Overcoming challenges in MDM implementation
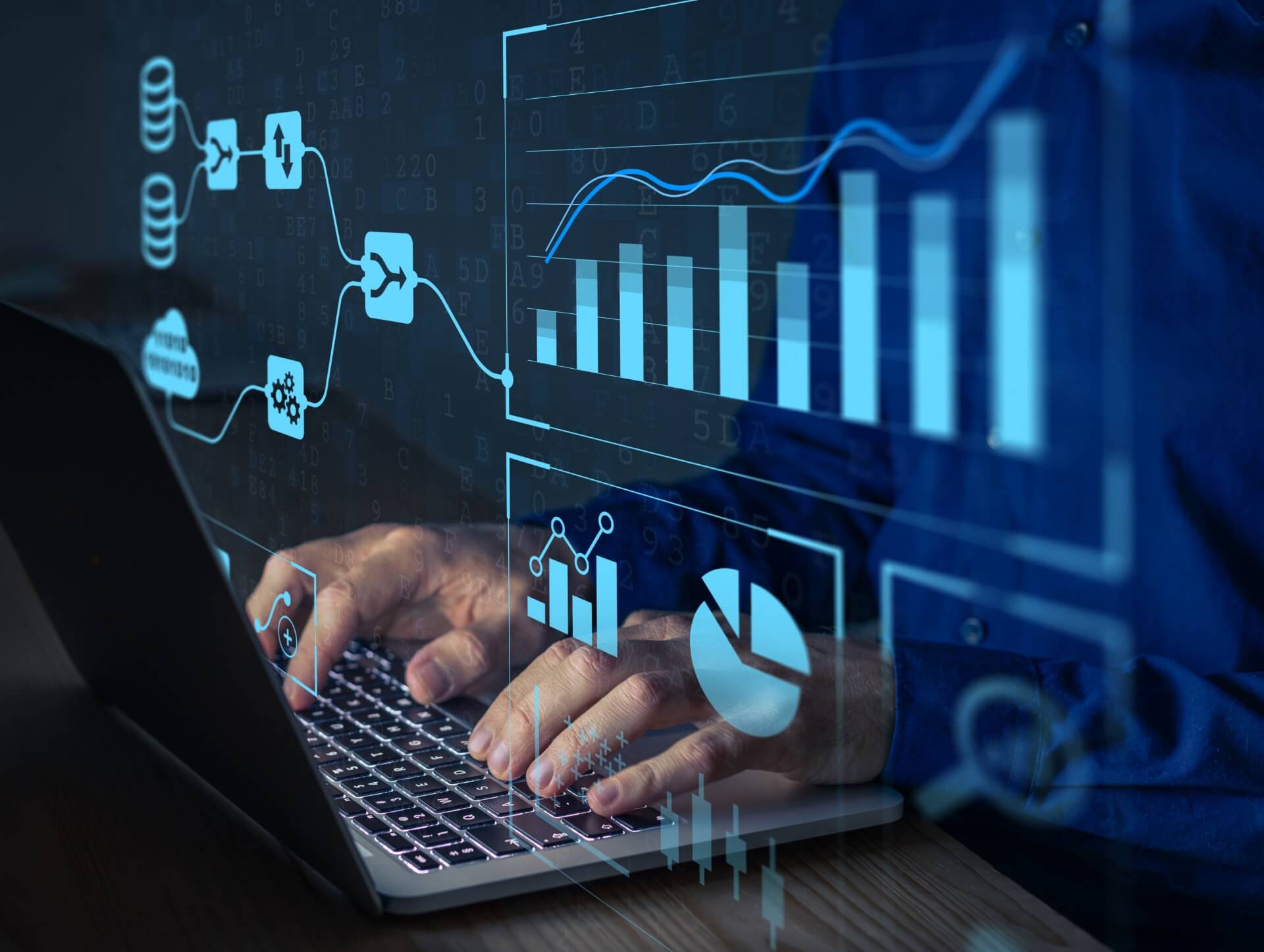
06/09/2023
Master Data Management (MDM) is a critical process for organizations to effectively manage and maintain their master data. It involves the consolidation, cleansing, and governance of master data across various systems and departments within an organization. Implementing MDM can bring numerous benefits, such as improved data quality, increased operational efficiency, enhanced decision-making, and better compliance with regulations.
However, implementing MDM can also be a complex and challenging endeavor. In this article, we will explore the common challenges faced during MDM implementation and provide strategies to overcome them.
1. Developing a Comprehensive MDM Strategy
A successful MDM implementation starts with a well-defined strategy. It is essential to have a clear understanding of your organization's goals, objectives, and requirements before embarking on an MDM initiative. This involves defining the scope of the project, identifying the key stakeholders, and establishing a governance structure.
To develop a comprehensive MDM strategy, consider the following:
- Define the business objectives: Identify the specific business objectives that the MDM implementation aims to achieve. This could include improving data quality, streamlining business processes, or enabling better analytics.
- Assess the current state: Evaluate the current state of your organization's data management practices, systems, and processes. Identify any gaps or areas for improvement.
- Define the target state: Determine the desired future state of your organization's data management. This includes defining the ideal data architecture, governance framework, and data quality standards.
- Establish a governance structure: Define the roles, responsibilities, and decision-making processes for managing master data. This includes establishing a data governance council and assigning data stewards.
- Develop a roadmap: Create a detailed plan that outlines the specific activities, timelines, and resources required for implementing MDM. Prioritize the activities based on their importance and feasibility.
2. Ensuring Data Quality Management
Data quality is a critical aspect of MDM. Poor data quality can lead to inaccurate insights, inefficient processes, and compliance issues. To ensure data quality, organizations need to implement robust data quality management processes and tools.
Here are some best practices for data quality management in MDM:
- Data profiling: Perform data profiling to assess the quality of your existing data. Identify any data anomalies, inconsistencies, or duplicates.
- Data cleansing: Implement data cleansing processes to correct or remove any inaccuracies, inconsistencies, or duplicates in your data. This could involve standardizing data formats, validating data against predefined rules, or merging duplicate records.
- Data enrichment: Enhance your data by enriching it with additional attributes or information from external sources. This can improve the accuracy and completeness of your master data.
- Data monitoring: Continuously monitor your data quality to identify and address any issues or anomalies in real-time. Implement data quality dashboards and alerts to proactively manage data quality.
- Data governance: Establish data governance processes and policies to ensure ongoing data quality management. This includes defining data quality metrics, assigning data stewards, and establishing data quality rules and standards.
3. Selecting the Right MDM Solution
Choosing the right MDM solution is crucial for the success of your implementation. There are various MDM solutions available in the market, ranging from on-premises to cloud-based, open source to commercial, and industry-specific to generic.
Consider the following factors when selecting an MDM solution:
- Business requirements: Assess your organization's specific business requirements and choose an MDM solution that aligns with those needs. Consider factors such as data volume, complexity, integration requirements, and scalability.
- Functional capabilities: Evaluate the functional capabilities of the MDM solution. Does it support the required data domains, data models, and data integration capabilities? Can it handle complex data hierarchies and relationships?
- Technology stack: Assess the underlying technology stack of the MDM solution. Consider factors such as the database platform, integration capabilities, security features, and scalability.
- Vendor reputation and support: Evaluate the reputation and track record of the MDM solution vendor. Consider factors such as customer reviews, references, and the vendor's ability to provide ongoing support and maintenance.
- Total cost of ownership: Consider the total cost of ownership of the MDM solution, including the initial license fees, implementation costs, maintenance fees, and ongoing operational costs.
4. Establishing Data Governance in MDM
Data governance is a critical component of successful MDM implementations. It involves defining and implementing policies, processes, and controls for managing master data across the organization.
Here are some best practices for establishing data governance in MDM:
- Define data governance roles and responsibilities: Clearly define the roles and responsibilities of data stewards, data owners, and other key stakeholders involved in managing master data. Assign accountability for data quality, data integrity, and data security.
- Establish data governance processes: Define and implement processes for data management, data quality management, data integration, and data security. This includes defining data standards, data policies, and data governance workflows.
- Implement data governance tools and technologies: Leverage data governance tools and technologies to automate and streamline data governance processes. This could include data governance platforms, data cataloging tools, and data lineage tools.
- Ensure executive sponsorship: Secure executive sponsorship and support for data governance initiatives. This includes obtaining buy-in from senior management, securing funding and resources, and aligning data governance with organizational goals and objectives.
- Continuously monitor and measure data governance: Establish metrics and KPIs to monitor the effectiveness of your data governance initiatives. Regularly review and refine your data governance processes based on feedback and insights.
Conclusion
Implementing MDM can be a complex and challenging process, but with the right strategy, tools, and processes in place, organizations can overcome these challenges and unlock the full potential of their master data. By developing a comprehensive MDM strategy, ensuring data quality management, selecting the right MDM solution, and establishing data governance, organizations can achieve the many benefits of MDM, such as improved data quality, increased operational efficiency, and enhanced decision-making capabilities.
Contact us
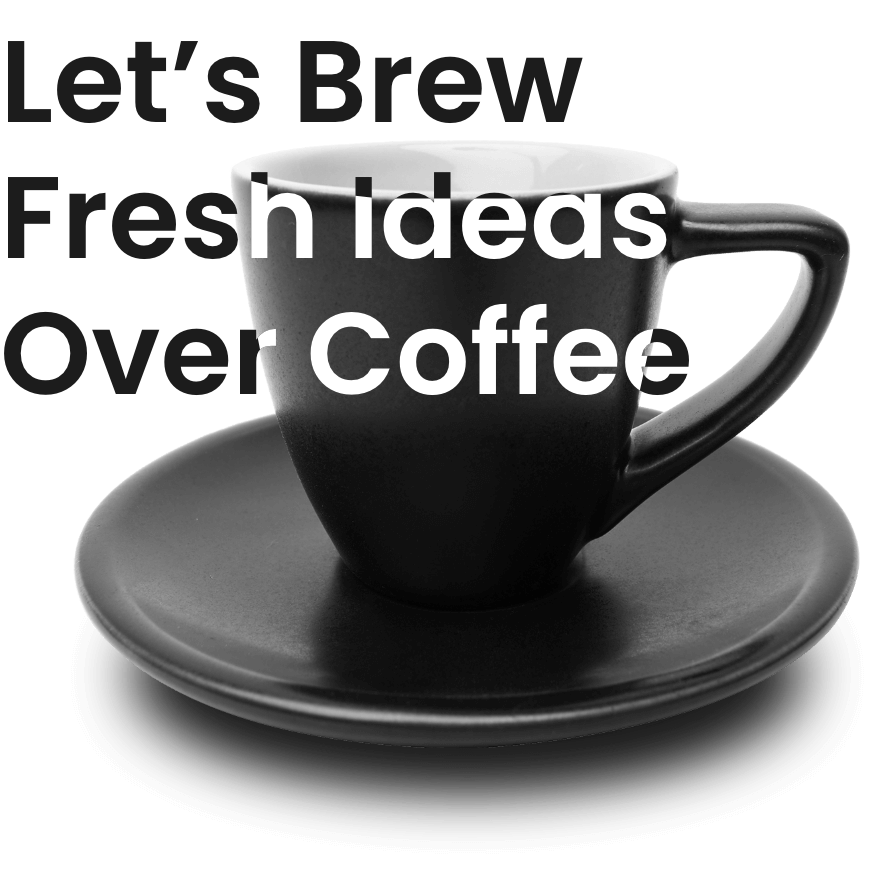
Spanning 8 cities worldwide and with partners in 100 more, we’re your local yet global agency.
Fancy a coffee, virtual or physical? It’s on us – let’s connect!