The challenges of data integration in MDM implementation
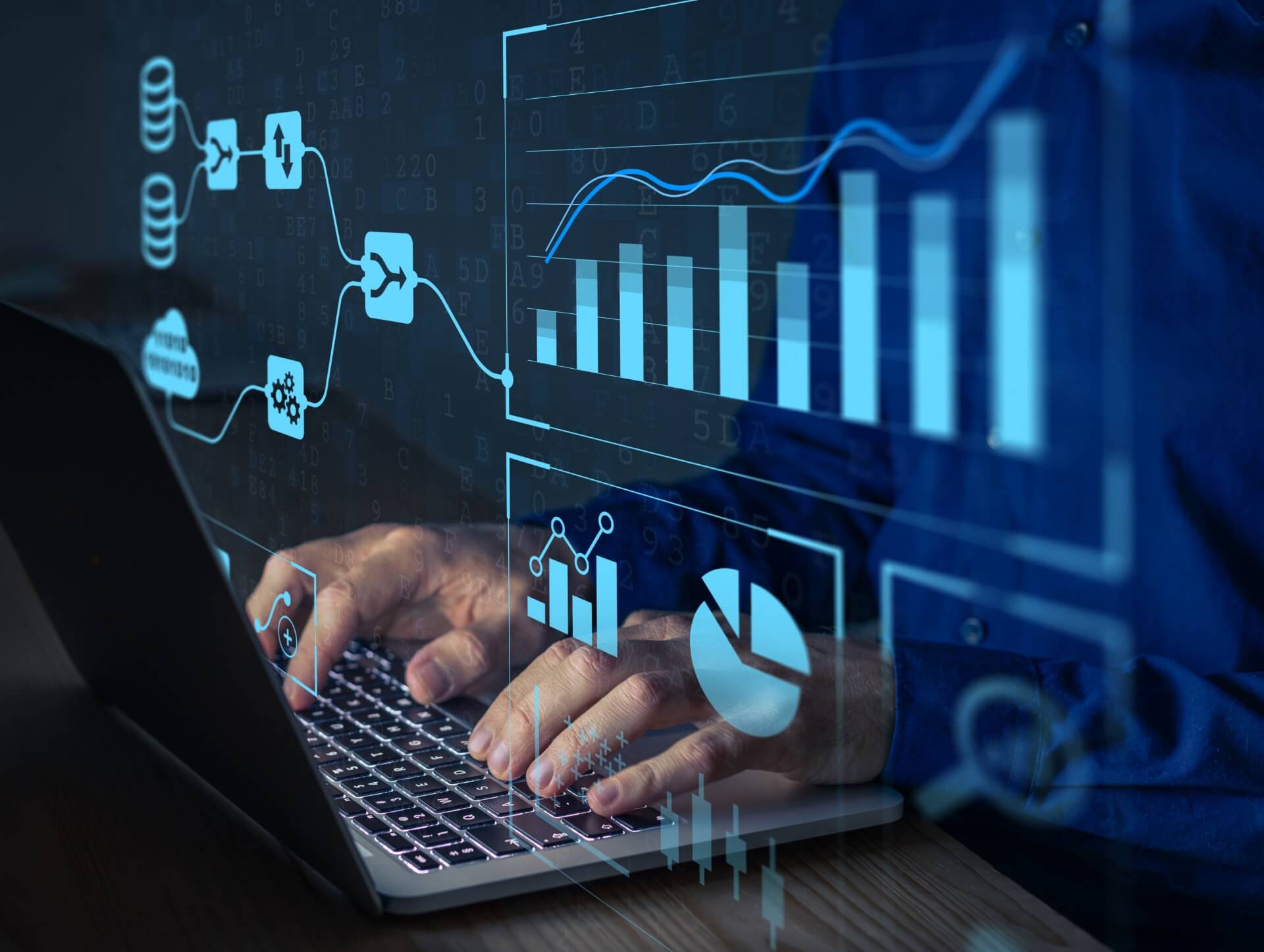
06/09/2023
Master Data Management (MDM) is a crucial process for businesses to ensure the accuracy, consistency, and reliability of their critical data. It involves creating a single, trusted source of master data across all systems and applications within an organization.
MDM solutions provide a centralized platform for managing and governing master data, enabling organizations to improve data quality, streamline business processes, and make informed decisions based on reliable data. However, implementing MDM comes with its own set of challenges, particularly in the area of data integration.
The Importance of Data Integration in MDM
Data integration is the process of combining data from different sources and formats to create a unified view of the data. In the context of MDM, data integration is critical because it involves bringing together data from various systems and applications to create a single, consistent, and accurate master data record.
Without effective data integration, organizations may end up with duplicate, incomplete, or inconsistent data, leading to data quality issues and unreliable master data. Data integration ensures that all relevant data is captured, validated, and transformed into a standardized format, ready to be used for MDM purposes.
The Challenges of Data Integration in MDM Implementation
Implementing data integration in MDM can be challenging due to various factors. Let's explore some of the common challenges organizations face:
1. Complex Data Landscape
Organizations often have complex data landscapes with data residing in multiple systems, databases, and applications. Each of these systems may have its own data formats, structures, and quality standards. Integrating data from these disparate sources and ensuring its consistency and accuracy can be a complex task.
Organizations need to invest in data integration tools and technologies that can handle the complexity of their data landscape and enable seamless integration of data from various sources. These tools should support data transformation, cleansing, and enrichment to ensure that the integrated data meets the required quality standards.
2. Data Mapping and Transformation
Data integration involves mapping and transforming data from different sources into a common format that aligns with the master data model. This process requires a deep understanding of the data semantics, structures, and relationships.
Organizations need to invest time and effort in defining and documenting data mapping rules and transformation logic. This includes identifying the attributes and fields that need to be integrated, defining the mapping rules between the source and target attributes, and implementing the necessary transformations to ensure data consistency and quality.
3. Data Quality Management
Poor data quality can significantly impact the success of MDM implementation. Data integration brings together data from various sources, and if the quality of the source data is not up to the mark, it can lead to inaccurate and unreliable master data.
Organizations need to implement data quality management practices and tools to ensure the integrity and accuracy of the integrated data. This involves data profiling, cleansing, standardization, and validation to identify and correct any data quality issues before integrating the data into the master data repository.
4. Data Governance and Security
Data integration in MDM raises concerns about data governance and security. Organizations need to establish data governance policies and processes to ensure that the integrated data is governed and managed effectively.
Data security is also a critical aspect of data integration in MDM. Organizations need to implement robust security measures to protect the integrated data from unauthorized access, data breaches, and other security threats.
Overcoming Data Integration Challenges in MDM
While data integration challenges in MDM can be complex, organizations can overcome them by following these best practices:
1. Define a Clear MDM Strategy
A well-defined MDM strategy is essential to guide the data integration process. Organizations should clearly define their MDM goals, objectives, and desired outcomes. This will help in identifying the relevant data sources, understanding the data integration requirements, and defining the necessary data mappings and transformations.
Having a clear MDM strategy also ensures that the data integration efforts align with the overall business objectives and helps in gaining stakeholder buy-in and support.
2. Invest in the Right Tools and Technologies
Choosing the right data integration tools and technologies is crucial for successful MDM implementation. Organizations should evaluate and select tools that can handle the complexity of their data landscape, support data transformation and cleansing, and provide robust data quality management capabilities.
Open source MDM tools and cloud-based MDM platforms are gaining popularity due to their flexibility, scalability, and cost-effectiveness. Organizations should consider these options while selecting the tools and technologies for data integration in MDM.
3. Establish Data Governance Framework
Data governance plays a vital role in ensuring the success of data integration in MDM. Organizations should establish a data governance framework that defines the roles, responsibilities, and processes for governing the integrated data.
This includes defining data ownership, data stewardship, data quality standards, and data access policies. Regular data governance audits and reviews should be conducted to ensure that the integrated data remains accurate, consistent, and compliant with regulatory requirements.
4. Implement Data Quality Management Practices
Data quality management is critical for successful data integration in MDM. Organizations should implement data profiling, cleansing, standardization, and validation processes to ensure the integrity and accuracy of the integrated data.
Data quality tools and technologies can help in identifying and resolving data quality issues, such as duplicates, inconsistencies, and incomplete data. Organizations should also establish data quality metrics and KPIs to measure and monitor the data quality of the integrated data.
Conclusion
Data integration is a critical aspect of MDM implementation. It involves bringing together data from various sources and formats to create a unified view of the data. However, data integration in MDM comes with its own set of challenges, such as complex data landscapes, data mapping and transformation, data quality management, and data governance and security.
Organizations can overcome these challenges by defining a clear MDM strategy, investing in the right tools and technologies, establishing a data governance framework, and implementing data quality management practices. By addressing these challenges effectively, organizations can ensure the success of their MDM implementation and leverage the benefits of accurate and reliable master data.
Contact us
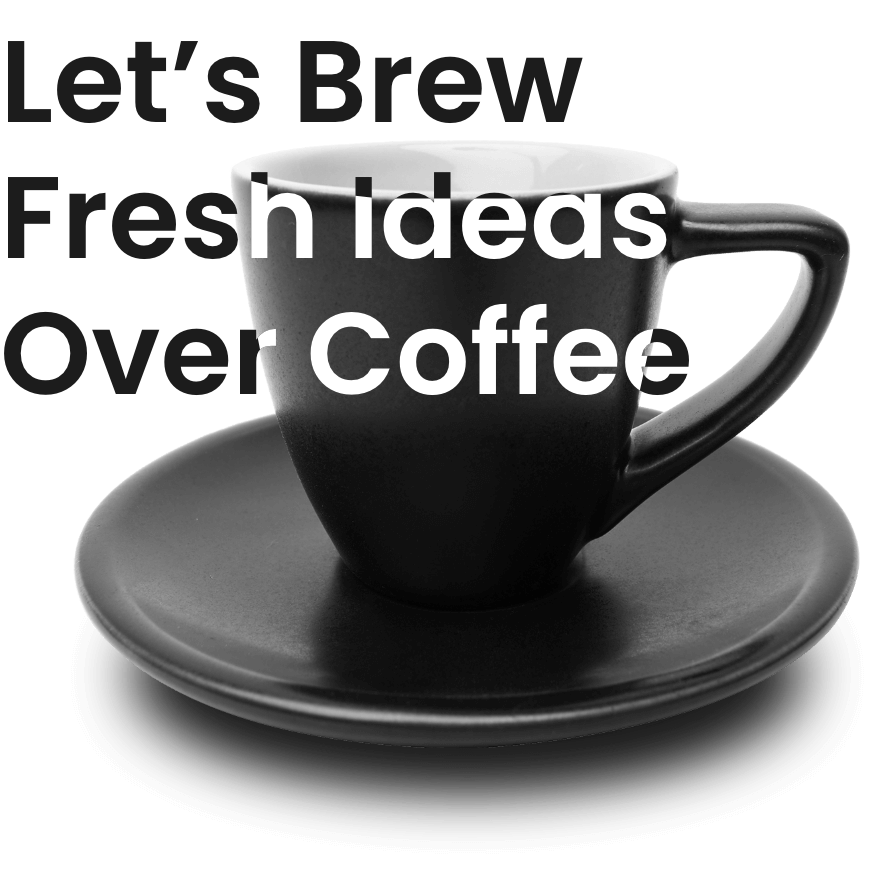
Spanning 8 cities worldwide and with partners in 100 more, we’re your local yet global agency.
Fancy a coffee, virtual or physical? It’s on us – let’s connect!