The challenges of managing master data in a data-driven organization
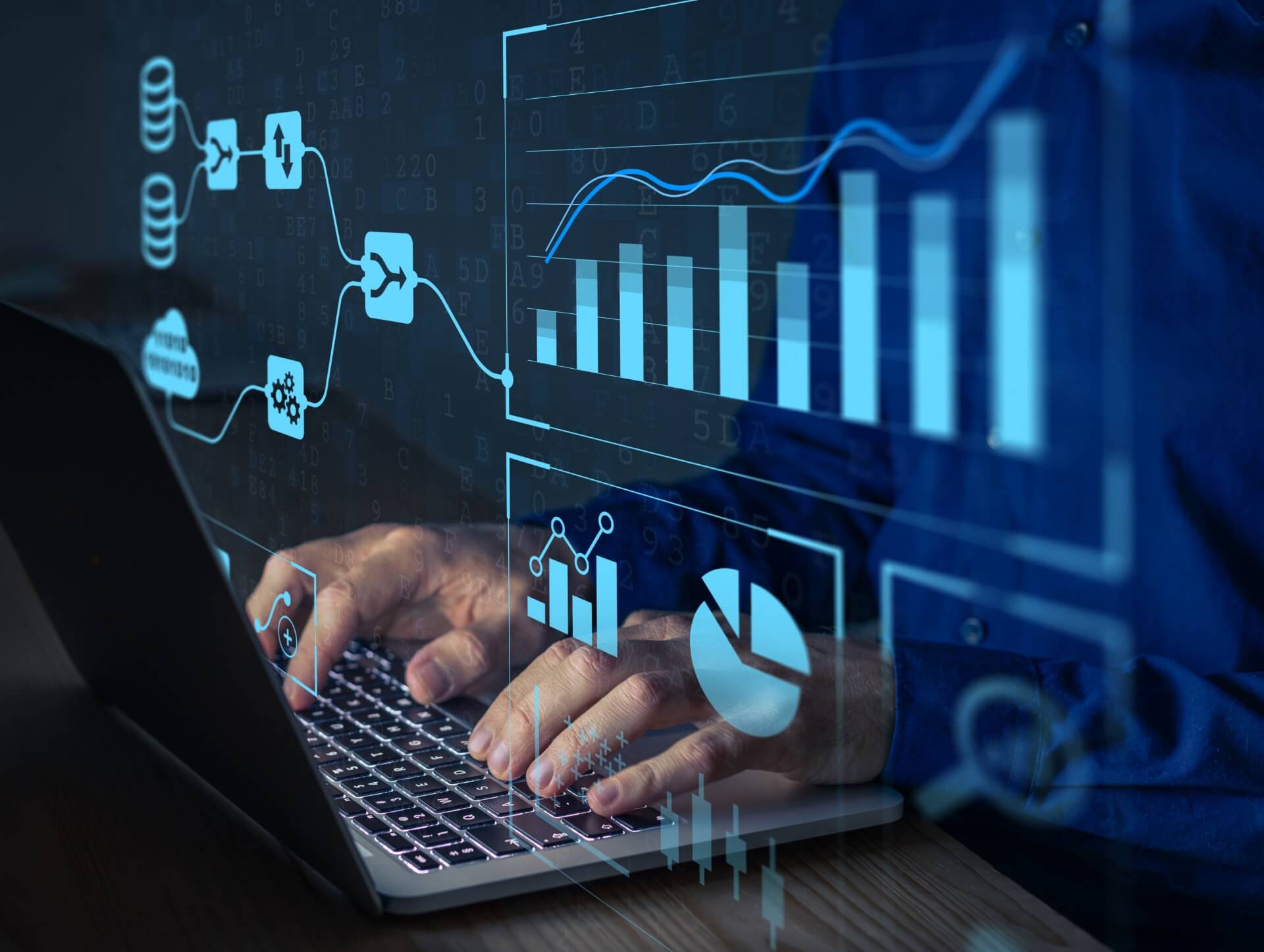
06/09/2023
Data has become the lifeblood of modern organizations. Whether it's customer data, product data, or any other type of data, organizations are relying on it to make informed decisions and gain a competitive edge. However, managing this data effectively can be a daunting task, especially when dealing with large volumes and multiple sources. This is where master data management (MDM) comes into play. In this article, we will explore the challenges faced in managing master data in a data-driven organization and how MDM solutions can help overcome them.
The Importance of Master Data Management
Before diving into the challenges, let's first understand the importance of master data management. Master data refers to the critical data entities that are essential for the smooth functioning of an organization. It includes data about customers, products, suppliers, employees, and other core entities. Without accurate and reliable master data, organizations may face several issues such as incorrect reporting, inefficient processes, and poor decision-making.
MDM provides a comprehensive approach to managing master data throughout its lifecycle. It involves creating a single, trusted source of master data that can be shared across different systems and applications. By implementing MDM, organizations can achieve data consistency, improve data quality, enhance data governance, and enable better data-driven decision-making.
The Challenges in Managing Master Data
While MDM offers numerous benefits, implementing and managing it can be challenging. Let's look at some of the common challenges faced by organizations:
Data Quality Management
One of the primary challenges in managing master data is ensuring data quality. Data quality refers to the accuracy, completeness, consistency, and timeliness of data. Poor data quality can lead to erroneous insights and decisions. Organizations need to establish data quality management processes and tools to identify and rectify data quality issues. This includes data cleansing, data enrichment, and data validation techniques.
Implementing data quality management practices requires a holistic approach that involves defining data quality rules, establishing data stewardship roles, and leveraging data profiling and monitoring tools. It is an ongoing process that requires continuous monitoring and improvement to maintain high-quality master data.
Data Governance in MDM
Data governance plays a crucial role in MDM. It involves defining policies, procedures, and guidelines for managing data assets. Data governance ensures that data is managed consistently, securely, and in accordance with regulatory requirements. However, establishing effective data governance in MDM can be challenging.
Organizations need to define data ownership, roles, and responsibilities, and establish a data governance framework. This includes creating data governance committees, implementing data governance tools, and conducting regular data governance audits. Data governance in MDM requires collaboration between business and IT stakeholders to ensure that data is governed effectively throughout its lifecycle.
MDM Strategy for Businesses
Developing an MDM strategy is essential for successful implementation and management. An MDM strategy outlines the goals, objectives, and roadmap for implementing MDM in an organization. It includes defining the scope of MDM, identifying the master data domains, and selecting the appropriate MDM tools and technologies.
Creating an MDM strategy requires a deep understanding of the organization's data landscape, business processes, and IT infrastructure. It involves conducting a thorough assessment of existing data management practices, identifying pain points, and defining key performance indicators (KPIs) for measuring the success of MDM initiatives. An effective MDM strategy aligns with the organization's business objectives and supports its data-driven initiatives.
MDM Implementation Process
The implementation process of MDM can be complex and time-consuming. It involves various stages such as data profiling, data cleansing, data integration, data modeling, and data migration. Each stage requires careful planning, coordination, and execution.
Organizations need to identify the right MDM solution that aligns with their requirements and budget. They need to consider factors such as scalability, flexibility, ease of use, and integration capabilities. The implementation process also involves defining data mapping rules, establishing data workflows, and configuring data validation and enrichment rules.
During the implementation process, organizations may face challenges such as data migration issues, integration complexities, and resistance to change. It is important to have a well-defined implementation plan, engage stakeholders, and provide adequate training and support to ensure a smooth transition to the MDM solution.
Master Data Governance
Master data governance involves defining policies, processes, and standards for managing master data. It ensures that master data is accurate, consistent, and up-to-date. However, governing master data can be challenging, especially in organizations with multiple data sources and systems.
Organizations need to establish data stewardship roles and responsibilities, define data governance workflows, and implement data governance tools. They need to enforce data governance policies and procedures across the organization and monitor compliance. Master data governance requires collaboration between business and IT stakeholders to ensure that master data is governed effectively throughout its lifecycle.
MDM Tools and Technologies
Choosing the right MDM tools and technologies is crucial for the success of MDM initiatives. There are various MDM solutions available in the market, ranging from open source to cloud-based platforms. Organizations need to evaluate their requirements, budget, and IT infrastructure before selecting an MDM solution.
MDM tools should have features such as data integration, data cleansing, data modeling, data governance, and data analytics. They should be easy to use, scalable, and provide a comprehensive view of master data. Organizations also need to consider factors such as vendor support, product roadmap, and future-proofing their MDM investments.
Integration Challenges
Data integration is a critical aspect of MDM. It involves consolidating data from various sources and systems to create a single, unified view of master data. However, integrating data from diverse sources can be challenging.
Organizations need to consider data integration techniques such as extract, transform, load (ETL), application programming interfaces (APIs), and data virtualization. They need to define data mapping rules, resolve data conflicts, and establish data synchronization processes. Data integration challenges include data inconsistencies, data duplication, and data latency.
Security and Privacy
Data security and privacy are paramount in MDM. Organizations need to ensure that master data is protected against unauthorized access, data breaches, and data leaks. They need to define data security policies, implement access controls, and encrypt sensitive data.
Organizations also need to comply with data privacy regulations such as the General Data Protection Regulation (GDPR) and Health Insurance Portability and Accountability Act (HIPAA). This includes obtaining consent for data collection, providing data subjects with rights to access and delete their data, and implementing data anonymization techniques.
Data Analytics and Insights
MDM provides a solid foundation for data analytics and insights. By having accurate and reliable master data, organizations can leverage advanced analytics techniques to gain valuable insights and make data-driven decisions. However, organizations may face challenges in deriving meaningful insights from master data.
They need to have the right analytics tools and technologies that can handle large volumes of data and perform complex analytics tasks. They need to define analytics use cases, establish data governance for analytics, and enable self-service analytics capabilities. Data analytics challenges include data silos, data quality issues, and lack of analytical skills.
Customer Experience
MDM plays a crucial role in improving the customer experience. By having a 360-degree view of customers, organizations can personalize their interactions, provide better customer service, and enhance customer satisfaction. However, achieving a single view of the customer can be challenging.
Organizations need to integrate customer data from various touchpoints such as CRM systems, marketing automation platforms, and e-commerce platforms. They need to resolve data conflicts, eliminate duplicate records, and update customer profiles in real-time. Customer experience challenges include data inconsistencies, data latency, and lack of data integration capabilities.
Conclusion
Managing master data in a data-driven organization comes with its own set of challenges. However, with the right master data management solutions and strategies in place, organizations can overcome these challenges and unlock the full potential of their data. By ensuring data quality, establishing data governance, implementing the right tools and technologies, and leveraging data analytics, organizations can achieve a single, trusted source of master data that drives better decision-making and business outcomes.
Contact us
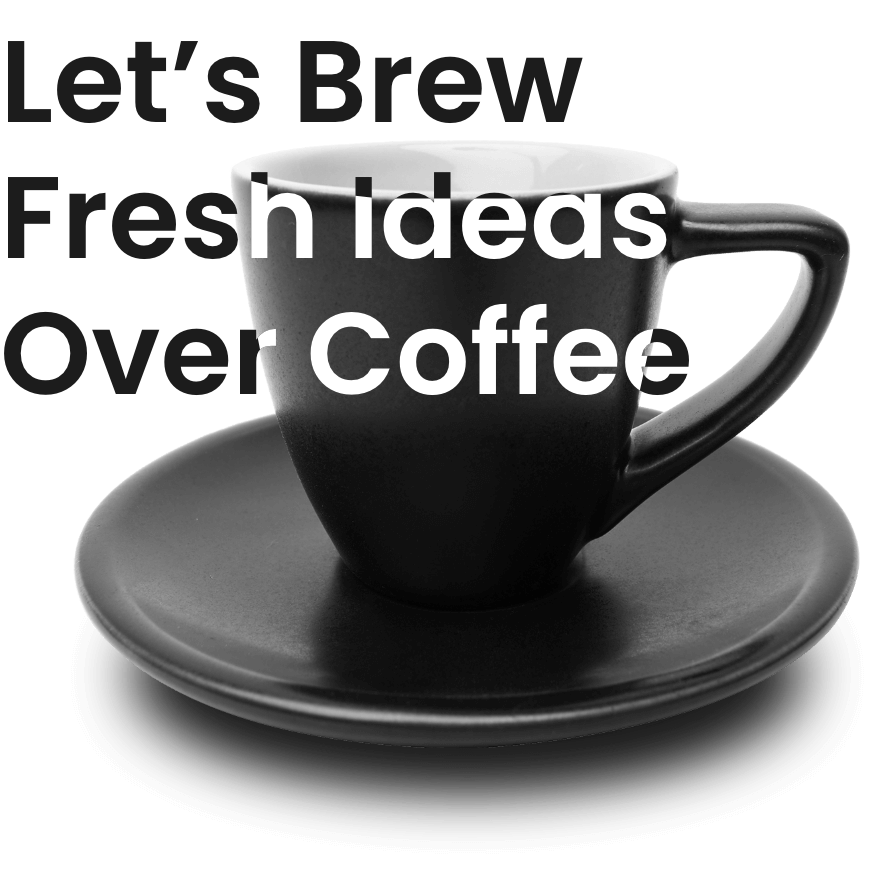
Spanning 8 cities worldwide and with partners in 100 more, we’re your local yet global agency.
Fancy a coffee, virtual or physical? It’s on us – let’s connect!