Data integration and data transformation in MDM
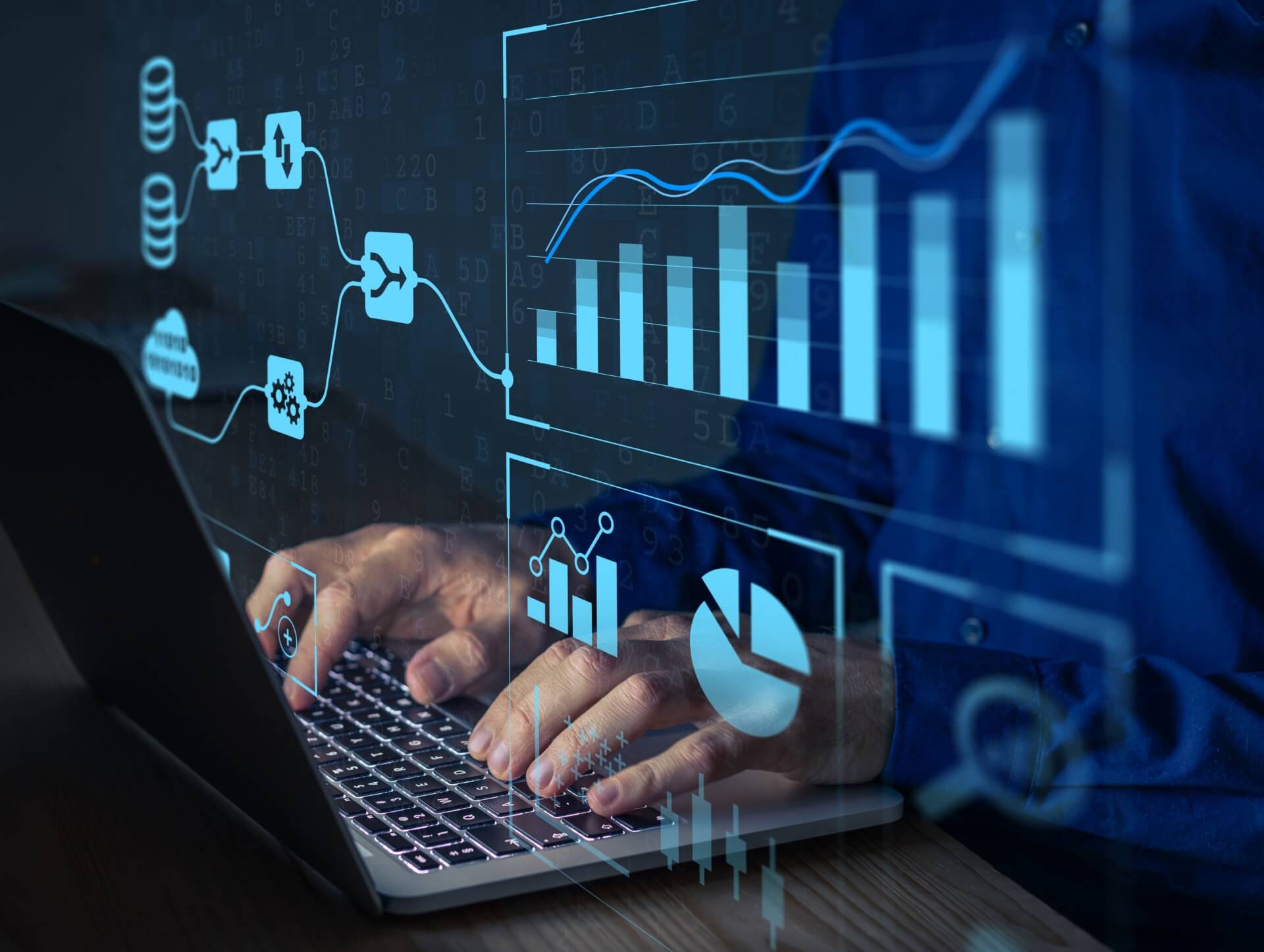
06/09/2023
Introduction to Master Data Management (MDM)
Master Data Management (MDM) is a comprehensive approach to managing and integrating an organization's critical data assets. It involves the establishment and maintenance of consistent, accurate, and reliable master data across various systems and applications.
MDM aims to provide a single, authoritative source of truth for master data, such as customer data, product data, supplier data, and employee data. By ensuring data consistency and integrity, MDM enables organizations to make informed business decisions, improve operational efficiency, and enhance customer satisfaction.
The Importance of Data Integration in MDM
Data integration plays a crucial role in MDM as it involves combining data from multiple sources and formats into a unified and consistent view. It enables organizations to create a holistic and accurate representation of master data, eliminating redundancies and inconsistencies.
With effective data integration, organizations can achieve a 360-degree view of their master data, enabling better decision-making and improved operational efficiency. It also enhances data quality and improves data governance by ensuring that data is standardized, cleansed, and validated before being integrated into the MDM system.
Data Transformation in MDM
Data transformation is the process of converting and mapping data from its source format to the desired format for integration into the MDM system. It involves various activities, such as data cleansing, data enrichment, data standardization, and data validation.
Data transformation ensures that data is accurate, consistent, and compliant with the defined data standards and business rules. It also involves resolving data conflicts and inconsistencies, such as duplicate records or conflicting data values, to create a single version of truth for master data.
Benefits of Data Integration and Data Transformation in MDM
Effective data integration and data transformation in MDM offer several benefits to organizations:
1. Improved Data Quality:
Data integration and transformation processes enable organizations to cleanse, standardize, and validate data, resulting in improved data quality. By removing duplicates, resolving conflicts, and ensuring consistency, organizations can rely on accurate and reliable master data for decision-making and operational processes.
2. Enhanced Data Governance:
Data integration and transformation contribute to better data governance by enforcing data standards, ensuring compliance with regulations, and improving data security. It enables organizations to establish and enforce data policies, monitor data quality, and track data lineage throughout the MDM lifecycle.
3. Increased Operational Efficiency:
By integrating and transforming data from various sources into a unified view, organizations can streamline business processes and improve operational efficiency. It eliminates the need for manual data entry and reconciliation, reduces errors, and enables faster access to accurate data across the organization.
4. Improved Decision-Making:
With a comprehensive and accurate view of master data, organizations can make informed decisions based on reliable and up-to-date information. Data integration and transformation enable organizations to analyze data more effectively, identify trends, and gain valuable insights for strategic planning and business growth.
5. Enhanced Customer Experience:
By integrating customer data from various sources, organizations can gain a 360-degree view of their customers, enabling personalized and targeted marketing campaigns, improved customer service, and enhanced customer experience. It allows organizations to understand customer preferences, behavior, and needs, leading to increased customer satisfaction and loyalty.
Challenges in Data Integration and Data Transformation in MDM
While data integration and transformation are crucial for successful MDM implementation, organizations often face several challenges:
1. Data Complexity:
Organizations often deal with large volumes of complex and diverse data from multiple sources, making data integration and transformation complex and time-consuming. Data may be stored in different formats, have inconsistent data structures, or contain missing or inaccurate information.
2. Data Quality Issues:
Poor data quality, such as duplicate records, inconsistent data values, or outdated information, can hinder data integration and transformation efforts. Organizations need to invest in data cleansing, standardization, and validation processes to ensure accurate and reliable master data.
3. Data Security and Privacy:
Data integration and transformation involve the movement and consolidation of sensitive and confidential data. Organizations need to ensure data security and privacy throughout the process to comply with regulations and protect sensitive information from unauthorized access or misuse.
4. Data Governance and Compliance:
Data integration and transformation require organizations to establish robust data governance practices and comply with data regulations and industry standards. It involves defining data policies, roles, and responsibilities, and ensuring data consistency, accuracy, and compliance throughout the MDM lifecycle.
Best Practices for Data Integration and Data Transformation in MDM
To overcome the challenges and ensure successful data integration and transformation in MDM, organizations should follow these best practices:
1. Define Data Governance Framework:
Establish a comprehensive data governance framework that includes data policies, standards, and guidelines. Define roles and responsibilities for data stewards and data owners to ensure accountability and compliance throughout the MDM lifecycle.
2. Invest in Data Quality Management:
Implement data quality management processes, such as data cleansing, standardization, and validation, to improve data quality. Regularly monitor and measure data quality metrics to identify and resolve data quality issues proactively.
3. Implement Data Integration and Transformation Tools:
Invest in data integration and transformation tools and technologies that support data mapping, data cleansing, and data transformation activities. These tools automate the integration and transformation processes, reducing manual effort and improving efficiency.
4. Ensure Data Security and Privacy:
Implement robust data security measures, such as encryption, access controls, and data masking, to protect sensitive data during data integration and transformation. Comply with data privacy regulations, such as GDPR, and ensure data privacy throughout the MDM lifecycle.
5. Adopt Data Integration and Transformation Standards:
Follow industry best practices and standards, such as XML, JSON, or CSV, for data integration and transformation. Use standardized data formats and data models to ensure compatibility and interoperability with other systems and applications.
Conclusion
Data integration and data transformation are critical components of successful MDM implementation. They enable organizations to achieve a unified and accurate view of master data, improve data quality, enhance data governance, and drive operational efficiency. By following best practices and investing in the right tools and technologies, organizations can overcome challenges and reap the benefits of effective data integration and data transformation in MDM.
Contact us
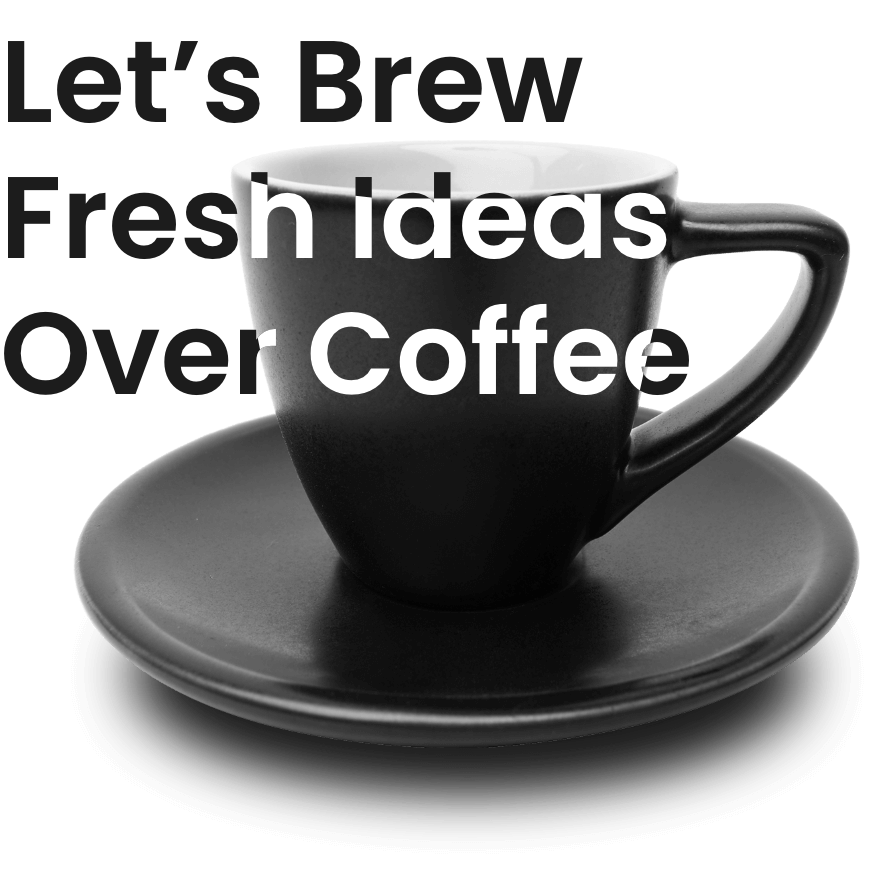
Spanning 8 cities worldwide and with partners in 100 more, we’re your local yet global agency.
Fancy a coffee, virtual or physical? It’s on us – let’s connect!