MDM best practices for managing asset data
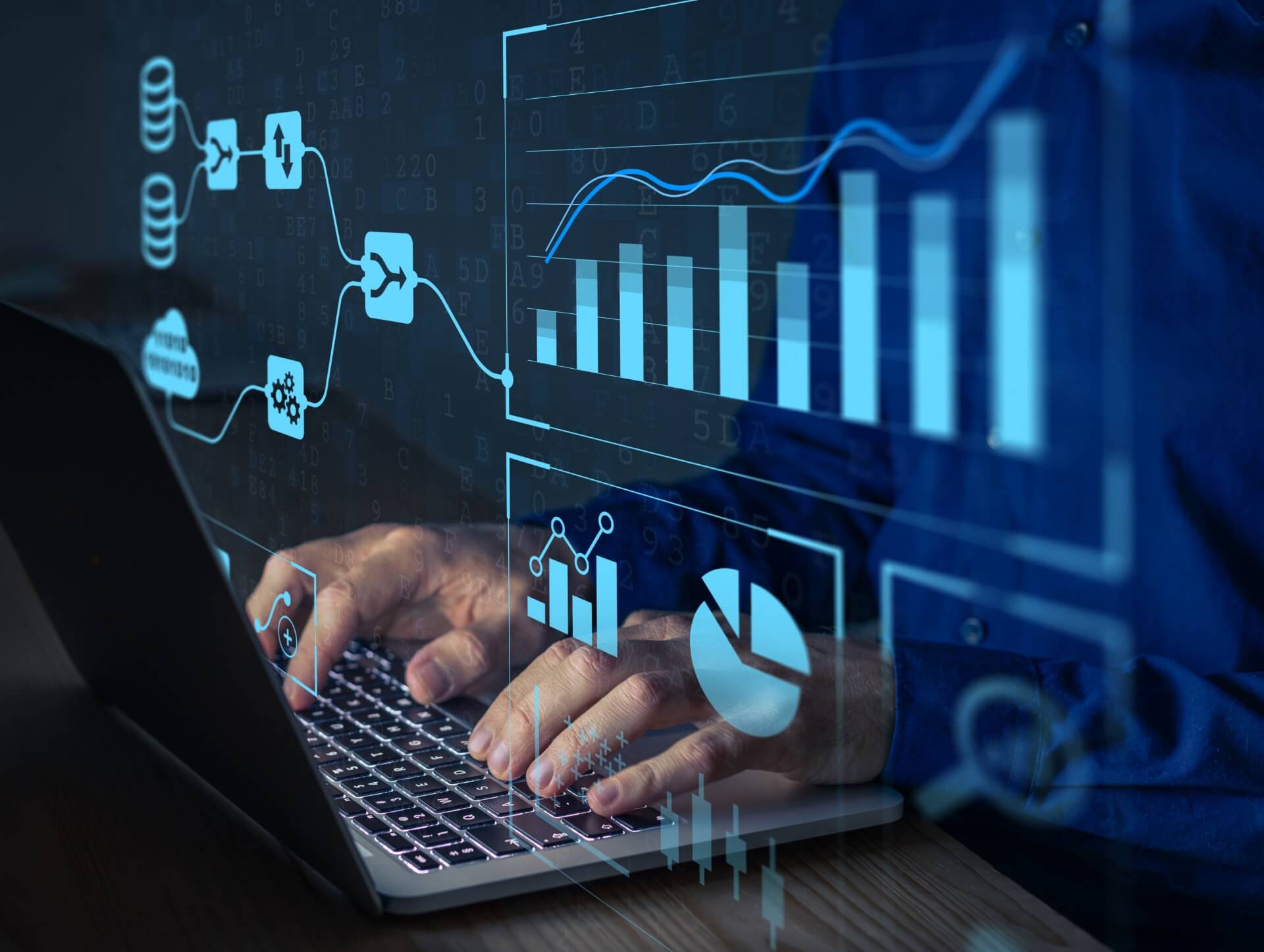
06/09/2023
Master Data Management (MDM) is a comprehensive approach to managing and maintaining an organization's critical data assets. It involves the processes, policies, and technologies required to create and maintain a single, accurate, and consistent version of data across various systems and applications.
The Importance of MDM
Effective MDM is crucial for businesses of all sizes and industries. It provides a solid foundation for data-driven decision-making, enhances operational efficiency, improves customer experience, and ensures compliance with regulatory requirements. By adopting MDM best practices, organizations can unlock the full potential of their data and gain a competitive edge in the market.
Benefits of Master Data Management
Implementing a robust MDM strategy offers several benefits to organizations:
- Improved Data Quality: MDM helps to eliminate data inconsistencies, duplicates, and inaccuracies, ensuring that organizations have access to reliable and accurate data.
- Enhanced Decision-Making: By providing a single, unified view of data, MDM enables organizations to make informed decisions based on reliable and up-to-date information.
- Streamlined Operations: MDM facilitates seamless data integration and data sharing across different systems and applications, reducing operational inefficiencies and improving productivity.
- Regulatory Compliance: MDM ensures that organizations comply with data privacy regulations and other legal requirements, minimizing the risk of non-compliance and associated penalties.
- Improved Customer Experience: By maintaining accurate and consistent customer data, MDM enables organizations to deliver personalized and targeted experiences to their customers, leading to increased customer satisfaction and loyalty.
MDM Best Practices
Implementing MDM effectively requires following certain best practices:
Data Governance in MDM
Data governance plays a critical role in the success of MDM initiatives. It involves defining clear roles, responsibilities, and processes for managing data, ensuring data quality, and enforcing data standards and policies. Organizations should establish a data governance framework that aligns with their business objectives and enables effective collaboration between business and IT stakeholders.
Data Quality Management
Ensuring data quality is essential for MDM success. Organizations should implement data quality management processes and tools to identify and resolve data issues, such as duplicates, inconsistencies, and inaccuracies. Data cleansing, validation, and enrichment techniques should be employed to improve data quality and reliability.
MDM Strategy for Businesses
Organizations should develop a comprehensive MDM strategy that aligns with their business goals and objectives. The strategy should define the scope of MDM implementation, identify the critical data domains to be managed, and outline the roadmap for implementation. A well-defined strategy helps organizations prioritize MDM initiatives and allocate resources effectively.
MDM Implementation Process
MDM implementation should follow a structured and phased approach. It involves activities such as data profiling, data modeling, data integration, data migration, and data governance. Organizations should establish a dedicated MDM team, comprising business and IT stakeholders, to oversee the implementation process and ensure its successful execution.
Master Data Governance
Master data governance involves establishing policies, procedures, and controls for managing master data throughout its lifecycle. It includes activities such as data stewardship, data ownership, data quality monitoring, and data lifecycle management. By implementing effective master data governance practices, organizations can ensure the accuracy, consistency, and reliability of master data.
MDM Tools and Technologies
There are various MDM tools and technologies available in the market to support MDM initiatives. These tools provide functionalities such as data integration, data cleansing, data matching, data quality management, and data governance. Organizations should carefully evaluate and select the right set of tools that align with their MDM requirements and objectives.
Data Integration in MDM
Data integration is a critical component of MDM. It involves integrating data from various sources and systems to create a unified and consistent view of data. Organizations should adopt data integration techniques such as Extract, Transform, Load (ETL), and Application Programming Interfaces (APIs) to establish seamless data flow and synchronization between different systems.
MDM for Enterprise Scalability
MDM should be designed and implemented to scale with the growing needs of the organization. It should be able to handle large volumes of data, accommodate new data sources and systems, and support the evolving business requirements. Organizations should consider factors such as data volume, data velocity, and data variety while designing their MDM architecture.
Master Data Modeling
Master data modeling involves designing the structure and relationships of master data entities. It defines the attributes, hierarchies, and classifications of master data, enabling organizations to manage and organize data effectively. Organizations should adopt industry-standard data modeling techniques such as Entity-Relationship (ER) modeling and Unified Modeling Language (UML) to design their master data model.
MDM and Data Security
Data security is a critical aspect of MDM. Organizations should implement robust security measures to protect master data from unauthorized access, manipulation, and breaches. This includes access controls, encryption, data masking, and regular security audits. Data security should be integrated into the overall MDM architecture and processes.
MDM and Data Analytics
MDM can significantly enhance the effectiveness of data analytics initiatives. By providing accurate and consistent data, MDM enables organizations to perform reliable analysis and gain valuable insights. Integrated MDM and data analytics solutions can help organizations make data-driven decisions, identify trends and patterns, and uncover hidden opportunities.
MDM and Customer Experience
MDM has a direct impact on customer experience. By maintaining accurate and up-to-date customer data, organizations can deliver personalized and targeted experiences to their customers. This includes personalized marketing campaigns, tailored product recommendations, and proactive customer support. MDM enables organizations to understand their customers better and build strong, long-lasting relationships.
Challenges in MDM
Implementing MDM can be challenging due to various factors:
- Data Complexity: Organizations often deal with large volumes of complex data, making it challenging to manage and maintain master data effectively.
- Data Silos: Data silos and fragmented systems hinder the consolidation and integration of data, leading to data inconsistencies and duplication.
- Data Ownership: Determining data ownership and accountability can be challenging, especially in large organizations with multiple business units and departments.
- Change Management: MDM implementation involves significant changes to business processes and systems, which may face resistance from employees and stakeholders.
- Technical Complexity: Implementing MDM requires expertise in data integration, data modeling, and data management technologies, which can be complex and require specialized skills.
MDM Case Studies
Several organizations have successfully implemented MDM solutions to address their data management challenges:
- Company A: Company A, a global retail chain, implemented an MDM solution to consolidate customer data from various sales channels, enabling personalized marketing campaigns and improved customer service.
- Company B: Company B, a healthcare provider, implemented an MDM platform to manage patient data across multiple systems, ensuring data accuracy and compliance with healthcare regulations.
- Company C: Company C, a financial services firm, implemented an MDM solution to consolidate and reconcile customer account data from different business units, enabling a unified view of customer relationships and improved cross-selling opportunities.
MDM in Multi-Cloud Environments
In today's digital landscape, organizations often operate in multi-cloud environments, with data spread across various cloud platforms and services. MDM can help organizations manage and synchronize data across multiple clouds, ensuring data consistency and accuracy. Organizations should consider MDM solutions that support multi-cloud integration and data governance.
MDM ROI and Value
MDM initiatives can deliver significant return on investment (ROI) and business value. By improving data quality, streamlining operations, and enabling data-driven decision-making, organizations can achieve cost savings, increased revenue, improved customer satisfaction, and reduced compliance risks. It is essential to measure and track the ROI of MDM initiatives to demonstrate their value to the organization.
Data Governance in Lifecycle Management
Data governance is a critical component of data lifecycle management. It involves defining policies, processes, and controls for managing data throughout its lifecycle, from creation to archival. Data governance ensures that data is accurate, consistent, and compliant with regulatory requirements at each stage of the data lifecycle.
Data Security throughout the Lifecycle
Data security should be a top priority throughout the data lifecycle. Organizations should implement security measures such as encryption, access controls, and data masking to protect data from unauthorized access and breaches. Regular security audits and vulnerability assessments should be conducted to identify and mitigate security risks.
Data Compliance in Management
Effective data management should ensure compliance with relevant data privacy regulations and industry standards. Organizations should establish data governance policies and procedures that address data compliance requirements and regularly monitor and audit data management practices to ensure compliance.
Master Data in Lifecycle Management
Master data plays a crucial role in data lifecycle management. It provides a consistent and reliable foundation for data integration, analysis, and decision-making throughout the data lifecycle. Organizations should focus on managing and governing master data effectively to ensure the accuracy and integrity of data across its lifecycle.
Metadata Management in Data Lifecycle
Metadata management is essential for effective data lifecycle management. It involves capturing and managing metadata, such as data definitions, data lineage, and data quality rules, throughout the data lifecycle. Metadata provides context and insights into data, enabling organizations to understand and use data effectively.
Automation in Data Lifecycle
Automation plays a crucial role in optimizing data lifecycle management processes. Organizations should leverage automation technologies such as robotic process automation (RPA) and artificial intelligence (AI) to streamline data management activities, reduce manual effort, and improve efficiency and accuracy.
Cloud-Based Data Lifecycle
Cloud computing offers several advantages for data lifecycle management. It provides scalability, flexibility, and cost-effectiveness, enabling organizations to store, process, and analyze large volumes of data. Cloud-based data lifecycle management solutions offer centralized data storage, seamless data integration, and advanced analytics capabilities.
Analytics in Data Lifecycle
Analytics plays a crucial role in extracting insights and value from data throughout its lifecycle. Organizations should leverage analytics tools and techniques to analyze data at each stage of the data lifecycle, from data acquisition to data archival. By applying analytics, organizations can uncover hidden patterns, trends, and opportunities.
Challenges in Data Lifecycle Management
Data lifecycle management can face several challenges:
- Data Volume: Managing large volumes of data can be challenging, especially with the exponential growth of data in today's digital world.
- Data Variety: Organizations deal with diverse data types, such as structured, unstructured, and semi-structured data, which require different management approaches.
- Data Velocity: The speed at which data is generated and processed poses challenges in capturing, storing, and analyzing data in real-time.
- Data Governance: Ensuring data governance throughout the data lifecycle can be complex, requiring clear policies, processes, and controls.
- Data Privacy: Organizations must comply with data privacy regulations and protect sensitive data throughout the data lifecycle.
Best Practices for Lifecycle Management
To effectively manage the data lifecycle, organizations should follow best practices:
- Define Clear Data Lifecycle Processes: Establish clear processes and workflows for data acquisition, storage, processing, analysis, and archival.
- Implement Data Governance: Establish data governance policies and procedures to ensure data accuracy, consistency, and compliance throughout the data lifecycle.
- Adopt Automation: Leverage automation technologies to streamline data management processes, reduce manual effort, and improve efficiency.
- Establish Data Security Measures: Implement robust data security measures to protect data from unauthorized access, breaches, and cyber threats.
- Leverage Analytics: Apply analytics tools and techniques to extract insights and value from data at each stage of the data lifecycle.
ROI of Effective Data Lifecycle
Effective data lifecycle management can deliver significant ROI and business value:
- Cost Savings: By optimizing data storage, processing, and management, organizations can achieve cost savings in hardware, software, and operational expenses.
- Improved Efficiency: Streamlining data management processes and workflows improves operational efficiency and reduces manual effort.
- Enhanced Decision-Making: By providing timely and accurate data, organizations can make informed decisions, leading to improved business outcomes.
- Compliance Risk Reduction: Effective data lifecycle management ensures compliance with data privacy regulations and reduces the risk of non-compliance and associated penalties.
- Business Insights: By leveraging analytics throughout the data lifecycle, organizations can gain valuable insights and uncover hidden opportunities.
Contact us
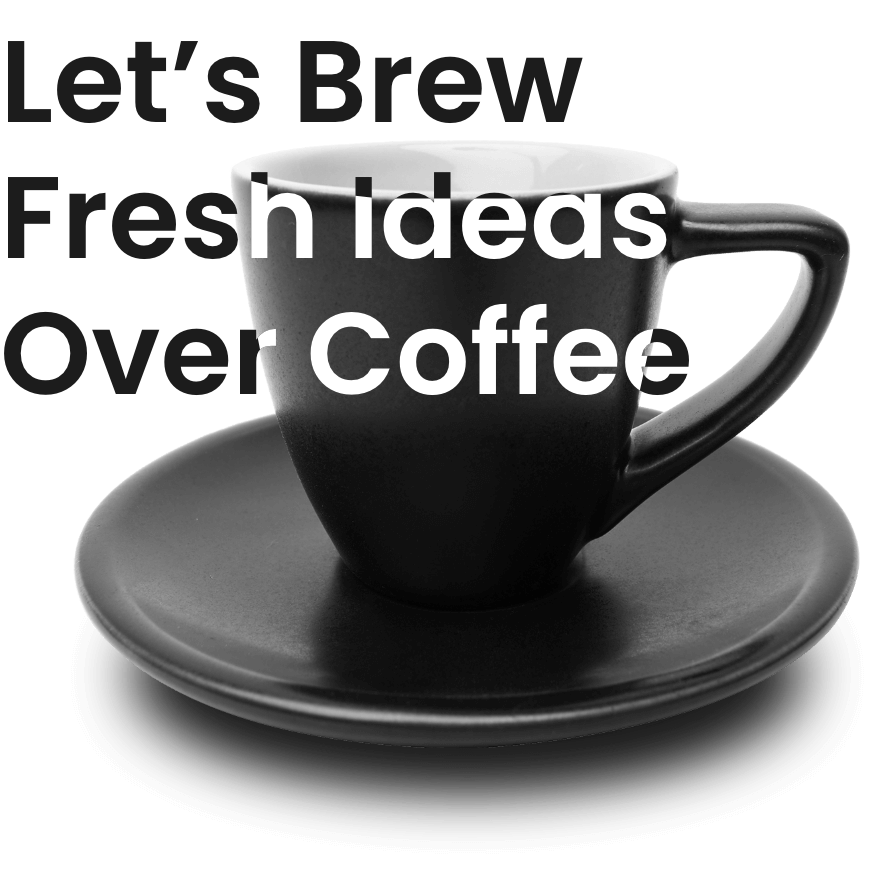
Spanning 8 cities worldwide and with partners in 100 more, we’re your local yet global agency.
Fancy a coffee, virtual or physical? It’s on us – let’s connect!