The challenges of data migration strategies in MDM implementation
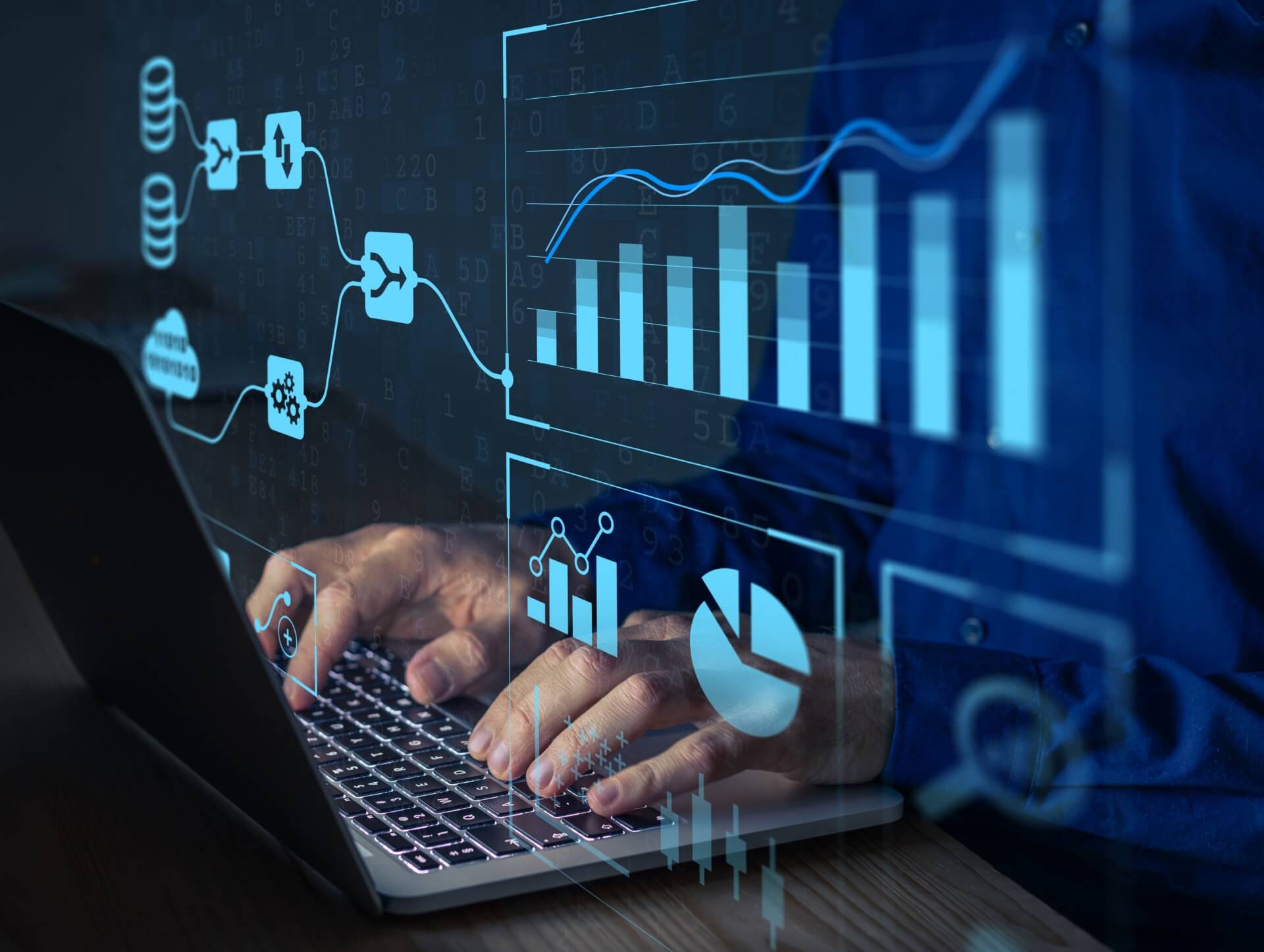
06/09/2023
Master Data Management (MDM) is a critical process for organizations to ensure consistent and accurate data across various systems and applications. It involves the management of master data, which refers to the key data entities that are common and shared across different business units and processes. MDM helps organizations improve data quality, streamline operations, and enable better decision-making. However, implementing MDM can be a complex and challenging task, especially when it comes to data migration strategies.
Understanding Data Migration in MDM
Data migration is the process of transferring data from one system to another, ensuring data integrity and consistency. In the context of MDM, data migration is crucial as it involves consolidating and integrating data from various sources into a centralized master data repository. This repository serves as the single source of truth for master data, providing a unified and accurate view of data across the organization. However, data migration in MDM implementation poses several challenges that organizations need to address.
Challenges in Data Migration Strategies
1. Data Quality Management:
One of the key challenges in data migration strategies for MDM implementation is ensuring data quality. Data quality refers to the accuracy, completeness, consistency, and relevance of data. During the data migration process, organizations need to cleanse and validate the data to ensure that only high-quality data is migrated to the master data repository. This involves identifying and resolving data quality issues such as duplicate records, missing values, and inconsistencies. Implementing effective data quality management practices and utilizing data cleansing tools can help overcome this challenge.
2. Data Integration:
Data integration is another significant challenge in data migration strategies for MDM implementation. Organizations often have data spread across multiple systems and databases, making it difficult to integrate and consolidate the data into a centralized repository. Data integration involves mapping and transforming data from different sources to match the format and structure of the master data repository. It requires careful planning, data mapping, and data transformation to ensure seamless integration. Utilizing data integration tools and technologies can simplify this process and overcome integration challenges.
3. Data Governance:
Data governance plays a crucial role in data migration strategies for MDM implementation. It involves defining and implementing policies, processes, and controls to ensure data quality, privacy, and security. Data governance helps organizations establish data standards, enforce data management practices, and ensure compliance with regulations. During the data migration process, organizations need to ensure that data governance principles are followed to maintain data integrity and protect sensitive information. Implementing a robust data governance framework and utilizing data governance tools can address this challenge effectively.
4. Change Management:
Change management is a significant challenge in data migration strategies for MDM implementation. Migrating data to a new system or platform involves significant changes in processes, systems, and workflows. Employees need to adapt to the new system and understand the benefits of MDM. Resistance to change and lack of awareness can hinder the success of data migration and MDM implementation. Organizations need to invest in change management strategies, communication, and training to ensure smooth transition and adoption of the new system.
Best Practices for Data Migration in MDM Implementation
1. Define Clear Goals and Objectives:
Before embarking on data migration for MDM implementation, organizations should clearly define their goals and objectives. This includes understanding the specific business needs, identifying the key data entities to be managed, and setting measurable targets for data quality improvement. Clear goals and objectives provide a roadmap for the data migration process and help organizations stay focused on achieving their desired outcomes.
2. Assess Data Quality:
Prior to data migration, organizations should conduct a comprehensive assessment of data quality. This includes identifying data quality issues, assessing data completeness and accuracy, and identifying data sources and systems for migration. Assessing data quality helps organizations prioritize data cleansing and validation activities and ensures that only high-quality data is migrated to the master data repository. Data quality assessment should be an ongoing process throughout the data migration and MDM implementation phases.
3. Plan Data Mapping and Transformation:
Data mapping and transformation are critical steps in data migration for MDM implementation. Organizations should develop a clear and detailed plan for mapping data from different sources to the master data repository. This involves defining data mapping rules, identifying data transformation requirements, and addressing data format and structure differences. A well-defined data mapping and transformation plan helps ensure data integrity and consistency in the master data repository.
4. Implement Data Validation and Cleansing:
Data validation and cleansing are essential for ensuring data quality during the data migration process. Organizations should implement data validation checks to identify and resolve data quality issues such as duplicate records, missing values, and inconsistencies. Data cleansing tools and technologies can be utilized to automate the data validation and cleansing process, saving time and effort. Regular data validation and cleansing should be performed to maintain data quality in the master data repository.
Conclusion
Data migration is a crucial aspect of MDM implementation, and organizations need to overcome various challenges to ensure a successful migration process. By addressing challenges such as data quality management, data integration, data governance, and change management, organizations can achieve the benefits of MDM, including improved data quality, streamlined operations, and better decision-making. Implementing best practices such as defining clear goals and objectives, assessing data quality, planning data mapping and transformation, and implementing data validation and cleansing can help organizations overcome these challenges and achieve a successful data migration in MDM implementation.
Contact us
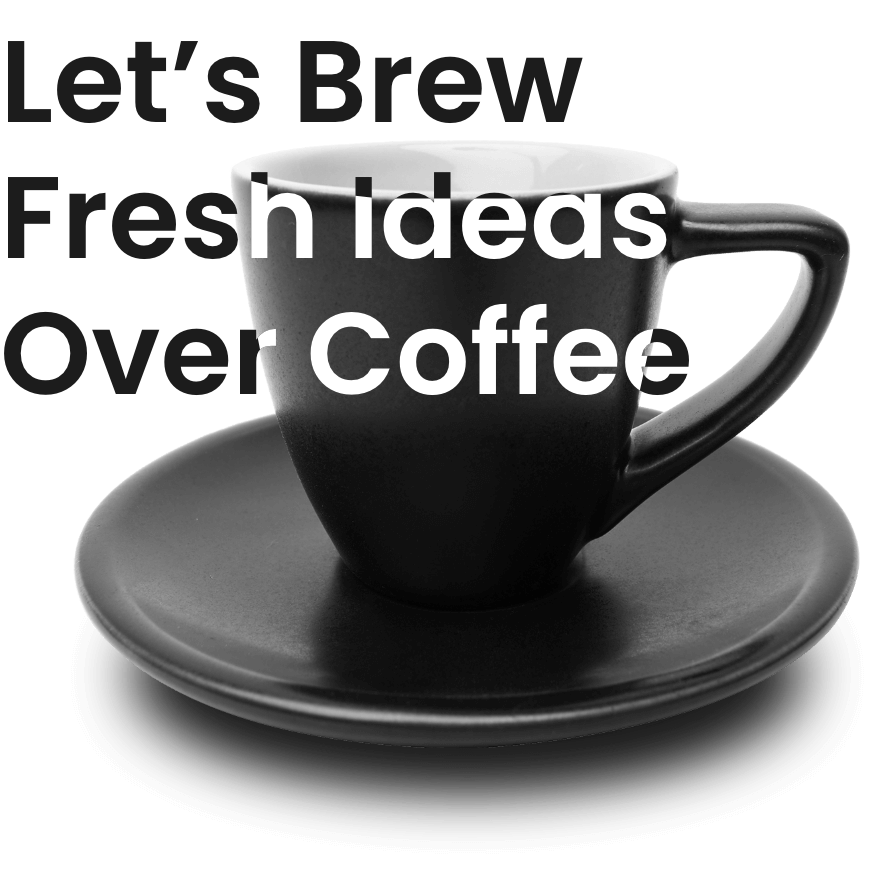
Spanning 8 cities worldwide and with partners in 100 more, we’re your local yet global agency.
Fancy a coffee, virtual or physical? It’s on us – let’s connect!