Master data modeling for data governance in healthcare
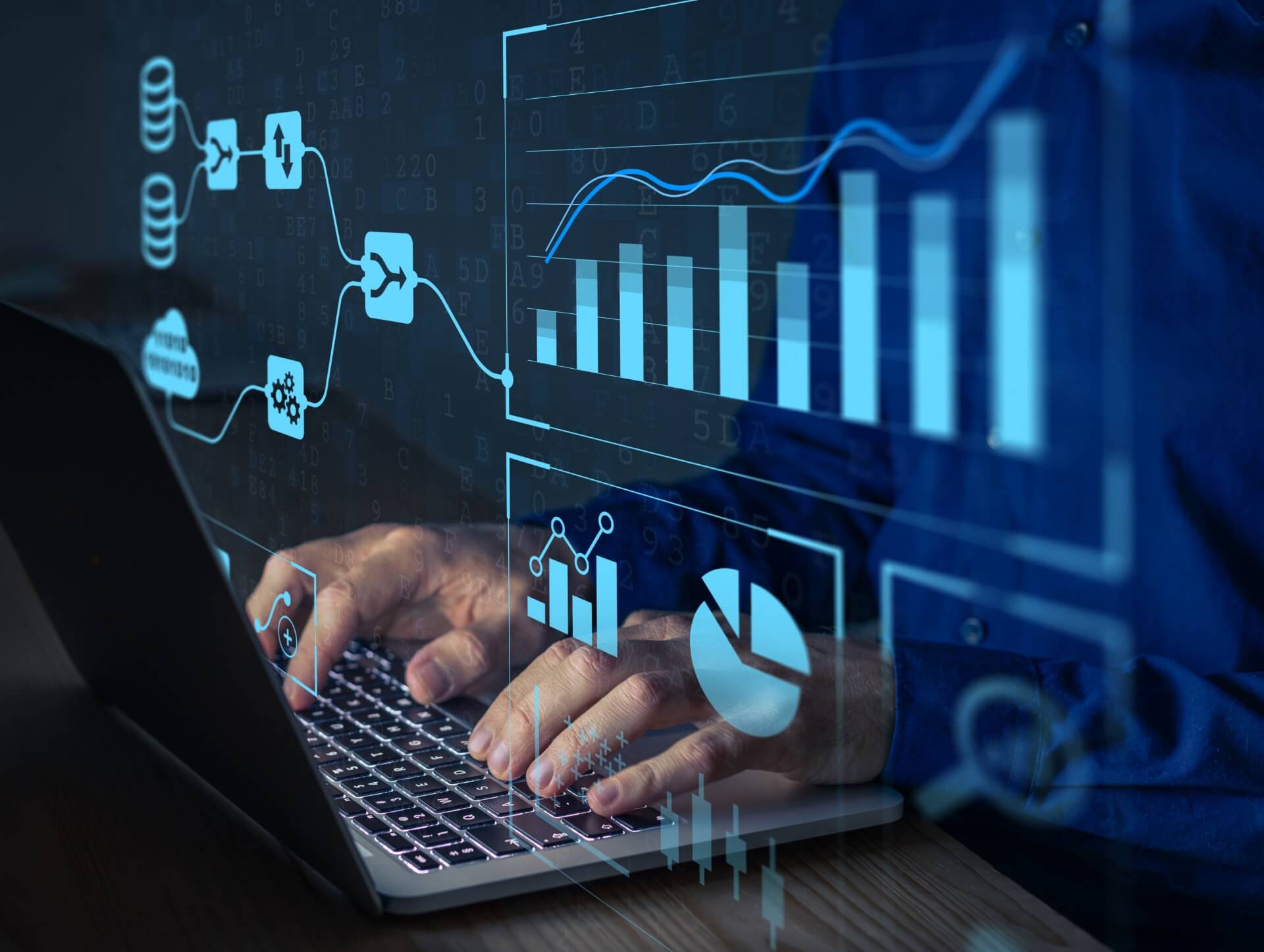
06/09/2023
Master data modeling is a critical component of data governance in healthcare. It involves creating a structured representation of the essential data entities and their relationships within an organization. By implementing effective master data modeling, healthcare organizations can ensure data accuracy, consistency, and integrity, leading to improved decision-making, operational efficiency, and patient care.
The Importance of Master Data Modeling
Master data modeling plays a crucial role in healthcare data governance for several reasons:
- Data Accuracy: By defining and standardizing data elements and their relationships, master data modeling ensures the accuracy and consistency of data across the organization. This is particularly important in healthcare, where accurate and reliable data is essential for patient safety and quality of care.
- Decision-Making: Master data modeling provides a foundation for data-driven decision-making in healthcare organizations. By having a clear understanding of the relationships between data entities, healthcare professionals can analyze and interpret data more effectively, leading to better decisions and outcomes.
- Operational Efficiency: Master data modeling enables efficient data integration and interoperability across different systems and departments within a healthcare organization. This streamlines processes, reduces duplication of effort, and improves overall operational efficiency.
- Regulatory Compliance: Healthcare organizations are subject to numerous regulations and compliance requirements. Master data modeling helps ensure compliance by providing a centralized and standardized view of data, making it easier to monitor and report on data-related activities.
Best Practices for Master Data Modeling in Healthcare
Effective master data modeling requires adherence to best practices to ensure its success. Here are some key best practices for master data modeling in healthcare:
1. Understand the Business Context
Before embarking on master data modeling, it is crucial to understand the specific business context and requirements of the healthcare organization. This includes identifying the key data entities, their attributes, and the relationships between them. By understanding the business context, healthcare organizations can ensure that the master data model aligns with their specific needs and goals.
2. Involve Stakeholders and Subject Matter Experts
Master data modeling should be a collaborative effort involving key stakeholders and subject matter experts from various departments within the healthcare organization. This ensures that all relevant perspectives are considered and that the resulting master data model accurately represents the organization's data landscape.
3. Standardize Data Definitions and Formats
Standardizing data definitions and formats is essential for ensuring data consistency and interoperability. Healthcare organizations should establish clear guidelines and standards for data definitions, naming conventions, and data formats. This promotes data quality and facilitates data integration and sharing across different systems and departments.
4. Implement Data Governance Framework
Data governance is a critical aspect of master data modeling in healthcare. Healthcare organizations should establish a data governance framework that defines roles, responsibilities, and processes for managing and maintaining master data. This includes data stewardship, data quality management, and data lifecycle management.
Challenges in Master Data Modeling for Data Governance
While master data modeling offers numerous benefits, it also presents several challenges that healthcare organizations need to address:
1. Data Complexity
Healthcare data is inherently complex, with numerous data entities, attributes, and relationships. Master data modeling requires a deep understanding of the underlying data complexities to ensure accurate representation and effective data governance.
2. Data Integration and Interoperability
Healthcare organizations often have multiple systems and applications that store and manage different types of data. Master data modeling needs to consider the integration and interoperability requirements to ensure seamless data exchange and sharing across these systems.
3. Data Quality Management
Poor data quality can have significant implications for patient care and decision-making. Master data modeling should incorporate data quality management practices to ensure data accuracy, completeness, and consistency.
4. Data Security and Privacy
Healthcare data is highly sensitive and subject to strict security and privacy regulations. Master data modeling needs to consider data security and privacy requirements to protect patient information and comply with applicable regulations.
Case Studies: Successful Master Data Modeling in Healthcare
Several healthcare organizations have successfully implemented master data modeling for data governance. Here are two case studies that highlight the benefits and challenges of master data modeling:
Case Study 1: Hospital A
Hospital A, a large healthcare organization, implemented a master data management solution to improve data governance and decision-making. By creating a comprehensive master data model that included patient data, medical procedures, and healthcare providers, Hospital A was able to streamline data integration, improve data quality, and enhance patient care outcomes.
Case Study 2: Healthcare System B
Healthcare System B, a regional healthcare system, implemented a cloud-based master data management platform to address data integration challenges across its multiple facilities. The master data model included data entities such as patient demographics, insurance information, and clinical data. By adopting a cloud-based approach, Healthcare System B achieved greater scalability, flexibility, and cost-efficiency in managing its master data.
Conclusion
Master data modeling is a critical component of data governance in healthcare. It enables healthcare organizations to achieve data accuracy, consistency, and integrity, leading to improved decision-making, operational efficiency, and patient care. By following best practices and addressing the challenges associated with master data modeling, healthcare organizations can unlock the full potential of their data and drive better health outcomes.
References
1. Smith, J. (2020). The Role of Master Data Modeling in Healthcare Data Governance. Journal of Healthcare Data Management.
2. Johnson, M. (2019). Master Data Management in Healthcare: Best Practices and Case Studies. Healthcare IT Today.
3. Healthcare Organization X. (2021). Master Data Modeling Case Study: Improving Data Governance and Decision-Making.
4. Healthcare System Y. (2020). Cloud-Based Master Data Management: A Case Study in Data Integration and Scalability.
Contact us
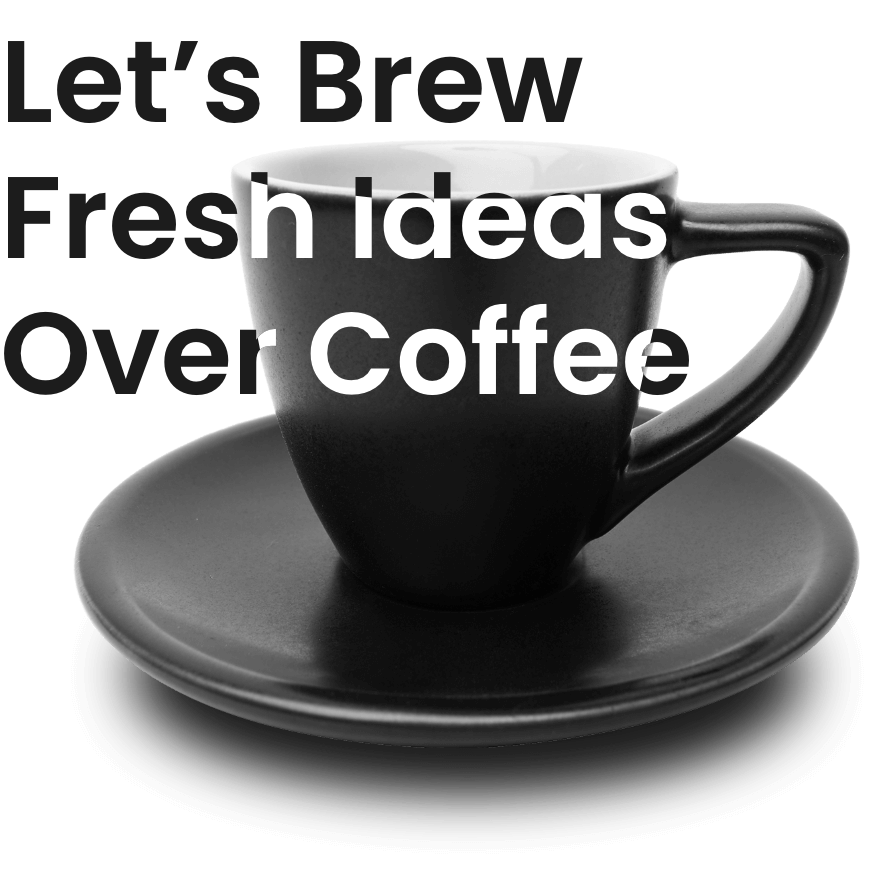
Spanning 8 cities worldwide and with partners in 100 more, we’re your local yet global agency.
Fancy a coffee, virtual or physical? It’s on us – let’s connect!