship in MDM
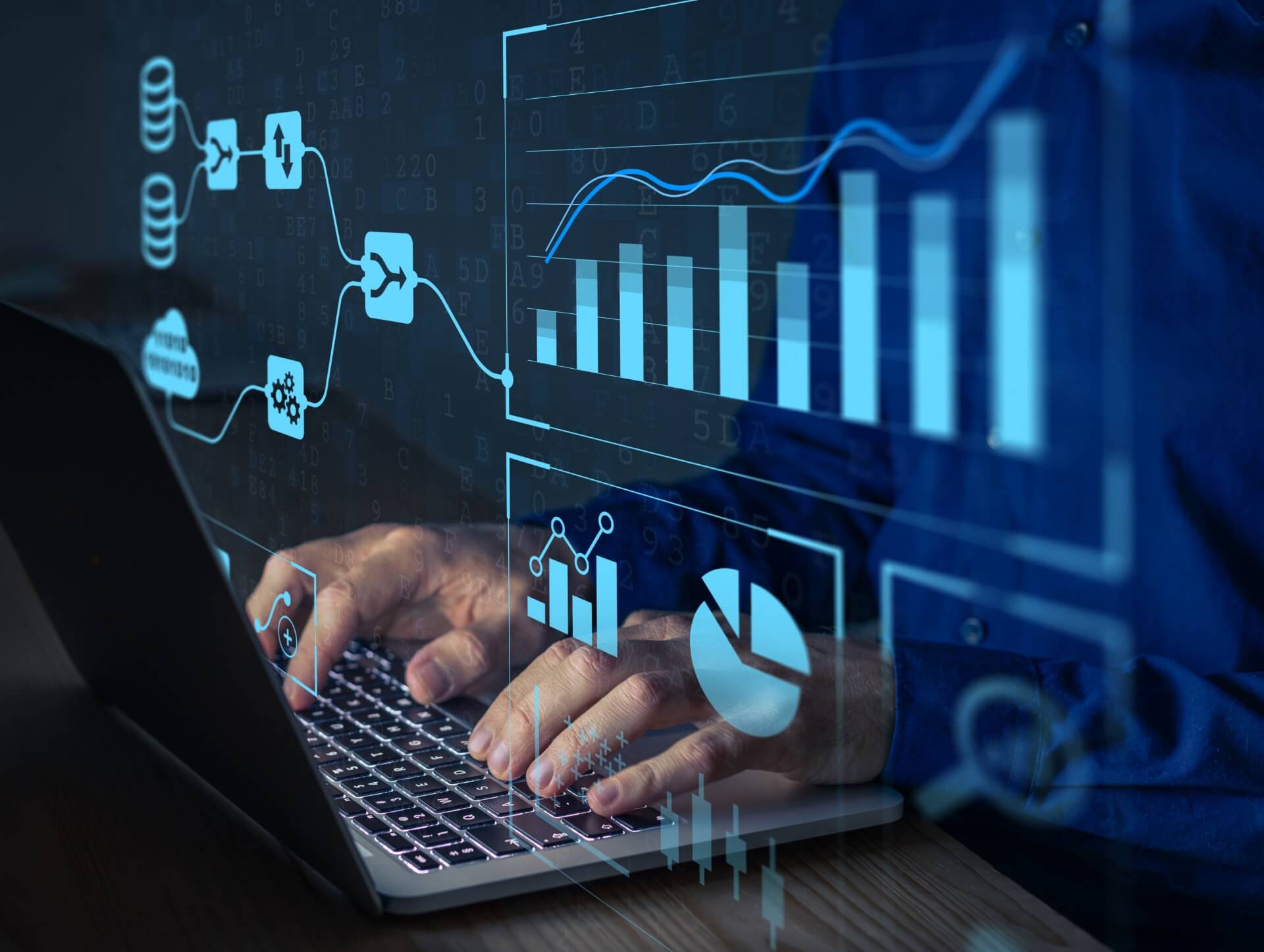
06/09/2023
Master Data Management (MDM) is a comprehensive approach to managing and integrating an organization's critical data assets. It involves creating and maintaining a single, reliable source of master data that can be shared across different systems and applications within the enterprise. MDM is essential for businesses looking to improve data quality, streamline processes, and enhance decision-making capabilities.
Understanding Data Integration in MDM
Data integration plays a crucial role in MDM, as it involves bringing together data from various sources and systems to create a unified view of master data. With effective data integration, organizations can ensure that the master data is accurate, consistent, and up-to-date. It enables businesses to have a 360-degree view of their customers, products, suppliers, and other critical entities.
Data integration in MDM involves the following steps:
1. Data Profiling and Cleansing
Data profiling helps organizations understand the quality and structure of their data. It involves analyzing the data to identify any inconsistencies, duplicates, or errors. Once the data profiling is complete, data cleansing is performed to remove or correct the identified issues. This ensures that the integrated master data is of high quality and can be trusted for decision-making purposes.
2. Data Mapping and Transformation
Data mapping involves identifying the fields and attributes in different source systems that correspond to the master data entities. It establishes the relationships between the source data and the master data. After data mapping, data transformation is carried out to convert the source data into a format that can be easily integrated with the master data. This step ensures that the integrated master data is consistent and accurate.
3. Data Integration and Consolidation
Once the data mapping and transformation are complete, the actual integration and consolidation of data take place. This involves combining the master data with the transformed and cleansed data from the different source systems. The integrated data is then stored in a central repository, commonly known as the master data management platform. This platform serves as the single source of truth for the organization's master data.
4. Data Validation and Governance
Data validation is crucial to ensure the accuracy and integrity of the integrated master data. It involves performing data quality checks and validations to identify any discrepancies or inconsistencies. Data governance is also implemented to define and enforce policies and procedures for managing the master data throughout its lifecycle. This ensures that the master data remains accurate, consistent, and compliant with regulatory requirements.
Importance of Data Stewardship in MDM
Data stewardship is the process of managing and ensuring the quality, integrity, and security of an organization's data assets. In the context of MDM, data stewardship involves assigning responsibilities and accountabilities for managing the master data. Data stewards are responsible for maintaining data quality, resolving data-related issues, and enforcing data governance policies and procedures.
Data stewardship in MDM includes the following activities:
1. Data Ownership and Accountability
Data stewards take ownership of specific sets of master data and are accountable for their quality and accuracy. They ensure that the master data is complete, consistent, and up-to-date. They also work closely with data users and stakeholders to understand their data requirements and address any data-related issues or concerns.
2. Data Quality Management
Data stewards are responsible for monitoring and improving the quality of the master data. They define data quality metrics and establish data quality rules and standards. They also conduct regular data quality assessments and implement corrective actions to address any data quality issues. By ensuring high-quality master data, organizations can make more informed decisions and achieve better business outcomes.
3. Data Governance and Compliance
Data stewards play a key role in implementing data governance practices and ensuring compliance with data management policies and regulations. They define data governance frameworks, establish data governance committees, and enforce data governance policies and procedures. They also work with legal and regulatory teams to ensure that the organization's master data is compliant with relevant data protection and privacy laws.
4. Data Security and Access Control
Data stewards are responsible for ensuring the security and confidentiality of the master data. They define data security policies and access control mechanisms to protect the master data from unauthorized access, use, or disclosure. They also monitor data access activities and investigate any data security incidents or breaches. By implementing robust data security measures, organizations can mitigate the risk of data breaches and safeguard their sensitive information.
Challenges in Data Integration and Data Stewardship
While data integration and data stewardship are crucial components of MDM, organizations often face several challenges in implementing and managing them effectively. Some of the common challenges include:
1. Data Complexity and Volume
Organizations deal with large volumes of data from multiple sources, making data integration and stewardship complex. Managing and integrating diverse data types, formats, and structures can be challenging, especially when dealing with big data and real-time data streams. Organizations need to invest in robust data integration and stewardship tools and technologies to handle the complexity and volume of data effectively.
2. Data Governance and Compliance
Implementing data governance practices and ensuring compliance with data management regulations can be challenging. Organizations need to define clear data governance frameworks, establish data governance committees, and enforce data governance policies and procedures. They also need to stay updated with the evolving data protection and privacy laws to ensure compliance. Lack of proper data governance can lead to data quality issues, security breaches, and legal and regulatory penalties.
3. Data Quality and Consistency
Ensuring data quality and consistency is a critical challenge in MDM. Poor data quality can lead to inaccurate insights and decisions, impacting business outcomes. Organizations need to invest in data quality management tools and technologies to identify and resolve data quality issues. They also need to establish data quality metrics and implement data quality monitoring processes to maintain the integrity and reliability of the master data.
4. Data Security and Privacy
Data security and privacy are major concerns in MDM. Organizations need to implement robust data security measures to protect the master data from unauthorized access, use, or disclosure. They need to establish data access controls, encryption mechanisms, and data masking techniques to safeguard sensitive information. They also need to comply with data protection and privacy laws to maintain customer trust and loyalty.
Best Practices for Data Integration and Data Stewardship in MDM
To overcome the challenges and ensure successful data integration and data stewardship in MDM, organizations can follow these best practices:
1. Define a Clear MDM Strategy
Before implementing data integration and data stewardship practices, organizations should define a clear MDM strategy. This strategy should align with the organization's overall business goals and objectives. It should outline the key stakeholders, data governance framework, data quality metrics, and security and privacy requirements. A well-defined MDM strategy provides a roadmap for effective data integration and stewardship.
2. Invest in Robust MDM Tools and Technologies
Organizations should invest in robust MDM tools and technologies to support data integration and data stewardship activities. These tools should have features like data profiling, data cleansing, data mapping, data transformation, data integration, and data governance. They should also support data security and privacy requirements. Choosing the right MDM tools and technologies can streamline and automate data integration and stewardship processes.
3. Establish Data Governance Frameworks
Data governance is essential for successful data integration and stewardship. Organizations should establish data governance frameworks that define roles, responsibilities, and processes for managing the master data. Data governance committees should be formed to oversee data governance activities and ensure compliance with data management policies and regulations. Regular data governance audits should be conducted to assess the effectiveness of data governance practices.
4. Implement Data Quality Management Processes
Data quality management is crucial for maintaining the integrity and reliability of the master data. Organizations should implement data quality management processes that include data profiling, data cleansing, data validation, and data monitoring. Data quality metrics should be defined, and data quality rules and standards should be established. Regular data quality assessments should be conducted, and corrective actions should be taken to address any data quality issues.
5. Train and Empower Data Stewards
Data stewards play a vital role in data integration and stewardship. Organizations should provide proper training and empower data stewards with the necessary skills and knowledge to perform their roles effectively. They should understand the organization's data governance policies and procedures and be equipped with the right tools and technologies. Data stewards should also have strong communication and collaboration skills to work with data users and stakeholders.
6. Monitor and Measure MDM Performance
Organizations should regularly monitor and measure the performance of their MDM initiatives. Key performance indicators (KPIs) should be defined to track the effectiveness of data integration and stewardship processes. Metrics such as data quality, data accuracy, data consistency, and data security should be monitored. Any deviations or issues should be addressed promptly to ensure continuous improvement in MDM practices.
7. Continuously Improve MDM Practices
MDM is an ongoing process, and organizations should continuously strive to improve their data integration and stewardship practices. They should stay updated with the latest trends and advancements in MDM and leverage new tools and technologies to enhance their MDM capabilities. Feedback from data users and stakeholders should be collected and incorporated into the MDM processes. Continuous improvement in MDM practices leads to better data quality, increased operational efficiency, and improved business outcomes.
Conclusion
Data integration and data stewardship are critical components of Master Data Management (MDM). Effective data integration ensures the accuracy and consistency of master data, while data stewardship ensures the quality, integrity, and security of the master data. Organizations face challenges in implementing and managing data integration and stewardship, but by following best practices and investing in robust MDM tools and technologies, they can overcome these challenges and achieve the benefits of MDM. Successful data integration and stewardship in MDM lead to improved data quality, streamlined processes, enhanced decision-making capabilities, and better business outcomes.
Contact us
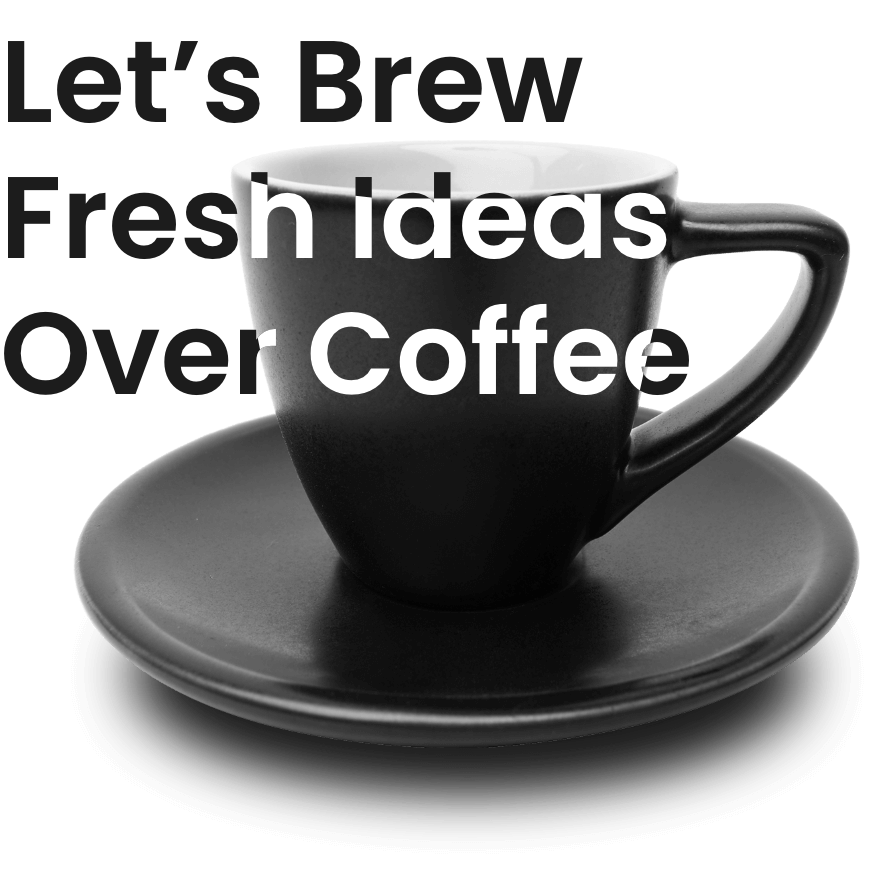
Spanning 8 cities worldwide and with partners in 100 more, we’re your local yet global agency.
Fancy a coffee, virtual or physical? It’s on us – let’s connect!