Master data modeling for data integration in the Internet of Things (IoT)
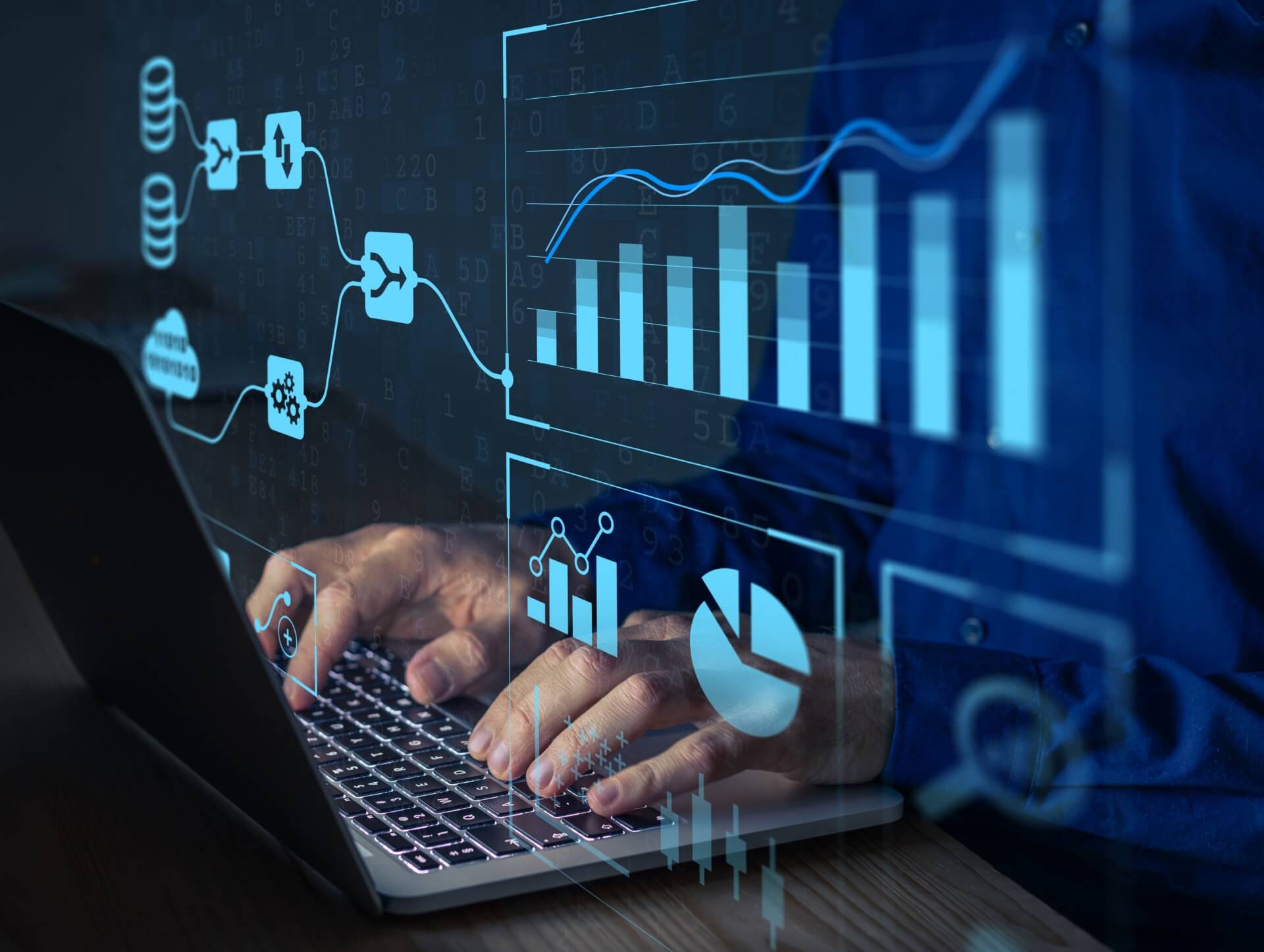
06/09/2023
In the rapidly evolving world of technology and data, the Internet of Things (IoT) has emerged as a game-changer. IoT is the network of interconnected devices and sensors that collect and exchange data. It has revolutionized various industries, from healthcare to manufacturing, by enabling real-time monitoring, automation, and data-driven decision-making. However, harnessing the full potential of IoT requires efficient data integration and management. This is where master data modeling comes into play.
What is Master Data Modeling?
Master data modeling is the process of defining and organizing the key data entities and their relationships within an organization. It involves creating a comprehensive data model that represents the structure and semantics of the data. This model serves as a blueprint for data integration and ensures consistency, accuracy, and quality of data across different systems and applications. Master data modeling is essential for effective data governance, data management, and data integration in the IoT landscape.
The Importance of Master Data Modeling for Data Integration in IoT
Master data modeling plays a crucial role in enabling seamless data integration in the IoT ecosystem. Here are some key reasons why it is important:
1. Ensures Data Consistency and Accuracy
In the IoT environment, data is generated and collected from various sources, such as sensors, devices, and applications. This data often comes in different formats and structures. Master data modeling helps in standardizing and harmonizing this data by defining common data elements and their relationships. It ensures that data is consistent and accurate, enabling meaningful insights and analysis.
2. Facilitates Data Governance and Compliance
Data governance is a critical aspect of data integration in the IoT. It involves defining policies, procedures, and controls for managing data throughout its lifecycle. Master data modeling provides a foundation for effective data governance by establishing data ownership, data quality standards, and data security measures. It enables organizations to comply with regulatory requirements and industry standards, such as GDPR and ISO 27001.
3. Enables Data Integration and Interoperability
In the IoT landscape, data integration is a complex process due to the heterogeneity of devices, protocols, and data formats. Master data modeling helps in mapping and transforming data from different sources, making it compatible for integration. It enables interoperability between systems and applications, allowing seamless data exchange and collaboration. This ensures that the right data is available at the right time and in the right format for decision-making.
4. Supports Data Analytics and Insights
Data analytics is a key driver of value in the IoT ecosystem. By leveraging advanced analytics techniques, organizations can extract meaningful insights from the vast amount of data generated by IoT devices. Master data modeling provides a structured and organized view of data, making it easier to analyze and derive actionable insights. It enables organizations to identify trends, patterns, and anomalies, leading to data-driven decision-making and improved business outcomes.
Challenges in Master Data Modeling for Data Integration in IoT
While master data modeling is essential for effective data integration in the IoT, there are several challenges that organizations need to overcome:
1. Data Complexity and Volume
In the IoT landscape, data is generated at an unprecedented scale and velocity. Managing and modeling such large volumes of data can be challenging. Organizations need to invest in scalable master data management solutions and platforms that can handle the complexity and volume of IoT data.
2. Data Quality and Integrity
Data quality is a critical factor in master data modeling. In the IoT, data is collected from various sources with varying degrees of accuracy and reliability. Ensuring data quality and integrity requires robust data cleansing, validation, and enrichment processes. Organizations need to implement data quality management practices and tools to address these challenges.
3. Data Security and Privacy
With the increasing number of connected devices and the sensitive nature of IoT data, data security and privacy are major concerns. Organizations need to implement strong data security measures, such as encryption and access controls, to protect sensitive data. They also need to comply with data protection regulations and ensure privacy by design in their master data modeling process.
4. Integration Complexity
Integrating data from diverse sources, such as sensors, devices, and legacy systems, can be complex. Organizations need to invest in integration technologies and platforms that support data transformation, mapping, and synchronization. They also need to define clear data integration strategies and establish data integration best practices to overcome integration challenges.
Best Practices for Master Data Modeling for Data Integration in IoT
To overcome the challenges and ensure successful master data modeling for data integration in the IoT, organizations should follow these best practices:
1. Define a Clear Master Data Management Strategy
A well-defined master data management (MDM) strategy is the foundation for effective data integration in the IoT. Organizations should clearly define their MDM goals, objectives, and target outcomes. They should identify the key data entities and their relationships that are critical for their business operations. This will help in creating a comprehensive master data model that aligns with their business needs.
2. Invest in the Right MDM Tools and Technologies
Choosing the right MDM tools and technologies is crucial for successful master data modeling. Organizations should evaluate and select MDM solutions that can handle the complexity and volume of IoT data. They should consider factors such as scalability, flexibility, data governance capabilities, and integration capabilities. Open source and cloud-based MDM solutions can be cost-effective options for organizations.
3. Establish Data Governance and Data Quality Management Processes
Data governance is the key to ensuring data consistency, accuracy, and integrity in the IoT ecosystem. Organizations should establish data governance processes and policies that define data ownership, data quality standards, and data security measures. They should implement data quality management practices, such as data cleansing, validation, and enrichment, to ensure high-quality data for master data modeling.
4. Implement a Robust Data Integration Architecture
A well-designed data integration architecture is essential for seamless data integration in the IoT. Organizations should invest in integration technologies and platforms that support data transformation, mapping, and synchronization. They should define clear data integration strategies, such as batch processing or real-time streaming, based on their business requirements. Implementing data integration best practices, such as data mapping and data synchronization, can help in overcoming integration challenges.
Conclusion
Master data modeling is a critical component of data integration in the IoT landscape. It enables organizations to achieve data consistency, accuracy, and quality, ensuring meaningful insights and analysis. By following best practices and overcoming the challenges, organizations can harness the full potential of IoT and drive business growth. Effective master data modeling, combined with robust data governance and data integration strategies, is the key to success in the IoT era.
Contact us
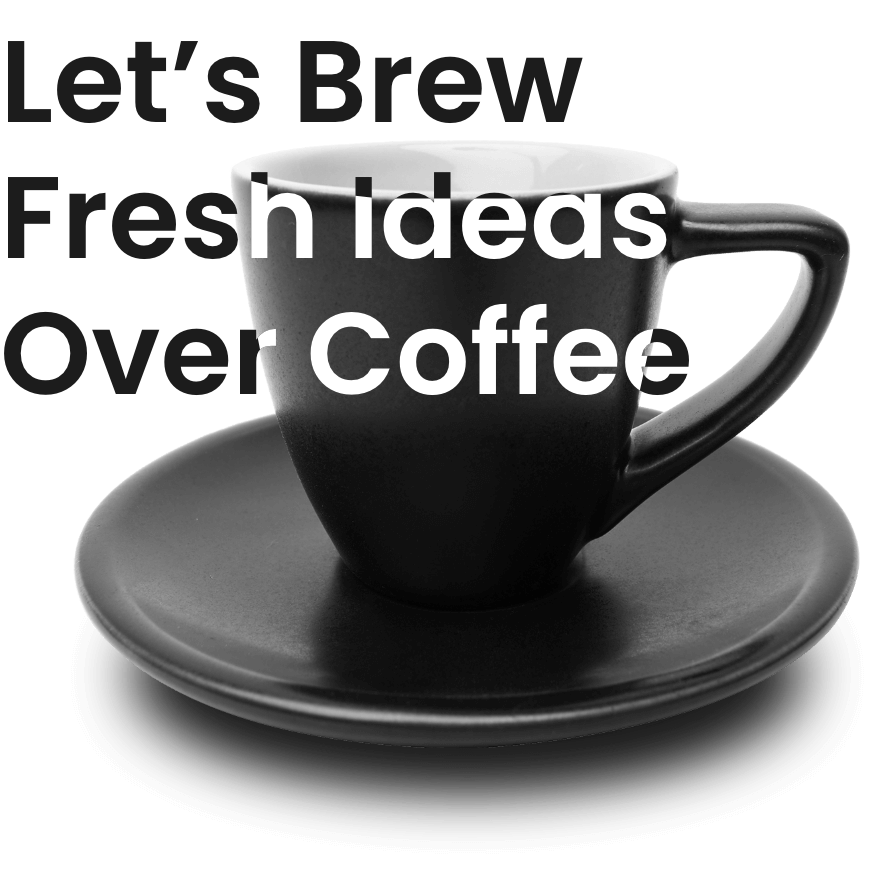
Spanning 8 cities worldwide and with partners in 100 more, we’re your local yet global agency.
Fancy a coffee, virtual or physical? It’s on us – let’s connect!