Master data modeling for regulatory compliance
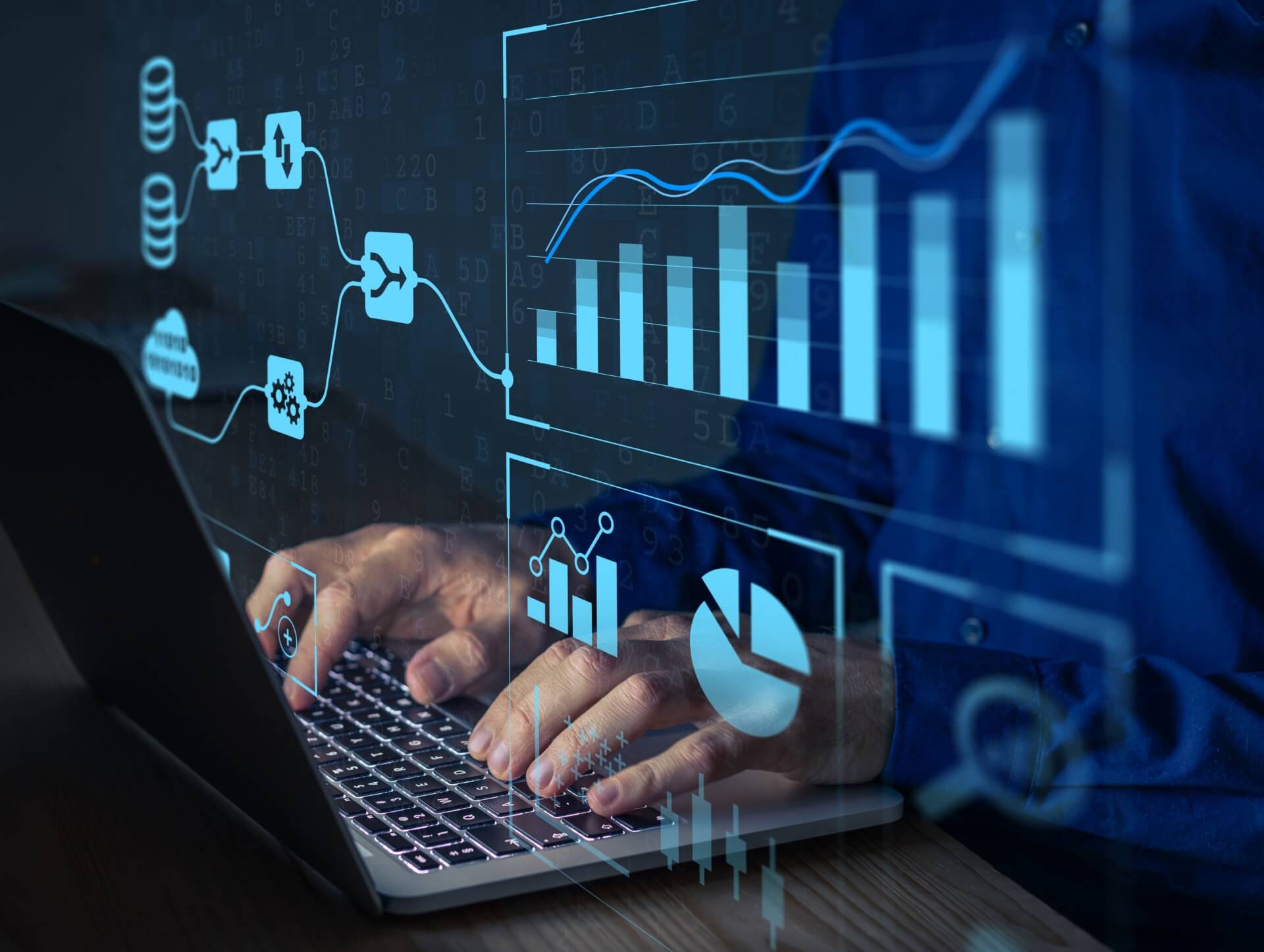
06/09/2023
The Importance of Master Data Modeling
Master Data Management (MDM) is a critical process for organizations looking to ensure data accuracy, consistency, and integrity. One of the key components of MDM is master data modeling, which involves the creation and management of data models to define and represent the organization's master data entities, attributes, and relationships.
Master data modeling provides a structured approach to organizing and representing the organization's master data, allowing for better governance, improved data quality, and enhanced decision-making. It helps in defining the data elements, their relationships, and the rules that govern their usage.
With regulatory compliance becoming increasingly important in today's business landscape, master data modeling plays a crucial role in ensuring that organizations meet the necessary regulatory requirements. It enables organizations to establish a strong foundation for data governance, data quality management, and data security throughout the data lifecycle.
The Benefits of Master Data Modeling
Master data modeling offers several benefits for organizations seeking regulatory compliance:
- Improved Data Governance: Master data modeling provides a clear and consistent view of the organization's data assets, enabling effective data governance. It helps in establishing data ownership, defining data stewardship roles, and enforcing data policies and standards.
- Enhanced Data Quality: By defining data elements, relationships, and validation rules, master data modeling helps in improving data quality. It ensures that the organization's data is accurate, complete, and consistent across different systems and processes.
- Better Decision-Making: With a well-designed master data model, organizations can gain a holistic view of their data, enabling better decision-making. It allows for data analysis and reporting, leading to actionable insights and informed business decisions.
- Increased Efficiency: Master data modeling streamlines data integration and data management processes, leading to increased operational efficiency. It enables seamless data exchange between different systems and applications, reducing data duplication and redundancy.
Master Data Modeling Best Practices
To effectively leverage master data modeling for regulatory compliance, organizations should follow the following best practices:
- Understand the Business Requirements: Before starting the master data modeling process, it is crucial to understand the organization's business requirements and data needs. This involves engaging with business stakeholders, subject matter experts, and data governance teams to identify the key data entities, attributes, and relationships.
- Define a Clear Data Governance Framework: Establishing a robust data governance framework is essential for successful master data modeling. It involves defining data stewardship roles, responsibilities, and processes, as well as implementing data policies and standards to ensure data integrity and compliance.
- Utilize Industry Standards and Best Practices: To ensure interoperability and compatibility with external systems, organizations should leverage industry standards and best practices in master data modeling. This includes utilizing standard data models, data integration protocols, and data exchange formats.
- Implement Data Quality Management: Data quality management is a critical aspect of master data modeling. Organizations should implement data cleansing, data validation, and data enrichment processes to ensure that the master data is accurate, complete, and consistent.
- Consider Scalability and Flexibility: When designing the master data model, organizations should consider future scalability and flexibility requirements. The model should be able to accommodate new data elements, relationships, and business rules as the organization grows and evolves.
- Ensure Data Security and Privacy: Master data modeling should incorporate data security and privacy considerations. This involves implementing access controls, encryption, and anonymization techniques to protect sensitive data and comply with data protection regulations.
Challenges in Master Data Modeling for Regulatory Compliance
While master data modeling offers numerous benefits for regulatory compliance, organizations may face certain challenges during the process:
- Data Complexity: Organizations often deal with large volumes of complex data, making it challenging to define and represent the data accurately in the master data model.
- Data Integration: Integrating master data from multiple sources and systems can be complex, requiring organizations to establish data integration processes and protocols.
- Data Quality: Ensuring data quality is an ongoing challenge in master data modeling. It requires continuous monitoring, cleansing, and enrichment of the master data to maintain its accuracy and consistency.
- Organizational Alignment: Master data modeling requires collaboration and alignment across different business units and departments. Organizations may face challenges in establishing a common understanding and agreement on data definitions and relationships.
- Technology Adoption: Implementing a master data management platform and supporting technologies can be complex and requires careful planning and execution.
Case Studies: Successful Master Data Modeling Implementations
Several organizations have successfully implemented master data modeling for regulatory compliance. Here are a few case studies:
Case Study 1: Company X
Company X, a global manufacturing company, implemented a master data modeling solution to ensure compliance with industry regulations. By defining a comprehensive master data model, they were able to establish data governance processes, improve data quality, and enable seamless data integration across their global operations. This helped them streamline their regulatory reporting processes and avoid penalties for non-compliance.
Case Study 2: Company Y
Company Y, a leading healthcare provider, implemented a cloud-based master data management platform to enhance regulatory compliance. The master data model enabled them to consolidate and manage patient data from multiple sources, ensuring data accuracy and privacy. By leveraging the master data model, they were able to improve patient care, streamline billing processes, and comply with healthcare regulations.
Case Study 3: Company Z
Company Z, a financial services organization, implemented an open-source master data management solution to address their regulatory compliance challenges. The master data model helped them establish a single source of truth for customer data, enabling better risk management and regulatory reporting. The solution also allowed them to integrate data from multiple systems, improving operational efficiency and reducing compliance costs.
Conclusion
Master data modeling plays a crucial role in ensuring regulatory compliance for organizations. By defining and managing the organization's master data entities, attributes, and relationships, it provides a foundation for data governance, data quality management, and data security throughout the data lifecycle. With the right master data modeling strategy and best practices, organizations can achieve improved data governance, enhanced data quality, better decision-making, and increased operational efficiency. While there may be challenges in the process, successful implementations of master data modeling have demonstrated significant benefits for organizations across various industries.
Contact us
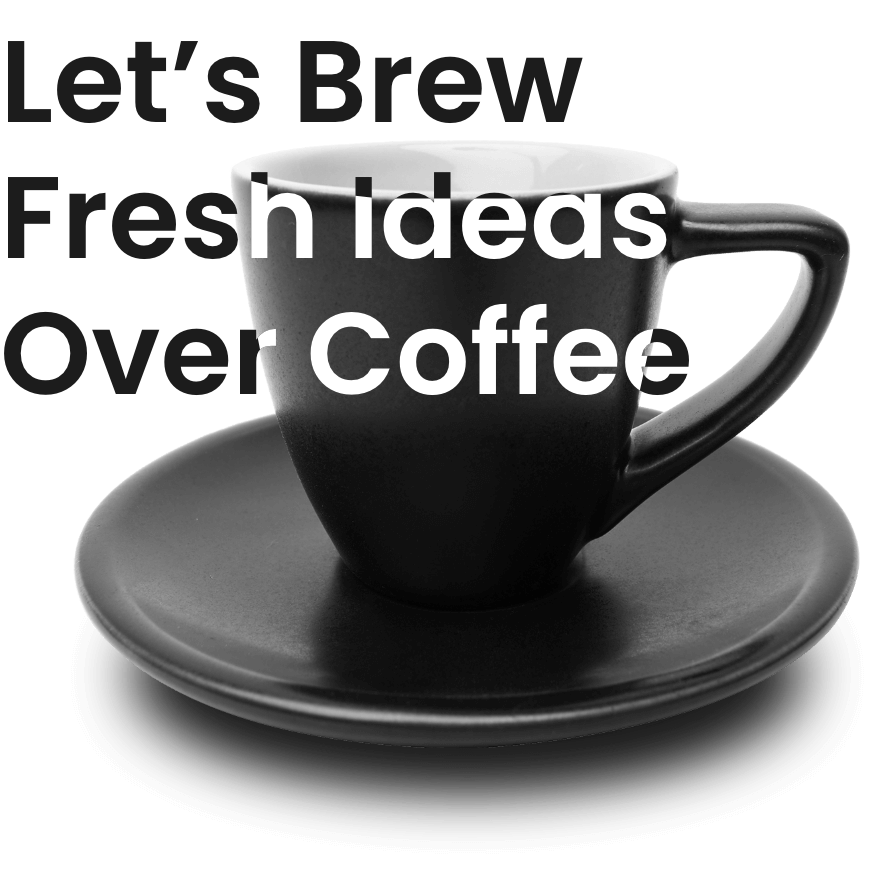
Spanning 8 cities worldwide and with partners in 100 more, we’re your local yet global agency.
Fancy a coffee, virtual or physical? It’s on us – let’s connect!