The benefits of using MDM tools for data warehousing and business intelligence
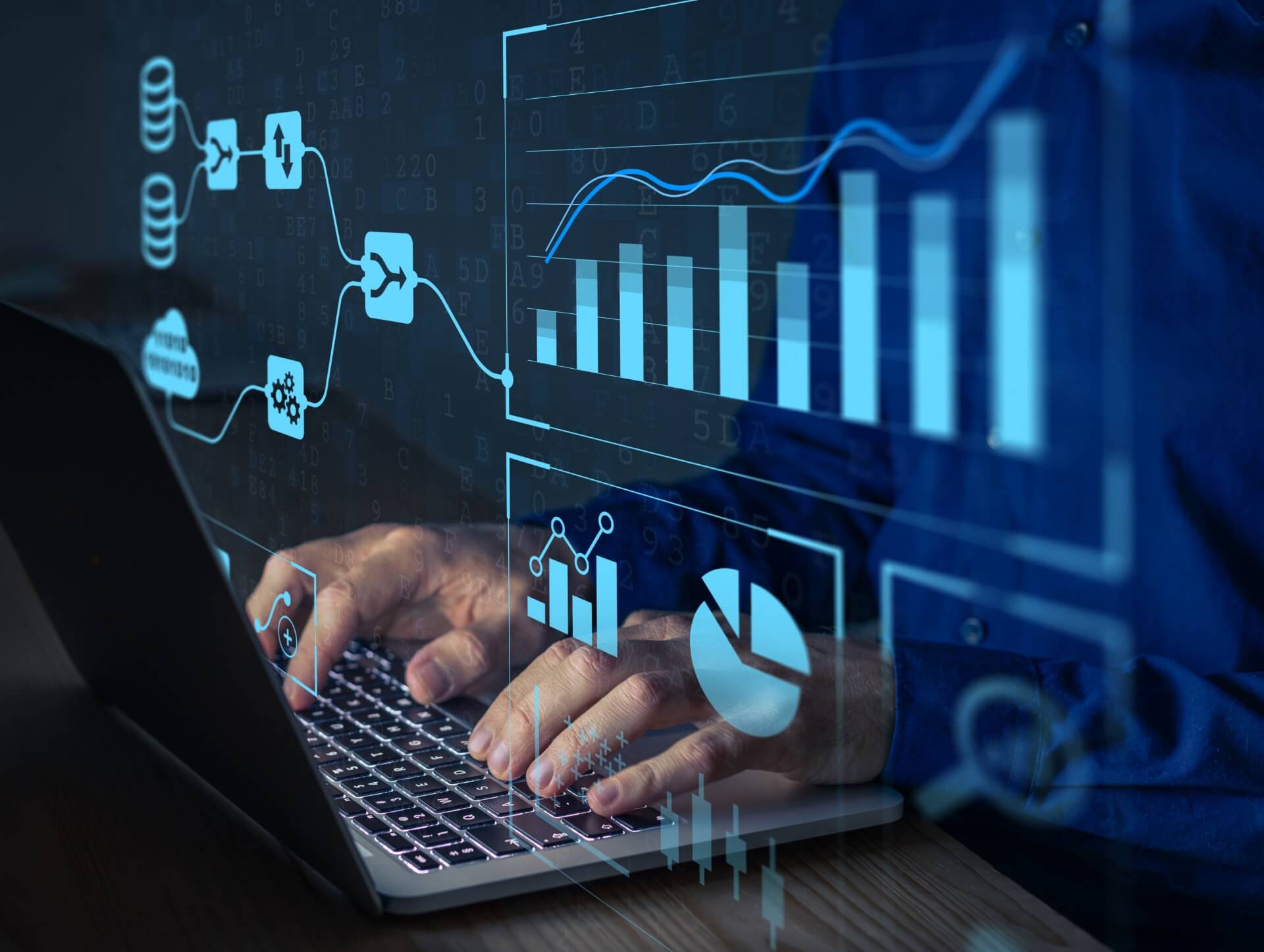
06/09/2023
In today's data-driven world, organizations are constantly dealing with massive amounts of data. This data is not only generated internally but also comes from various external sources. To extract meaningful insights from this data and make informed business decisions, companies need a robust data warehousing and business intelligence infrastructure. Master Data Management (MDM) tools play a crucial role in ensuring data quality, consistency, and accuracy, which are essential for effective data warehousing and business intelligence. In this article, we will explore the benefits of using MDM tools for data warehousing and business intelligence and discuss various aspects related to MDM strategies, implementation processes, and best practices.
1. What is Master Data Management?
Master Data Management (MDM) is a comprehensive approach to managing and integrating an organization's critical data, also known as master data. Master data includes essential entities such as customers, products, suppliers, employees, and other core business data. MDM aims to create a single, accurate, and consistent version of master data across the organization, eliminating data redundancies and discrepancies.
MDM involves various processes, including data governance, data integration, data quality management, and master data modeling. These processes are supported by MDM tools and technologies that provide functionalities for managing master data throughout its lifecycle.
2. The Benefits of Master Data Management
Implementing a robust MDM strategy and using MDM tools offer several benefits for data warehousing and business intelligence:
2.1 Improved Data Quality
One of the primary benefits of MDM is improved data quality. MDM tools help in cleansing and standardizing master data, ensuring consistency and accuracy. By eliminating duplicate and inconsistent data, organizations can rely on high-quality data for their data warehousing and business intelligence initiatives. Clean and accurate data leads to better decision-making and insights.
2.2 Enhanced Data Governance
Data governance is crucial for ensuring data integrity, security, and compliance. MDM tools provide functionalities for establishing data governance policies, rules, and workflows. These tools enable organizations to enforce data governance practices, monitor data quality, and maintain data security throughout the data warehousing and business intelligence processes. Effective data governance ensures that data is trusted and reliable, making it valuable for decision-making.
2.3 Streamlined Data Integration
Integrating data from multiple sources is a complex task. MDM tools simplify data integration processes by providing capabilities for data mapping, transformation, and reconciliation. These tools enable organizations to bring together data from various systems and applications into a centralized repository, ensuring a unified view of master data. Streamlined data integration improves data consistency and accessibility, facilitating effective data warehousing and business intelligence.
2.4 Improved Customer Experience
Customer master data management is a critical aspect of MDM. By maintaining accurate and complete customer data, organizations can enhance the customer experience. MDM tools enable organizations to create a single view of the customer, including their preferences, purchase history, and interactions. This unified customer view helps in personalized marketing, targeted promotions, and improved customer service. A better customer experience leads to increased customer satisfaction and loyalty.
3. MDM Strategies for Effective Data Management
Implementing an MDM strategy is essential for organizations to leverage the full potential of MDM tools. Here are some key strategies for effective data management:
3.1 Define Clear Goals and Objectives
Before implementing an MDM solution, organizations should define clear goals and objectives. These goals can include improving data quality, enhancing data governance, streamlining data integration processes, or achieving a single view of the customer. Clear goals help in aligning the MDM strategy with the overall business objectives and ensure that the implementation process is focused and targeted.
3.2 Establish Data Governance Framework
Data governance is a critical component of an MDM strategy. Organizations should establish a data governance framework that defines data ownership, roles, responsibilities, and data governance policies. This framework ensures that data is managed effectively throughout its lifecycle, from creation to retirement. Data governance provides a structure for data management and ensures that data is trusted, secure, and compliant with regulations.
3.3 Choose the Right MDM Solution
There are various MDM solutions available in the market, ranging from on-premises to cloud-based platforms. Organizations should carefully evaluate their requirements and choose an MDM solution that aligns with their business needs. Factors to consider include scalability, flexibility, data security, integration capabilities, and ease of use. Choosing the right MDM solution is crucial for successful data warehousing and business intelligence initiatives.
3.4 Implement Data Quality Management
Data quality is essential for effective data warehousing and business intelligence. Organizations should implement data quality management processes as part of their MDM strategy. Data quality management involves data profiling, cleansing, enrichment, and monitoring. MDM tools provide functionalities for data quality management, helping organizations maintain high-quality data for their data warehousing and business intelligence initiatives.
4. The Implementation Process of MDM
Implementing an MDM solution involves several steps, including data discovery, data profiling, data mapping, data cleansing, data integration, and data governance. Here is a high-level overview of the MDM implementation process:
4.1 Data Discovery
The first step in the MDM implementation process is data discovery. Organizations need to identify the sources of their master data and understand the data quality issues and inconsistencies. Data discovery involves analyzing the existing data landscape and identifying the data elements that need to be included in the MDM solution.
4.2 Data Profiling
Data profiling is the process of analyzing the quality, structure, and content of the master data. Organizations need to assess the data quality issues, such as duplicates, missing values, and inconsistencies. Data profiling helps in understanding the data quality challenges and forms the basis for data cleansing and transformation.
4.3 Data Mapping
Data mapping involves creating a relationship between the source data elements and the target data elements in the MDM solution. This step helps in defining how the data will be transformed and integrated into the MDM platform. Data mapping ensures that the data is accurately loaded into the MDM solution and aligned with the organization's data model.
4.4 Data Cleansing
Data cleansing is the process of identifying and correcting errors, inconsistencies, and inaccuracies in the master data. MDM tools provide functionalities for data cleansing, including duplicate detection, data standardization, and data enrichment. Data cleansing ensures that the master data is accurate, consistent, and complete, enabling effective data warehousing and business intelligence.
4.5 Data Integration
Data integration involves bringing together data from various sources into a centralized repository. MDM tools facilitate data integration by providing functionalities for data transformation, reconciliation, and consolidation. Data integration ensures a unified view of master data, enabling effective data warehousing and business intelligence.
4.6 Data Governance
Data governance is an ongoing process that ensures the effective management of master data. Organizations need to establish data governance policies, rules, and workflows to maintain data quality, security, and compliance. MDM tools support data governance by providing functionalities for data stewardship, data lineage, and data access control.
5. Best Practices for Effective MDM
To maximize the benefits of MDM tools for data warehousing and business intelligence, organizations should follow these best practices:
5.1 Start with a Pilot Project
Implementing MDM can be a complex and time-consuming process. It is recommended to start with a pilot project to validate the MDM strategy and implementation approach. A pilot project helps in identifying any challenges or issues early on and allows organizations to make necessary adjustments before scaling up the implementation.
5.2 Involve Stakeholders from Different Departments
MDM initiatives involve multiple stakeholders from different departments, including IT, data governance, business operations, and analytics. It is important to involve these stakeholders from the beginning to ensure their buy-in and alignment with the MDM strategy. Collaborative efforts and cross-functional teamwork are essential for successful MDM implementation.
5.3 Establish Data Governance Council
Creating a data governance council can help in driving data governance initiatives and ensuring the long-term success of MDM. The data governance council should consist of representatives from different departments and should be responsible for defining data governance policies, resolving data-related issues, and monitoring data quality and compliance.
5.4 Continuously Monitor and Improve Data Quality
Data quality is not a one-time activity but an ongoing process. Organizations should continuously monitor and improve data quality to ensure the accuracy and reliability of master data. Regular data quality assessments, data profiling, and data cleansing activities should be performed to maintain high-quality data for data warehousing and business intelligence.
6. Challenges in MDM
While MDM offers numerous benefits, organizations may also face several challenges during the implementation process. Some common challenges include:
6.1 Data Complexity
Organizations often have complex data landscapes, with data residing in various systems and applications. Managing and integrating this data can be challenging, requiring careful planning and execution. Data complexity can lead to issues such as data inconsistencies, data redundancies, and data quality problems.
6.2 Data Governance
Establishing and maintaining effective data governance practices can be a challenge. Data governance involves defining data ownership, roles, responsibilities, and policies. It requires collaboration and coordination among different departments and stakeholders. Lack of proper data governance can result in data security breaches, compliance issues, and data quality problems.
6.3 Data Integration
Data integration is a complex process, especially when dealing with data from multiple sources. Inconsistent data formats, data structures, and data quality issues can make data integration challenging. Organizations need to invest in robust data integration tools and technologies to ensure seamless data integration for effective data warehousing and business intelligence.
6.4 Change Management
Implementing an MDM solution involves significant changes in data management processes and workflows. Change management can be challenging, as it requires organizations to change their existing data management practices and adopt new technologies and methodologies. Proper change management strategies and training programs are essential to ensure successful MDM implementation.
7. MDM Case Studies
Several organizations have successfully implemented MDM solutions and achieved significant benefits. Here are a few case studies:
7.1 Case Study 1: Company XYZ
Company XYZ, a global retail chain, implemented an MDM solution to manage their customer data. By creating a single view of the customer, they were able to personalize their marketing campaigns and improve customer satisfaction. The MDM solution helped them identify cross-selling opportunities and target specific customer segments, leading to increased revenue and customer loyalty.
7.2 Case Study 2: Company ABC
Company ABC, a manufacturing company, implemented an MDM solution to streamline their product data management. By eliminating duplicate and inconsistent product data, they were able to improve their supply chain efficiency and reduce product information errors. The MDM solution helped them maintain accurate and up-to-date product information, enabling better decision-making and improved customer service.
7.3 Case Study 3: Company DEF
Company DEF, a financial services organization, implemented an MDM solution to manage their financial customer data. By ensuring data accuracy and consistency, they were able to comply with regulatory requirements and reduce the risk of financial fraud. The MDM solution helped them improve data security and integrity, enhancing customer trust and loyalty.
8. Conclusion
Master Data Management (MDM) tools play a crucial role in ensuring data quality, consistency, and accuracy for data warehousing and business intelligence. By implementing an effective MDM strategy and using the right MDM tools, organizations can improve data quality, enhance data governance, streamline data integration processes, and deliver better customer experiences. MDM offers numerous benefits, but organizations should also be aware of the challenges involved in MDM implementation. By following best practices and learning from successful case studies, organizations can leverage MDM tools to maximize the value of their data and drive business growth.
Contact us
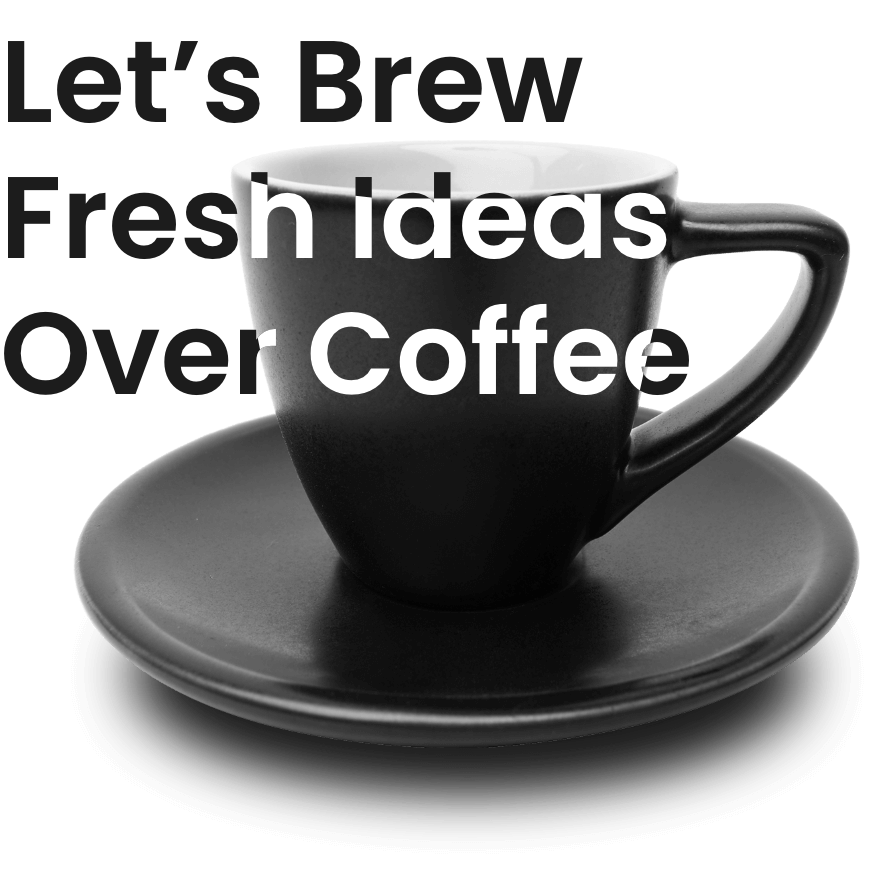
Spanning 8 cities worldwide and with partners in 100 more, we’re your local yet global agency.
Fancy a coffee, virtual or physical? It’s on us – let’s connect!