MDM best practices for managing financial data
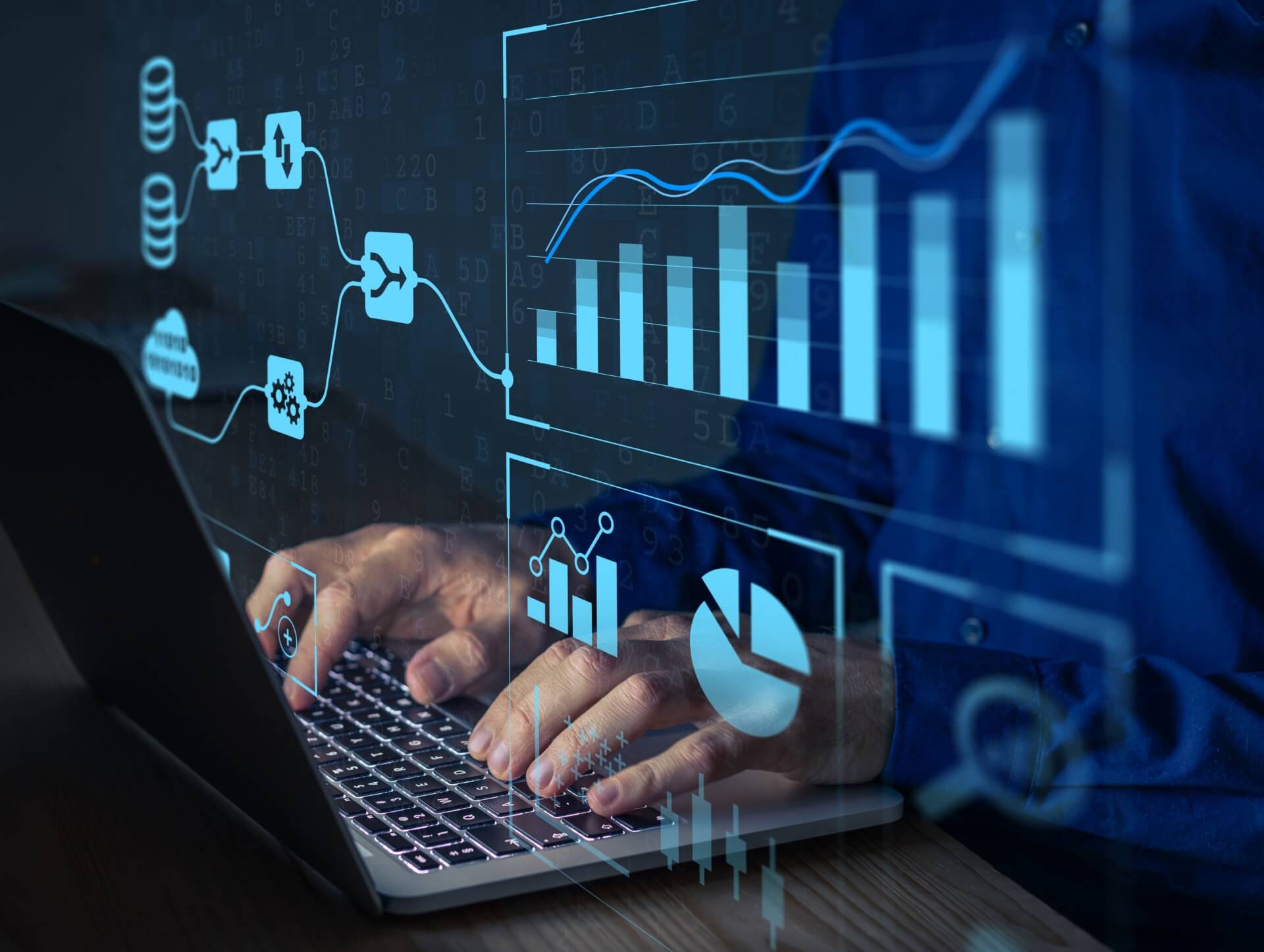
06/09/2023
Master Data Management (MDM) is a comprehensive approach to managing and integrating an organization's critical data assets. In the financial industry, where data accuracy and consistency are crucial, MDM plays a vital role in ensuring data quality, governance, and security. In this article, we will explore the best practices for managing financial data using MDM solutions and strategies.
The Benefits of MDM in Financial Data Management
Implementing MDM in financial data management brings numerous benefits to organizations:
- Improved Data Quality: MDM ensures that data is accurate, consistent, and up-to-date, enabling better decision-making and reducing the risk of errors.
- Enhanced Data Governance: MDM provides a framework for defining data ownership, accountability, and stewardship, ensuring compliance with regulations and industry standards.
- Increased Data Security: MDM helps organizations establish data security policies, access controls, and encryption methods, protecting sensitive financial information from unauthorized access or breaches.
- Better Data Integration: MDM enables seamless integration of data from various systems and sources, eliminating data silos and improving data accessibility and usability.
- Streamlined Business Processes: MDM enables organizations to automate data-related processes, such as customer onboarding, KYC (Know Your Customer) compliance, and financial reporting, improving operational efficiency and reducing costs.
MDM Best Practices for Managing Financial Data
When implementing MDM for financial data management, organizations should follow these best practices:
Data Governance in MDM
Data governance is a critical component of MDM, especially in the financial sector where data compliance and regulatory requirements are stringent. Organizations should establish a data governance framework that includes:
- Clearly defined data ownership and accountability
- Standardized data definitions and business rules
- Data quality monitoring and measurement
- Data stewardship and data governance roles
- Regular data audits and compliance assessments
Data Quality Management
Ensuring data quality is essential for accurate financial reporting and analysis. Organizations should implement data quality management practices, including:
- Data profiling and cleansing to identify and correct data inconsistencies
- Data validation and verification to ensure data accuracy
- Data enrichment to enhance data completeness and relevance
- Data deduplication to eliminate duplicate records
- Data integration and reconciliation to maintain data consistency across systems
MDM Strategy for Businesses
Developing a comprehensive MDM strategy is crucial for successful financial data management. Organizations should consider the following when formulating their MDM strategy:
- Defining clear business objectives and goals
- Identifying key data domains and entities
- Creating a roadmap for MDM implementation
- Establishing data governance and stewardship processes
- Choosing the right MDM tools and technologies
- Ensuring data security and compliance
- Measuring and monitoring MDM performance and ROI
MDM Implementation Process
Implementing MDM for financial data management requires a structured approach. Organizations should follow these steps:
- Assess the current state of data management and identify pain points
- Define the desired future state and set realistic goals
- Design the MDM architecture and data model
- Choose the appropriate MDM solution and technology stack
- Implement the MDM solution, ensuring data integration and migration
- Establish data governance and stewardship processes
- Train and educate employees on MDM best practices
- Monitor and measure MDM performance and make necessary improvements
Master Data Governance
Master data governance is essential for maintaining the integrity and quality of financial data. Organizations should establish master data governance practices, including:
- Defining data ownership and accountability for master data
- Establishing data stewardship roles and responsibilities
- Implementing data validation and verification processes
- Ensuring data completeness and accuracy
- Monitoring and measuring master data quality
MDM Tools and Technologies
Choosing the right MDM tools and technologies is critical for effective financial data management. Organizations should consider the following factors when selecting MDM solutions:
- Scalability and performance
- Data integration capabilities
- Data security features
- User-friendly interface
- Compatibility with existing systems
- Flexibility and customization options
- Vendor reputation and support
Data Integration in MDM
Data integration is a key component of MDM, enabling organizations to consolidate and harmonize data from various sources. Organizations should consider the following when implementing data integration in MDM:
- Identify data integration requirements
- Design data integration workflows and processes
- Implement data integration tools and technologies
- Ensure data mapping and transformation
- Validate and reconcile integrated data
- Monitor and measure data integration performance
MDM for Enterprise Scalability
Organizations should design their MDM architecture and solutions to scale with their growing business needs. Consider the following when implementing MDM for enterprise scalability:
- Design a flexible and scalable MDM architecture
- Choose MDM solutions that can handle large volumes of data
- Implement data partitioning and clustering techniques
- Ensure high availability and fault tolerance
- Regularly monitor and optimize MDM performance
MDM and Data Security
Data security is a top priority in financial data management. Organizations should implement the following measures to ensure data security in MDM:
- Establish data security policies and procedures
- Implement access controls and user authentication
- Encrypt sensitive financial data
- Regularly audit and monitor data access and usage
- Implement data backup and disaster recovery plans
- Keep software and systems up-to-date with security patches
MDM and Data Analytics
MDM plays a crucial role in enabling data analytics in financial organizations. By providing clean, consistent, and integrated data, MDM enables accurate and insightful data analytics. Organizations should consider the following when leveraging MDM for data analytics:
- Integrate MDM with data analytics platforms and tools
- Ensure data quality and accuracy for reliable analytics results
- Facilitate self-service analytics for business users
- Establish data governance and data stewardship processes for analytics
- Monitor and measure the impact of data analytics on business outcomes
MDM and Customer Experience
MDM plays a crucial role in enhancing the customer experience in the financial industry. By providing a single, accurate, and complete view of customer data, MDM enables personalized and targeted customer interactions. Organizations should consider the following when leveraging MDM for customer experience:
- Consolidate customer data from various systems and channels
- Eliminate duplicate customer records
- Enrich customer data with additional attributes and insights
- Enable real-time access to customer data for customer service representatives
- Personalize customer interactions based on accurate customer data
Challenges in MDM
Implementing MDM for financial data management comes with its share of challenges. Some common challenges include:
- Data complexity and heterogeneity
- Data quality issues
- Data integration and consolidation
- Data governance and compliance
- Resistance to change from employees
- Legacy system integration
- High implementation and maintenance costs
MDM Case Studies
Several organizations have successfully implemented MDM for financial data management. Let's look at some case studies:
- Case Study 1: Company A implemented an MDM solution to consolidate customer data from multiple systems. This resulted in improved data quality, enhanced customer segmentation, and increased cross-selling opportunities.
- Case Study 2: Company B implemented MDM to streamline their financial reporting process. This resulted in reduced reporting errors, improved regulatory compliance, and faster financial close cycles.
- Case Study 3: Company C implemented MDM to improve data governance and data security. This resulted in enhanced data privacy, reduced data breaches, and improved customer trust.
MDM in Multi-Cloud Environments
As organizations increasingly adopt multi-cloud environments, managing data across multiple cloud platforms becomes a challenge. MDM can help organizations achieve consistent and integrated data across multi-cloud environments. Organizations should consider the following when implementing MDM in multi-cloud environments:
- Choose MDM solutions that support multi-cloud integration
- Ensure data security and compliance across cloud platforms
- Implement data integration and synchronization processes
- Establish data governance and stewardship processes
- Monitor and measure data quality and performance across cloud platforms
MDM ROI and Value
Implementing MDM for financial data management can deliver significant ROI and business value. Organizations can realize the following benefits:
- Cost savings from improved operational efficiency
- Reduced errors and rework
- Enhanced regulatory compliance
- Better decision-making based on accurate and reliable data
- Improved customer satisfaction and loyalty
- Increased cross-selling and upselling opportunities
- Competitive advantage in the market
- Improved data security and reduced data breaches
Data Governance in Lifecycle Management
Data governance is essential throughout the data lifecycle, from data creation to data retirement. Organizations should establish data governance practices that span the entire data lifecycle, including:
- Defining data ownership and accountability at each stage of the lifecycle
- Implementing data quality monitoring and measurement throughout the lifecycle
- Ensuring data security and compliance at each stage
- Establishing data retention and disposal policies
- Regularly auditing and assessing data governance practices
Data Security Throughout the Lifecycle
Data security should be a top priority throughout the data lifecycle. Organizations should implement the following measures to ensure data security:
- Encrypt sensitive data at rest and in transit
- Implement access controls and user authentication mechanisms
- Regularly update and patch software and systems
- Monitor and log data access and usage
- Implement data backup and disaster recovery plans
- Train employees on data security best practices
Data Compliance in Management
Data compliance is crucial in financial data management. Organizations should ensure compliance with relevant regulations and industry standards, including:
- General Data Protection Regulation (GDPR)
- Sarbanes-Oxley Act (SOX)
- Payment Card Industry Data Security Standard (PCI DSS)
- Financial Industry Regulatory Authority (FINRA) regulations
- Consumer Financial Protection Bureau (CFPB) regulations
Master Data in Lifecycle Management
Master data is a critical component of the data lifecycle. Organizations should manage master data throughout its lifecycle, including:
- Creating and capturing accurate and complete master data
- Integrating master data with other data sources
- Ensuring data quality and consistency for master data
- Updating and maintaining master data as business needs evolve
- Retiring and disposing of master data in compliance with regulations
Metadata Management in Data Lifecycle
Metadata management is crucial for effective data lifecycle management. Organizations should implement metadata management practices, including:
- Defining and documenting metadata standards and definitions
- Establishing metadata governance processes and roles
- Capturing and managing metadata throughout the data lifecycle
- Linking metadata to data assets for better data lineage and traceability
- Regularly auditing and assessing metadata quality and accuracy
Automation in Data Lifecycle
Automation plays a significant role in streamlining the data lifecycle management process. Organizations should leverage automation to:
- Automate data capture and ingestion processes
- Automate data integration and transformation workflows
- Automate data quality monitoring and measurement
- Automate data backup and disaster recovery processes
- Automate data retention and disposal processes
- Automate metadata management and documentation
Cloud-Based Data Lifecycle
Cloud-based data lifecycle management offers several benefits, including scalability, flexibility, and cost-efficiency. Organizations should consider the following when implementing cloud-based data lifecycle management:
- Choose a reliable and secure cloud provider
- Ensure data security and compliance in the cloud
- Implement data integration and synchronization with on-premises systems
- Leverage cloud-based data management tools and technologies
- Monitor and measure cloud-based data lifecycle performance
Analytics in Data Lifecycle
Data analytics is an essential component of the data lifecycle. Organizations should leverage analytics to gain insights and drive business value throughout the data lifecycle. Consider the following when implementing analytics in data lifecycle management:
- Integrate analytics tools and technologies with data management platforms
- Ensure data quality and accuracy for reliable analytics results
- Facilitate self-service analytics for business users
- Establish data governance and data stewardship processes for analytics
- Monitor and measure the impact of data analytics on business outcomes
Challenges in Data Lifecycle Management
Data lifecycle management comes with its share of challenges. Some common challenges include:
- Data volume and complexity
- Data quality issues
- Data integration and consolidation
- Data security and compliance
- Legacy system integration
- High implementation and maintenance costs
- Resistance to change from employees
Best Practices for Lifecycle Management
Implementing effective data lifecycle management requires following best practices, including:
- Establishing clear data governance processes and roles
- Ensuring data quality and accuracy throughout the lifecycle
- Implementing data security and compliance measures
- Automating data management processes
- Integrating analytics throughout the data lifecycle
- Regularly monitoring and measuring data lifecycle performance
ROI of Effective Data Lifecycle
Implementing effective data lifecycle management can deliver significant ROI and business value. Organizations can realize the following benefits:
- Cost savings from improved operational efficiency
- Reduced errors and rework
- Enhanced regulatory compliance
- Better decision-making based on accurate and reliable data
- Improved customer satisfaction and loyalty
- Increased cross-selling and upselling opportunities
- Competitive advantage in the market
- Improved data security and reduced data breaches
Contact us
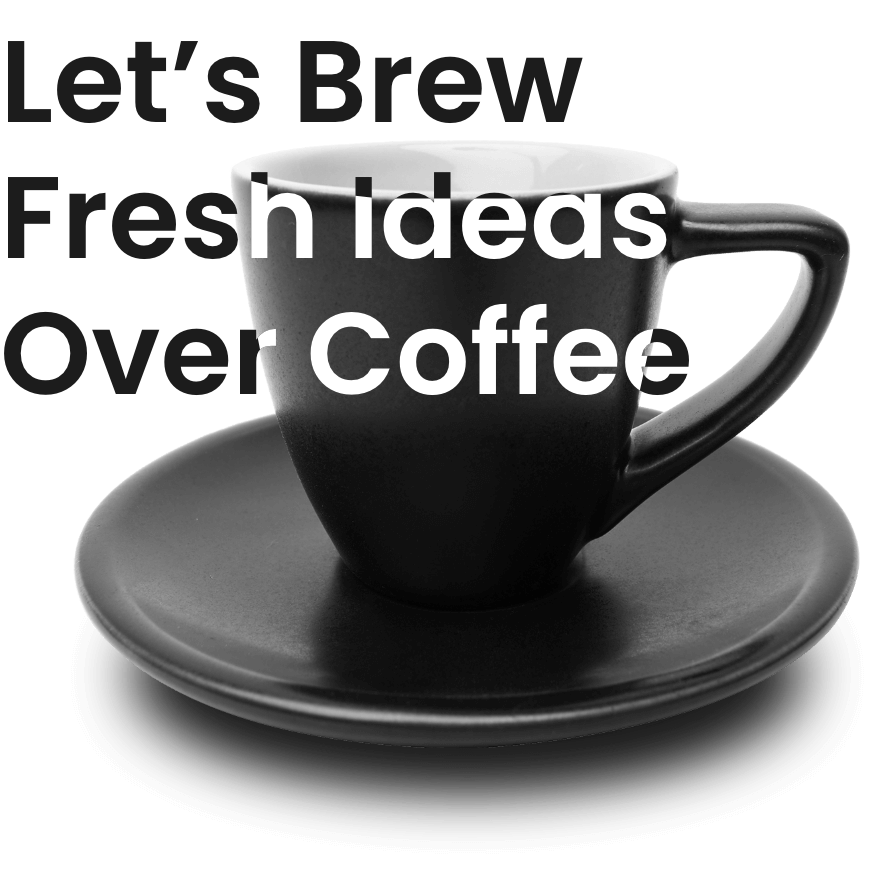
Spanning 8 cities worldwide and with partners in 100 more, we’re your local yet global agency.
Fancy a coffee, virtual or physical? It’s on us – let’s connect!