The challenges of data standardization in MDM implementation
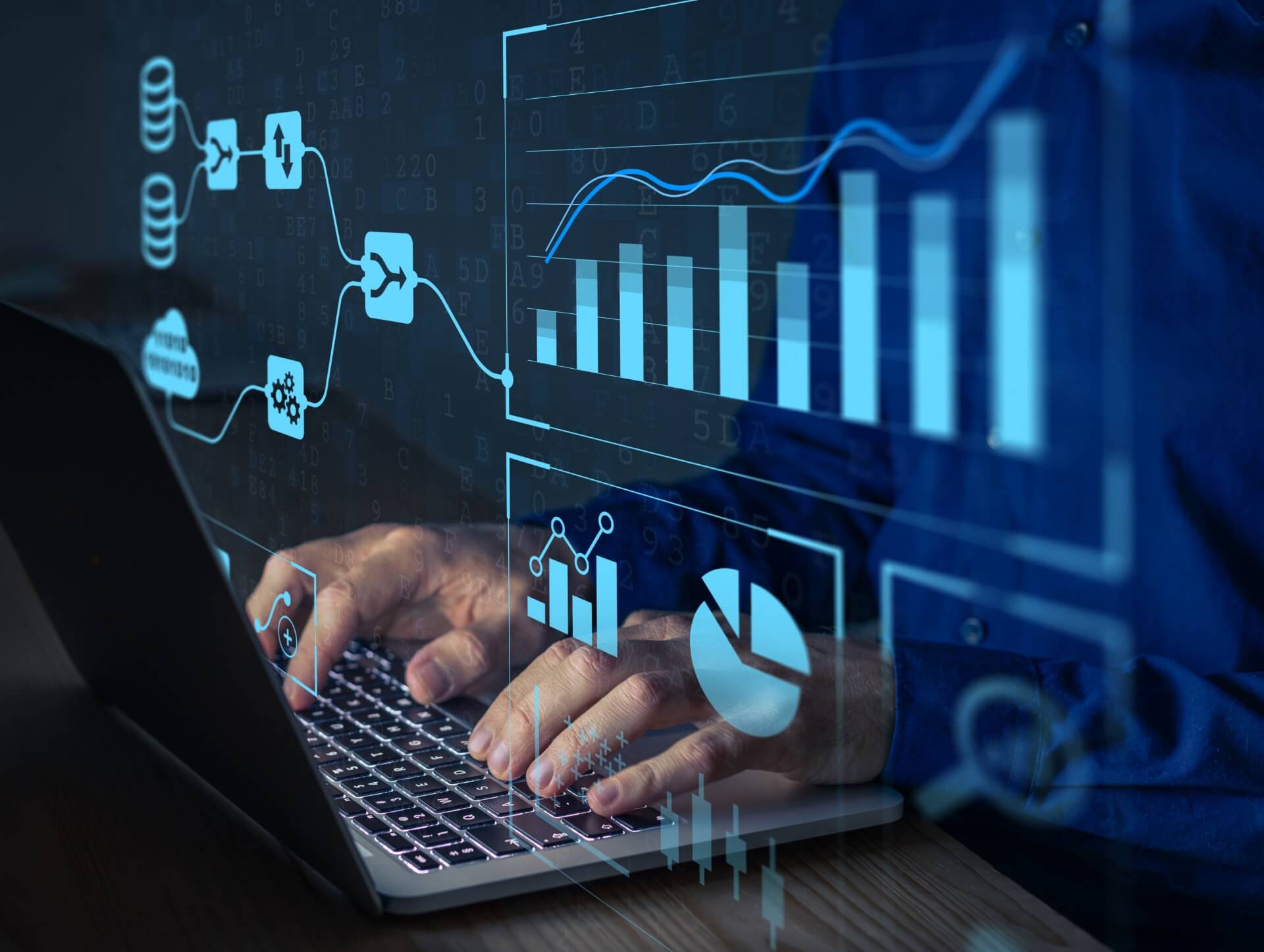
06/09/2023
Master Data Management (MDM) is a critical component of modern data-driven businesses. It involves the process of creating and managing a single, consistent, and accurate version of an organization's master data. Master data consists of core business entities such as customers, products, suppliers, and employees. In order to achieve effective MDM, data standardization is a key challenge that needs to be addressed.
What is Data Standardization?
Data standardization refers to the process of ensuring that data is consistently and uniformly structured, formatted, and labeled across different systems and applications. It involves defining and enforcing data standards, rules, and guidelines to ensure data consistency and quality. Standardized data is essential for accurate reporting, analytics, and decision-making.
The Importance of Data Standardization in MDM
Data standardization is crucial in MDM implementation for several reasons:
- Data Quality: Standardized data improves data quality by eliminating duplicates, inconsistencies, and errors. It ensures that data is accurate, complete, and up-to-date.
- Data Integration: Standardized data enables seamless integration of data from various sources and systems. It allows for data sharing and collaboration across departments and applications.
- Data Governance: Data standardization is a key aspect of data governance. It helps establish data policies, rules, and controls to ensure data compliance, privacy, and security.
- Data Analytics: Standardized data is essential for accurate and meaningful data analysis. It enables organizations to gain valuable insights, make informed decisions, and drive business growth.
Challenges in Data Standardization
Implementing data standardization in MDM can be challenging due to various factors:
- Data Complexity: Organizations deal with large volumes of complex data from multiple sources and systems. Standardizing this data requires a deep understanding of data structures, formats, and semantics.
- Data Variability: Data can vary in terms of formats, units, and coding schemes. Standardizing data across different systems and applications requires mapping and transformation of data elements.
- Data Governance: Data standardization requires strong data governance practices. Organizations need to establish data standards, policies, and procedures, and ensure compliance across the organization.
- Legacy Systems: Many organizations have legacy systems with outdated data formats and structures. Standardizing data from these systems requires data migration and transformation.
- System Integration: Integrating data from various systems and applications can be complex and time-consuming. Data standardization needs to be aligned with the integration process to ensure data consistency and quality.
- Change Management: Implementing data standardization requires change management efforts to overcome resistance from stakeholders and ensure adoption of standardized data practices.
Overcoming Data Standardization Challenges
To overcome the challenges of data standardization in MDM implementation, organizations can follow these best practices:
- Define Data Standards: Establish clear and comprehensive data standards, rules, and guidelines. Define data formats, structures, and naming conventions to ensure consistency and uniformity.
- Data Profiling and Analysis: Conduct data profiling and analysis to understand the quality and structure of existing data. Identify data inconsistencies, errors, and duplicates that need to be addressed.
- Data Mapping and Transformation: Develop data mapping and transformation rules to convert data from different formats and structures into a standardized format. Use data integration tools and technologies to automate this process.
- Data Governance and Stewardship: Establish a data governance framework to enforce data standards and policies. Appoint data stewards to ensure data compliance, privacy, and security.
- Data Quality Management: Implement data quality management processes and tools to monitor and improve data quality. Use data profiling, cleansing, and enrichment techniques to ensure standardized data.
- System Integration: Align data standardization efforts with system integration projects. Ensure that data standardization is part of the integration process and that data is validated and cleansed during integration.
- Change Management: Involve stakeholders from different departments and roles in the data standardization process. Communicate the benefits of standardized data and provide training and support to ensure adoption.
Conclusion
Data standardization is a critical challenge in MDM implementation. Standardized data is essential for data quality, integration, governance, and analytics. However, implementing data standardization can be complex due to data complexity, variability, legacy systems, and system integration. By following best practices such as defining data standards, conducting data profiling, mapping and transforming data, implementing data governance, and involving stakeholders in the process, organizations can overcome these challenges and achieve effective data standardization in MDM implementation.
Contact us
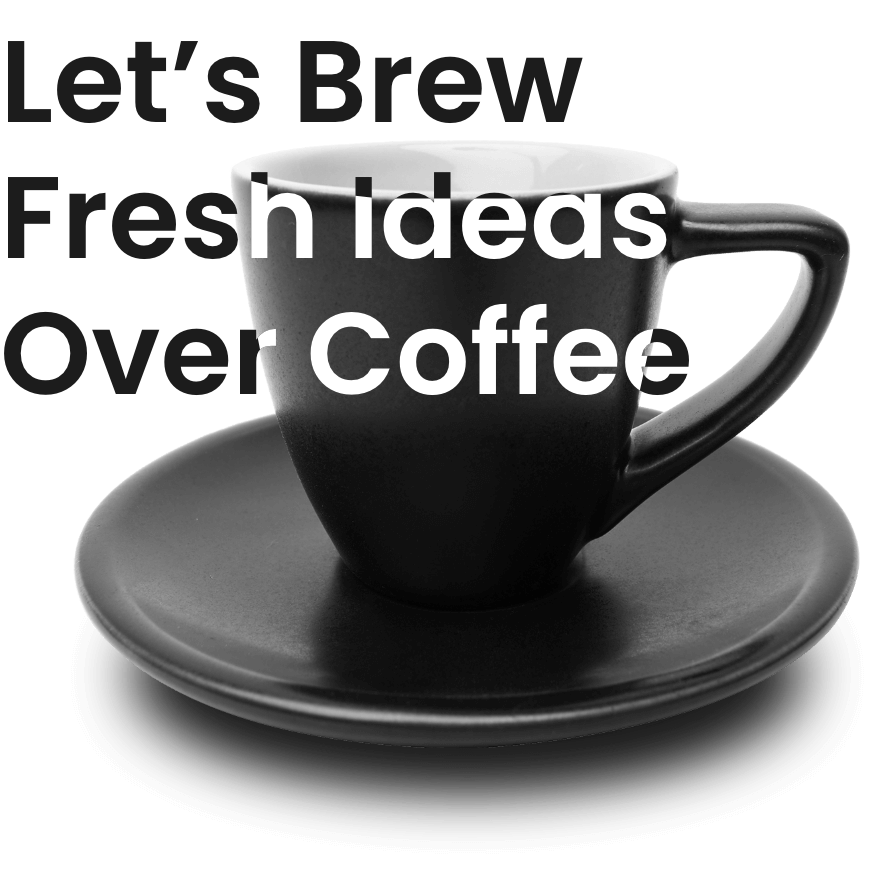
Spanning 8 cities worldwide and with partners in 100 more, we’re your local yet global agency.
Fancy a coffee, virtual or physical? It’s on us – let’s connect!