Master data modeling for improved decision-making
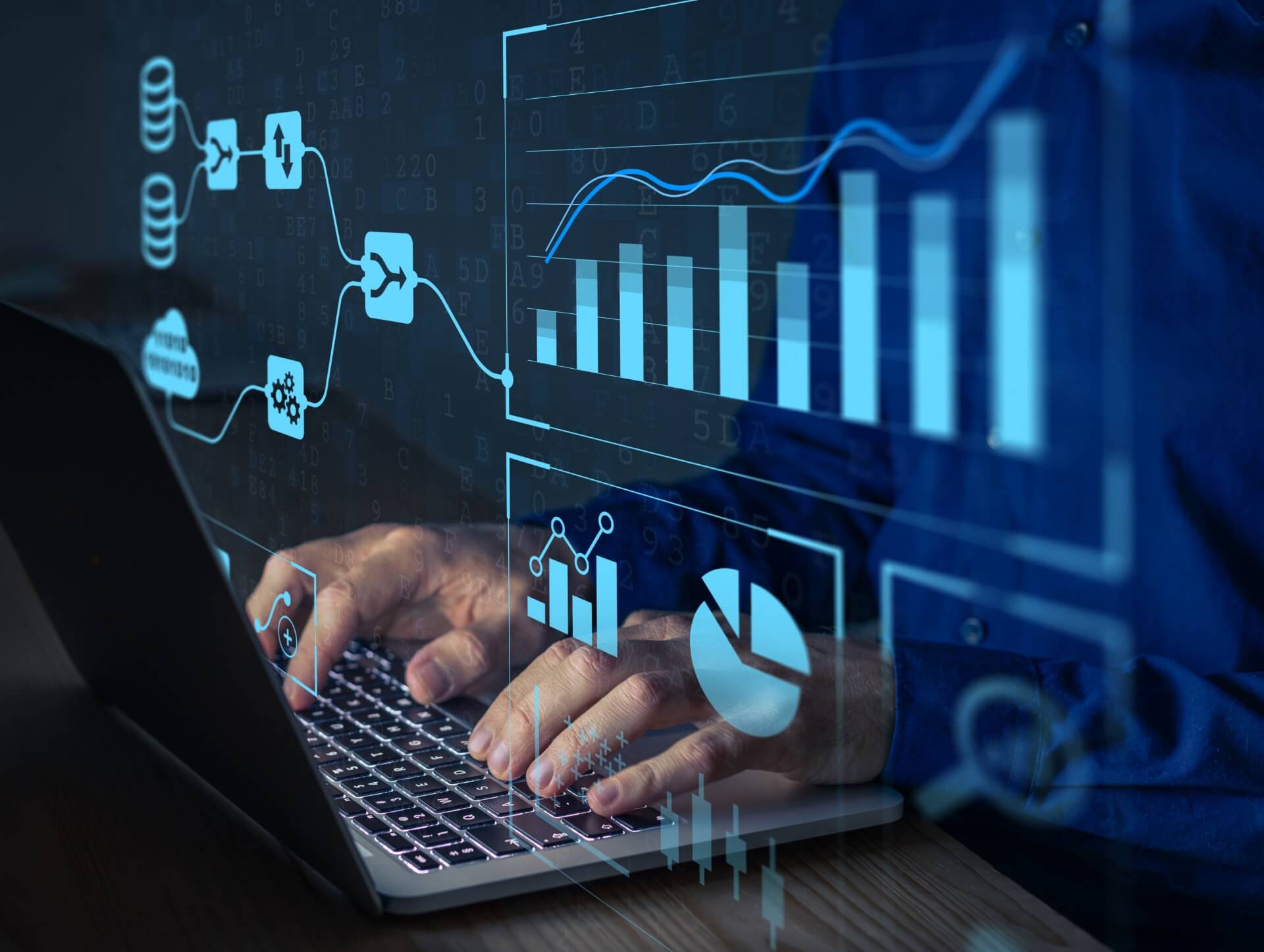
06/09/2023
Master data modeling plays a crucial role in the success of any business. It allows organizations to effectively manage their data assets, streamline processes, and make informed decisions. In this article, we will explore the concept of master data modeling and its benefits for businesses. We will also delve into best practices for master data management (MDM) and effective data lifecycle management. Let's dive in and discover how master data modeling can transform your business!
What is Master Data Modeling?
Master data modeling is the process of designing a structure or framework to organize and manage an organization's master data. Master data refers to the core data entities that are essential for running a business, such as customer information, product details, employee records, and more. Master data modeling involves defining the relationships, attributes, and hierarchies of these data entities to ensure consistency, accuracy, and integrity.
The Benefits of Master Data Management
Implementing a robust master data management strategy brings several benefits to businesses:
- Improved Data Quality: By implementing master data management solutions, organizations can ensure that their data is accurate, consistent, and up-to-date. This leads to improved decision-making and better business outcomes.
- Enhanced Data Governance: Master data management enables organizations to establish and enforce data governance policies, ensuring compliance with regulations and industry standards.
- Streamlined Business Processes: With a centralized master data management platform, organizations can streamline their business processes, eliminate redundancies, and improve operational efficiency.
- Increased Customer Satisfaction: Effective customer master data management allows organizations to provide personalized experiences to their customers, leading to higher satisfaction and loyalty.
- Improved Data Security: Master data management services include robust security measures to protect sensitive data, reducing the risk of data breaches and unauthorized access.
Master Data Management Best Practices
To maximize the benefits of master data management, organizations should follow these best practices:
- Define Clear Goals and Objectives: Before implementing a master data management solution, it is crucial to define clear goals and objectives. This will help align the MDM strategy with the overall business strategy and ensure that the implementation process is focused and effective.
- Establish Data Governance Framework: Implementing a robust data governance framework is essential for successful master data management. Define data ownership, roles, responsibilities, and processes to ensure data accuracy, integrity, and security.
- Ensure Data Quality Management: Data quality is critical for effective master data management. Implement data quality management processes and tools to identify and resolve data quality issues, ensuring high-quality master data.
- Invest in User Training and Change Management: User adoption is crucial for the success of any master data management initiative. Invest in comprehensive user training programs and change management strategies to ensure that employees understand the importance of MDM and are equipped to use the MDM tools and technologies effectively.
- Implement Data Integration Strategies: To achieve a single, unified view of master data, organizations need to implement robust data integration strategies. This involves integrating data from various sources and systems, ensuring data consistency and accuracy.
- Continuously Monitor and Improve: Master data management is an ongoing process. Continuously monitor and measure the effectiveness of the MDM solution, identify areas for improvement, and implement necessary changes to optimize the MDM strategy.
Master Data Modeling and Data Lifecycle Management
Master data modeling is closely intertwined with data lifecycle management. Data lifecycle management refers to the process of managing data throughout its lifecycle, from creation to deletion. Let's explore how master data modeling fits into the data lifecycle:
Data Governance in Lifecycle Management
Data governance plays a crucial role in effective data lifecycle management. It involves establishing policies, processes, and controls to ensure data quality, integrity, and security throughout the data lifecycle. Master data modeling provides the foundation for data governance by defining the structure and relationships of master data entities.
Data Security Throughout the Lifecycle
Data security is a top priority for organizations in today's digital landscape. Master data modeling enables organizations to identify and categorize sensitive data, implement appropriate security measures, and ensure data security throughout the data lifecycle. This includes securing data at rest, in transit, and during processing.
Data Compliance in Management
Compliance with regulations and industry standards is essential for businesses across various sectors. Master data modeling enables organizations to define data attributes and metadata that are required for compliance. It also helps in establishing data lineage and audit trails, facilitating compliance audits and reporting.
Master Data in Lifecycle Management
Master data is a critical component of data lifecycle management. It serves as the foundation for other data entities and processes, such as transactional data and analytical data. Effective master data modeling ensures the integrity and consistency of master data throughout its lifecycle, enabling accurate and reliable reporting and analysis.
Metadata Management in Data Lifecycle
Metadata management is essential for effective data lifecycle management. Metadata provides context and meaning to data, facilitating data discovery, understanding, and governance. Master data modeling involves defining metadata attributes and relationships, ensuring that metadata is accurately captured and managed throughout the data lifecycle.
Automation in Data Lifecycle
Automation plays a crucial role in efficient data lifecycle management. Master data modeling enables organizations to automate data processes, such as data integration, data cleansing, and data validation. Automation reduces manual effort, improves data accuracy, and enhances operational efficiency.
Cloud-Based Data Lifecycle
Cloud technology has revolutionized data lifecycle management. Organizations can leverage cloud-based master data management solutions to store, manage, and analyze their data in a secure and scalable environment. Cloud-based solutions offer flexibility, cost savings, and easy accessibility, enabling organizations to effectively manage their data throughout its lifecycle.
Analytics in Data Lifecycle
Data analytics plays a crucial role in data-driven decision-making. Master data modeling enables organizations to structure and organize their data in a way that is conducive to data analytics. By leveraging master data management solutions, organizations can unlock valuable insights from their data, leading to better strategic decisions and business outcomes.
Challenges in Data Lifecycle Management
Data lifecycle management is not without its challenges. Some common challenges include:
- Data Silos: Organizations often struggle with data silos, where data is fragmented and stored in different systems, making it challenging to achieve a single, unified view of data.
- Data Quality Issues: Ensuring data quality throughout the data lifecycle can be a complex task. Data errors, inconsistencies, and duplications can affect the accuracy and reliability of data.
- Data Security Risks: With the increasing volume and complexity of data, organizations face significant data security risks. Protecting data from unauthorized access, data breaches, and cyber threats is a constant challenge.
- Legacy Systems and Integration: Integrating data from legacy systems into a modern master data management platform can be challenging. Legacy systems may have data formats and structures that are incompatible with modern systems.
- Change Management: Implementing a data lifecycle management strategy requires significant organizational change. Ensuring user adoption and overcoming resistance to change can be a challenge.
Best Practices for Lifecycle Management
To overcome the challenges in data lifecycle management, organizations should follow these best practices:
- Implement a Data Governance Framework: Establish a robust data governance framework to ensure data quality, integrity, and security throughout the data lifecycle. Define data ownership, roles, and responsibilities, and implement data governance processes and controls.
- Invest in Data Integration and Migration: Implement robust data integration and migration strategies to consolidate data from various sources and systems. This will enable organizations to achieve a single, unified view of data and eliminate data silos.
- Ensure Data Quality Management: Implement data quality management processes and tools to identify and resolve data quality issues. Regularly monitor and measure data quality to ensure high-quality, reliable data throughout the data lifecycle.
- Implement Robust Data Security Measures: Data security should be a top priority throughout the data lifecycle. Implement robust security measures, such as encryption, access controls, and regular security audits, to protect sensitive data from unauthorized access and cyber threats.
- Invest in User Training and Change Management: User adoption is crucial for the success of data lifecycle management initiatives. Invest in comprehensive user training programs and change management strategies to ensure that employees understand the importance of data lifecycle management and are equipped to use the necessary tools and technologies effectively.
- Continuously Monitor and Improve: Data lifecycle management is an ongoing process. Continuously monitor and measure the effectiveness of the data lifecycle management strategy, identify areas for improvement, and implement necessary changes to optimize the data lifecycle.
Conclusion
Master data modeling is a critical component of effective master data management and data lifecycle management. By implementing a robust master data management strategy and following best practices for data lifecycle management, organizations can improve data quality, streamline processes, and make informed decisions. Master data modeling provides the foundation for successful data management initiatives, enabling organizations to unlock the true value of their data. Embrace master data modeling and take your business to new heights!
Contact us
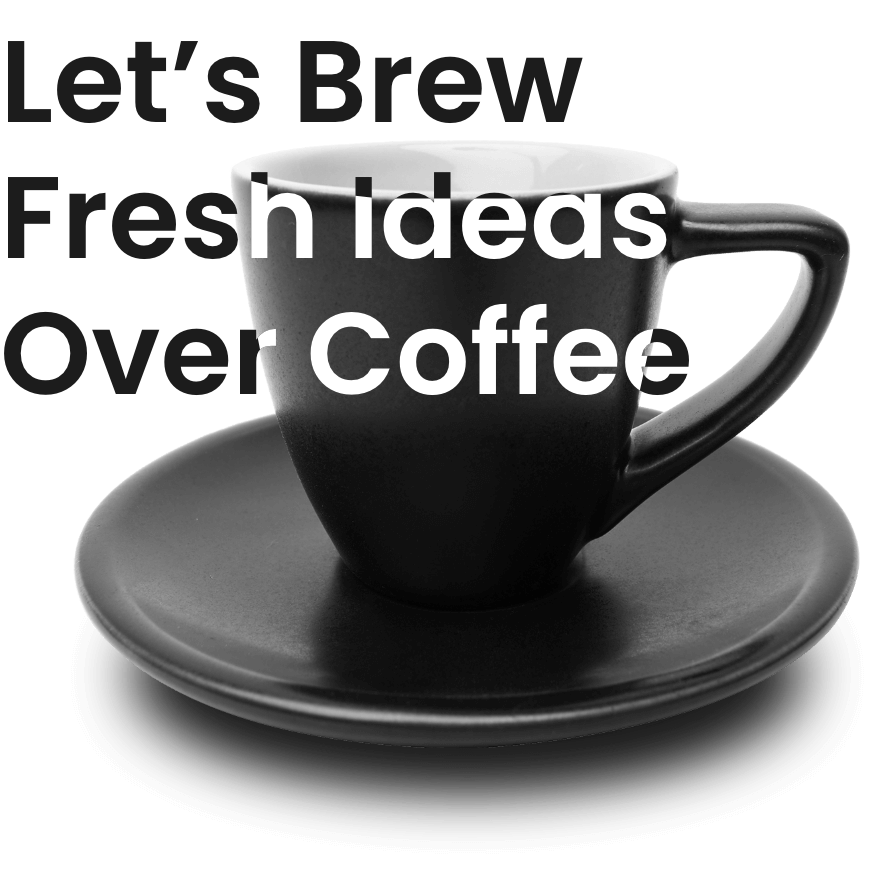
Spanning 8 cities worldwide and with partners in 100 more, we’re your local yet global agency.
Fancy a coffee, virtual or physical? It’s on us – let’s connect!