The challenges of data governance in real-time MDM implementation
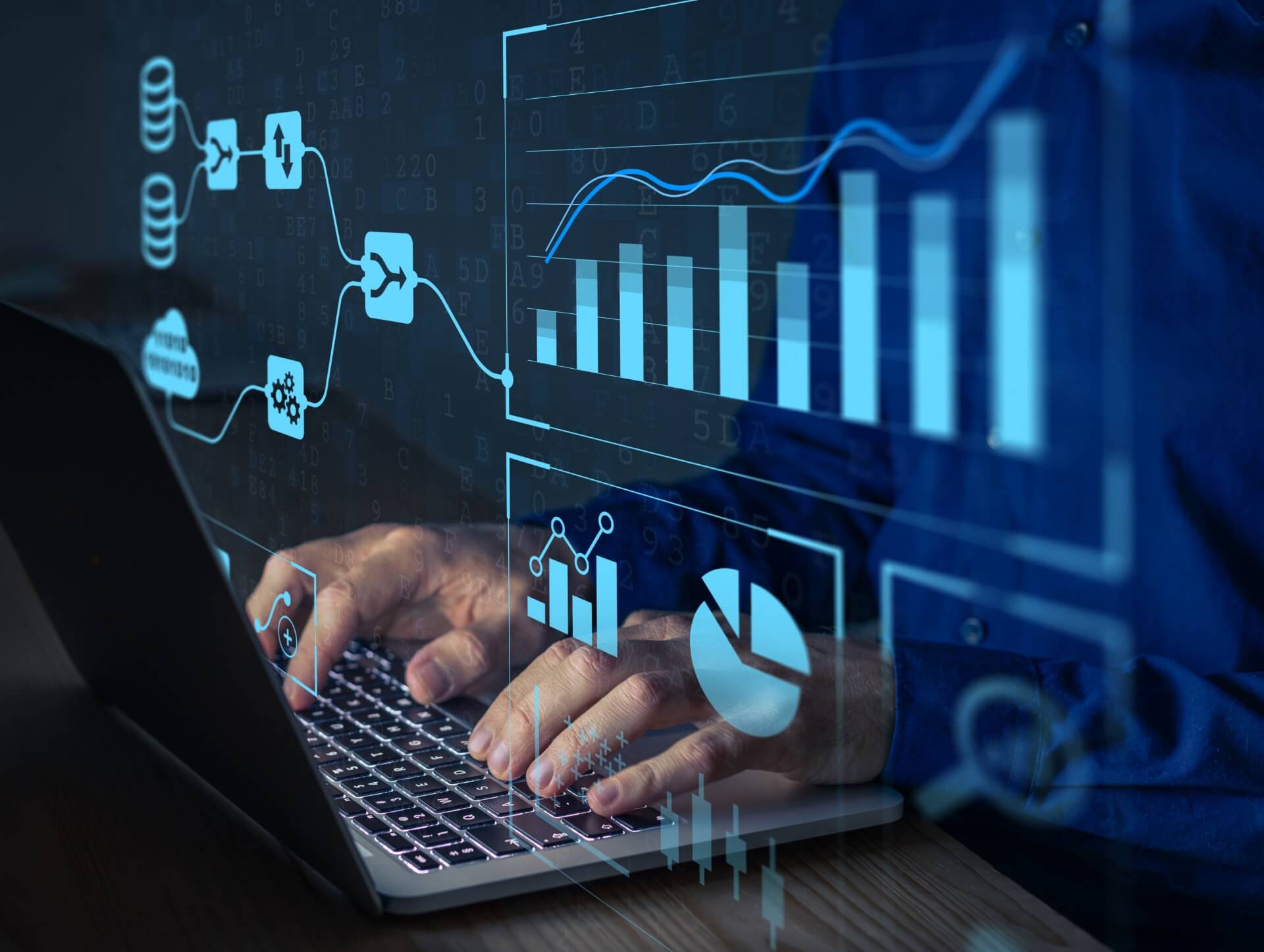
06/09/2023
Master Data Management (MDM) is a comprehensive approach to managing and integrating an organization's critical data. It involves the development and implementation of a master data management strategy, which includes processes, policies, and technologies to ensure the accurate and consistent management of master data across the enterprise. MDM provides numerous benefits, such as improved data quality, increased operational efficiency, and enhanced decision-making capabilities.
In today's fast-paced business environment, real-time MDM implementation has become increasingly important. Real-time MDM enables organizations to access and utilize their master data in real-time, allowing for more agile and responsive decision-making. However, implementing real-time MDM comes with its own set of challenges, particularly in the area of data governance.
Data Governance in Real-Time MDM
Data governance in MDM refers to the processes and policies that govern the management, usage, and security of master data. It ensures that master data is accurate, complete, consistent, and up-to-date. In the context of real-time MDM implementation, data governance becomes even more critical.
One of the main challenges in real-time MDM implementation is ensuring data quality management. Real-time data integration requires the ability to collect, validate, and cleanse data in real-time. This requires robust data quality management processes and technologies to identify and resolve data quality issues as they occur. Without proper data quality management, organizations risk making decisions based on inaccurate or incomplete data, leading to negative business outcomes.
Another challenge is developing an effective MDM strategy for businesses. Real-time MDM requires organizations to define and implement processes for capturing, validating, and updating master data in real-time. This involves establishing data governance policies, defining data ownership and stewardship roles, and implementing data governance tools and technologies. Developing an effective MDM strategy requires careful planning and coordination across different departments and stakeholders.
Data security is also a significant concern in real-time MDM implementation. Real-time access to master data introduces new security risks, as organizations need to ensure that only authorized users can access and modify master data. Implementing robust data security measures, such as encryption, access controls, and audit trails, is essential to protect sensitive master data from unauthorized access and ensure compliance with data privacy regulations.
Challenges in Data Lifecycle Management
Data lifecycle management refers to the process of managing data throughout its lifecycle, from creation to deletion. It involves various stages, including data capture, storage, processing, analysis, and archiving. Real-time MDM implementation introduces unique challenges in data lifecycle management.
One of the challenges is ensuring data compliance in management. Real-time MDM requires organizations to comply with data privacy regulations, industry standards, and internal data governance policies. This involves establishing processes and controls to ensure that master data is managed in accordance with relevant regulations and policies. Failure to comply with data compliance requirements can result in legal and financial consequences for organizations.
Data security throughout the lifecycle is another challenge in real-time MDM implementation. Organizations need to implement robust data security measures at each stage of the data lifecycle to protect master data from unauthorized access, modification, or deletion. This includes securing data during transmission, storage, and processing, as well as implementing data backup and recovery mechanisms to ensure data availability and integrity.
Metadata management is also a critical aspect of data lifecycle management in real-time MDM. Metadata provides context and meaning to master data, enabling organizations to understand and utilize the data effectively. Managing metadata involves capturing, storing, and maintaining metadata throughout the data lifecycle. This ensures that master data remains accurate, consistent, and accessible, even as it undergoes changes and updates.
Automation plays a crucial role in real-time MDM implementation and data lifecycle management. Real-time MDM requires automated processes and workflows to collect, validate, and update master data in real-time. Automation reduces manual effort, improves data accuracy, and enhances operational efficiency. Organizations need to leverage automation technologies, such as data integration tools, data quality tools, and workflow engines, to streamline their data lifecycle management processes.
Best Practices for Data Governance in Real-Time MDM Implementation
To overcome the challenges of data governance in real-time MDM implementation, organizations should follow best practices:
- Define clear data governance policies and procedures: Establish clear policies and procedures for managing master data, including data quality standards, data ownership, data stewardship roles, and data access controls.
- Implement data governance tools and technologies: Invest in data governance tools and technologies, such as data quality tools, data integration tools, and data security solutions, to automate and streamline data governance processes.
- Establish data governance committees: Create cross-functional data governance committees to oversee and govern the implementation of MDM and ensure alignment with business objectives.
- Train and educate employees: Provide training and education to employees on data governance principles, processes, and tools. This will enable them to understand their roles and responsibilities in data governance and ensure compliance with data governance policies.
- Monitor and measure data governance effectiveness: Regularly monitor and measure the effectiveness of data governance processes and policies. Use key performance indicators (KPIs) to assess data quality, data compliance, and data security metrics.
- Continuously improve data governance practices: Continuously review and improve data governance practices based on feedback and insights gained from monitoring and measuring data governance effectiveness.
Conclusion
Data governance is a critical component of real-time MDM implementation. It ensures that master data is accurate, complete, consistent, and up-to-date, enabling organizations to make informed decisions and achieve business objectives. However, implementing data governance in real-time MDM comes with its own set of challenges, such as data quality management, MDM strategy development, and data security. By following best practices and leveraging appropriate tools and technologies, organizations can overcome these challenges and successfully implement real-time MDM with robust data governance.
Contact us
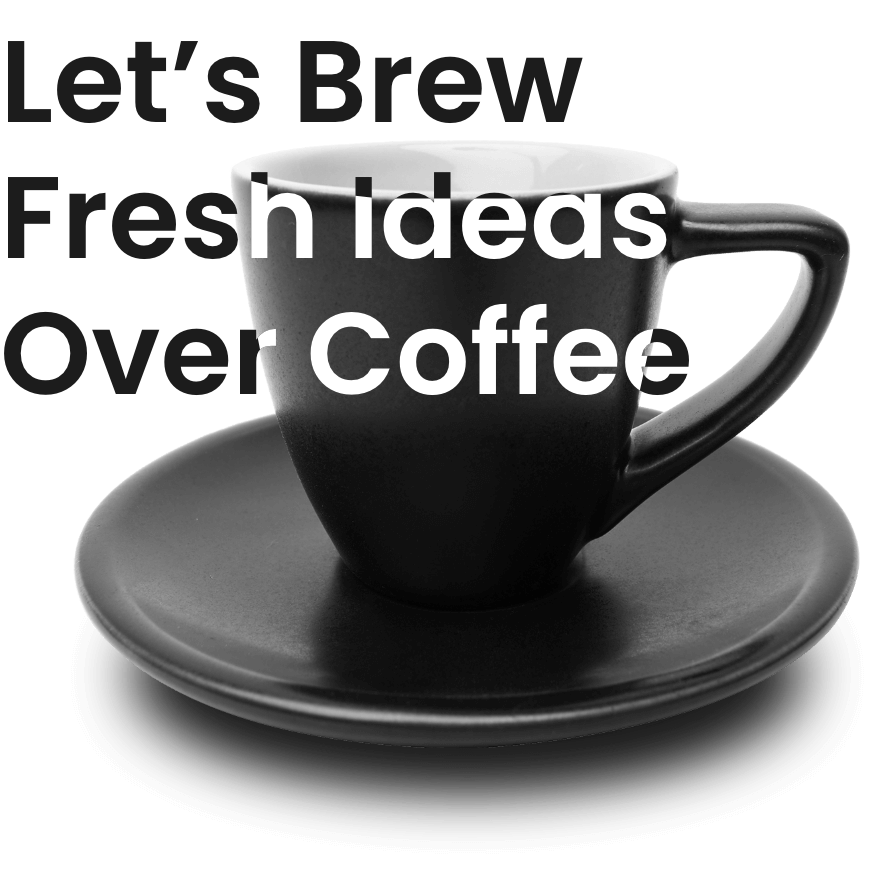
Spanning 8 cities worldwide and with partners in 100 more, we’re your local yet global agency.
Fancy a coffee, virtual or physical? It’s on us – let’s connect!