The challenges of data governance in MDM implementation
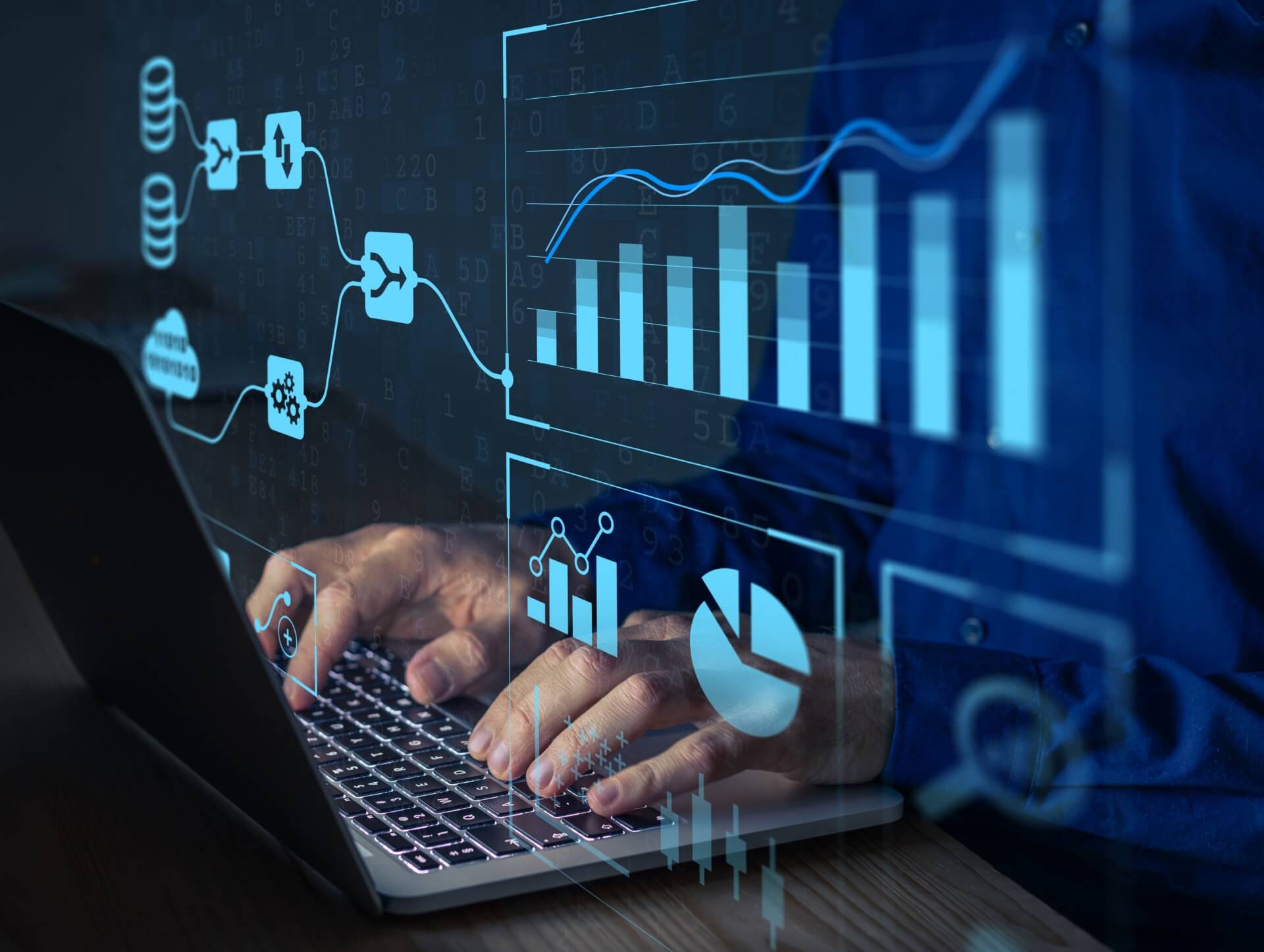
06/09/2023
Master Data Management (MDM) is a comprehensive approach to managing and organizing an organization's critical data to ensure accuracy, consistency, and reliability. It involves the creation and implementation of a master data management strategy, which includes defining data governance policies, establishing data quality management processes, and selecting the right MDM tools and technologies.
While the benefits of master data management are well-known, implementing MDM can be a complex and challenging process, especially when it comes to data governance. In this article, we will explore the challenges organizations face in implementing data governance in MDM and discuss best practices to overcome them.
Challenges in Data Governance in MDM
Data governance in MDM involves defining and enforcing policies and procedures for managing, protecting, and using master data throughout its lifecycle. However, several challenges can hinder the successful implementation of data governance in MDM. Let's take a closer look at some of these challenges:
1. Lack of Data Ownership and Accountability
One of the primary challenges in implementing data governance in MDM is the lack of clear data ownership and accountability. Without clear ownership, it becomes difficult to establish and enforce data governance policies and procedures. Data ownership should be assigned to individuals or teams responsible for the management and quality of specific master data domains, such as customer data, product data, or financial data.
To address this challenge, organizations must clearly define data ownership roles and responsibilities and ensure that these roles are well-communicated and understood across the organization. Data stewards can be appointed to take ownership of specific master data domains and ensure data quality and compliance with data governance policies and standards.
2. Data Quality Management
Data quality is crucial for the success of any MDM implementation. Poor data quality can lead to erroneous insights, inaccurate reporting, and unreliable decision-making. However, ensuring data quality is a challenging task, especially when dealing with large volumes of master data from multiple sources.
To overcome this challenge, organizations need to establish robust data quality management processes as part of their data governance framework. This includes defining data quality metrics and standards, implementing data cleansing and enrichment techniques, and regularly monitoring and measuring data quality. Data quality tools and technologies can also be leveraged to automate data quality management processes and ensure consistent data quality across the organization.
3. Data Integration in MDM
Data integration is a critical aspect of MDM, as it involves consolidating and harmonizing data from multiple sources to create a single, unified view of master data. However, integrating data from disparate sources can be challenging due to differences in data formats, structures, and semantics.
To address this challenge, organizations should invest in robust data integration tools and technologies that support data mapping, transformation, and cleansing. Data integration processes should be well-defined and standardized to ensure consistency and accuracy in data integration. Additionally, organizations should establish data integration best practices and guidelines to streamline the data integration process and avoid common pitfalls.
4. Data Security and Privacy
Data security and privacy are critical considerations in MDM implementation, as master data often contains sensitive and confidential information. Ensuring data security throughout the data lifecycle is a complex task, especially with the increasing number of data breaches and privacy regulations.
To overcome this challenge, organizations should implement robust data security measures as part of their data governance framework. This includes establishing access controls and user permissions, encrypting sensitive data, monitoring data access and usage, and regularly auditing and assessing data security measures. Compliance with data privacy regulations, such as GDPR or CCPA, should also be a top priority for organizations implementing MDM.
Best Practices for Overcoming Data Governance Challenges in MDM
While the challenges in implementing data governance in MDM can be daunting, organizations can overcome them by following these best practices:
1. Define a Clear Data Governance Strategy
A well-defined data governance strategy is essential for successful MDM implementation. This strategy should include clear objectives, roles, and responsibilities, as well as guidelines for data governance policies and procedures. It should also align with the organization's overall business goals and objectives.
Organizations should involve key stakeholders from different departments and functions in the development of the data governance strategy to ensure buy-in and alignment. The strategy should be communicated effectively to all employees to create awareness and understanding of the importance of data governance in MDM.
2. Establish Data Governance Policies and Standards
Data governance policies and standards provide the framework for managing and governing master data. These policies and standards should cover data ownership, data quality, data integration, data security, and compliance with regulatory requirements.
Organizations should establish a governance council or committee responsible for defining and enforcing these policies and standards. The council should include representatives from different business units and functions and should meet regularly to review and update the policies and standards as needed.
3. Invest in the Right MDM Tools and Technologies
Choosing the right MDM tools and technologies is crucial for successful data governance in MDM. Organizations should evaluate different MDM solutions and select the one that best fits their requirements and objectives.
Open-source MDM solutions and cloud-based MDM platforms are gaining popularity due to their flexibility, scalability, and cost-effectiveness. However, organizations should carefully consider their specific needs and evaluate the features, capabilities, and security of the MDM solution before making a decision.
4. Establish Data Governance Metrics and KPIs
To monitor the effectiveness of data governance in MDM, organizations should establish data governance metrics and key performance indicators (KPIs). These metrics and KPIs should align with the organization's overall business goals and objectives and should be regularly monitored and measured.
Some common data governance metrics include data quality metrics (e.g., data completeness, data accuracy, data consistency), data security metrics (e.g., number of data breaches, data access controls), and data compliance metrics (e.g., compliance with regulatory requirements).
Conclusion
Implementing data governance in MDM can be a complex and challenging process. However, by addressing the challenges mentioned above and following the best practices outlined, organizations can overcome these challenges and successfully implement data governance in MDM.
Data governance is a critical component of any MDM implementation, as it ensures the accuracy, consistency, and reliability of master data. It also enables organizations to derive valuable insights, make informed decisions, and improve customer experience.
By investing in the right MDM tools and technologies, establishing robust data governance policies and standards, and involving key stakeholders in the data governance process, organizations can unlock the full potential of their master data and drive business growth and success.
Contact us
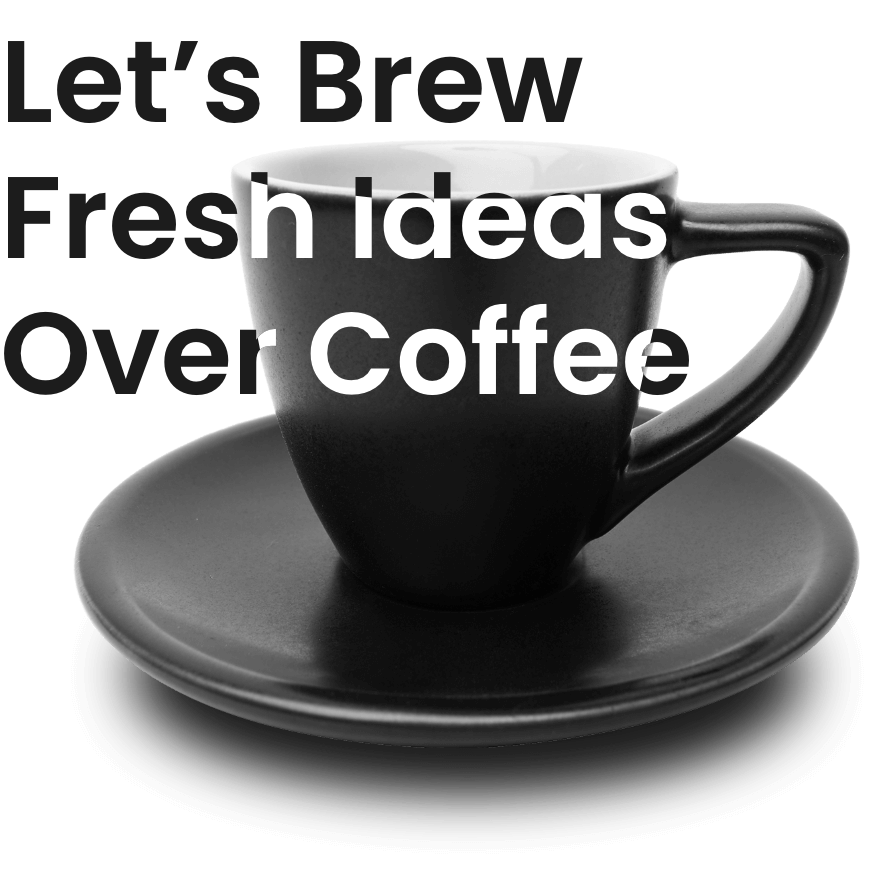
Spanning 8 cities worldwide and with partners in 100 more, we’re your local yet global agency.
Fancy a coffee, virtual or physical? It’s on us – let’s connect!