The challenges of scaling MDM in global enterprises with diverse data sources
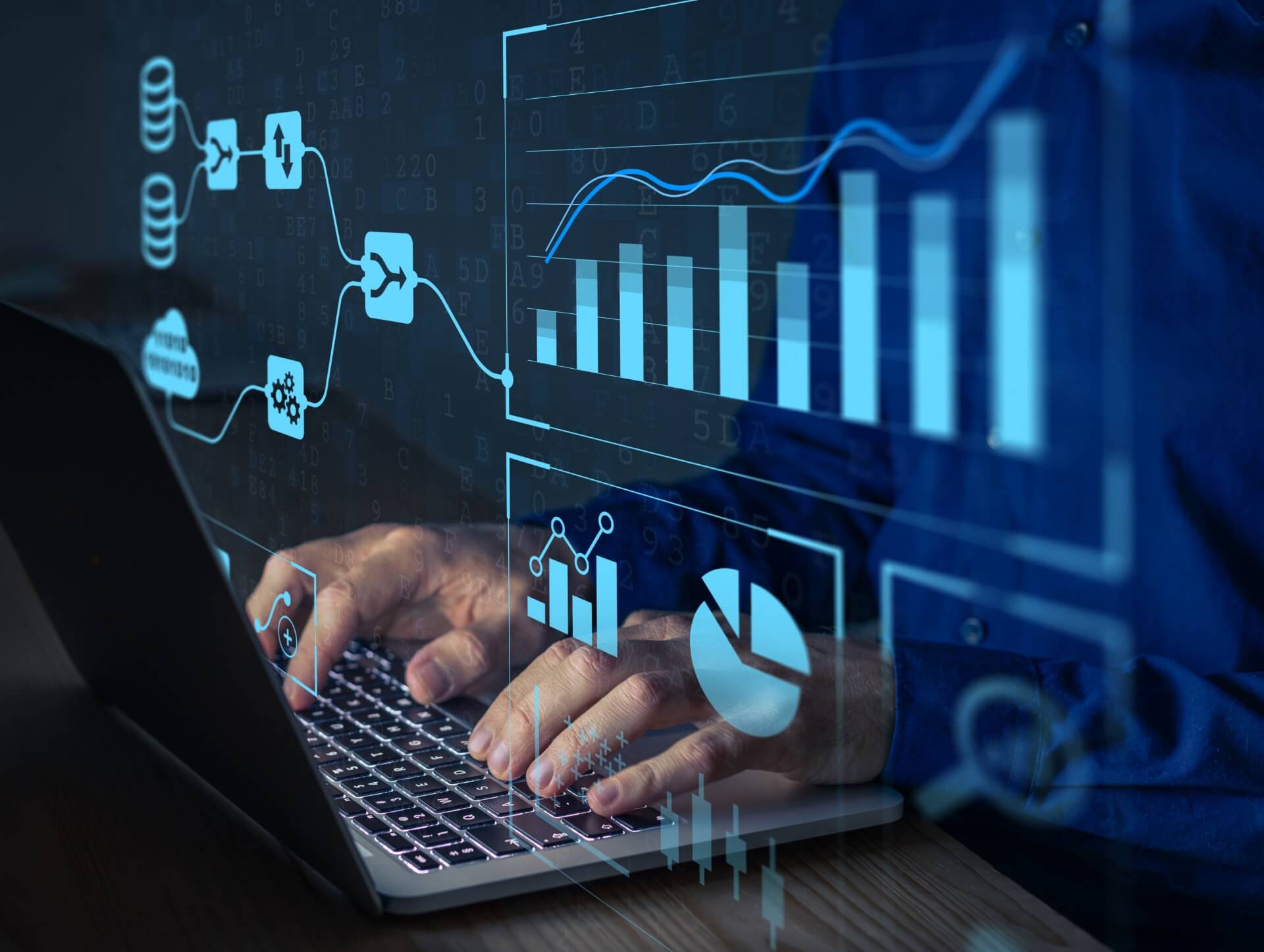
06/09/2023
Master Data Management (MDM) is a crucial aspect of data governance in modern enterprises. It involves the management and consolidation of an organization's critical data to ensure data accuracy, consistency, and integrity across various systems and applications. MDM solutions provide a centralized platform for managing master data, such as customer information, product data, and employee records, to enable better decision-making, operational efficiency, and regulatory compliance.
As enterprises expand globally and accumulate diverse data sources, scaling MDM becomes a challenging endeavor. This article explores the challenges faced by global enterprises in scaling MDM and provides insights into overcoming these challenges.
Challenges in scaling MDM
Scaling MDM in global enterprises comes with its own set of challenges. Let's explore some of the key challenges:
1. Data integration in MDM
One of the primary challenges in scaling MDM is integrating data from diverse sources. Global enterprises often have multiple systems, applications, and databases that store master data. These data sources may use different formats, structures, and standards, making data integration a complex task. Data integration involves mapping, transforming, and harmonizing data from various sources to create a unified view of master data.
To overcome this challenge, organizations need to invest in robust data integration tools and technologies. These tools can automate the data integration process, reducing manual effort and ensuring data consistency. Additionally, establishing data governance practices and standards can help streamline the data integration process and ensure data quality.
2. Data quality management
Data quality is crucial for the success of any MDM initiative. Poor data quality can lead to inaccurate insights, inefficient processes, and compliance issues. Ensuring data quality becomes even more challenging in global enterprises with diverse data sources. Data quality issues can arise due to inconsistent data formats, duplicate records, missing values, or outdated information.
To address this challenge, organizations need to implement robust data quality management practices. This includes data profiling, data cleansing, and data enrichment techniques. Automated data quality tools can help identify and rectify data quality issues, ensuring high-quality master data for decision-making and operational efficiency.
3. Data governance in MDM
Data governance is a critical component of MDM. It involves defining policies, procedures, and responsibilities for managing and ensuring the quality, availability, and security of data. Implementing effective data governance becomes challenging in global enterprises where data is spread across multiple geographies, business units, and systems.
To overcome this challenge, organizations need to establish a robust data governance framework that defines data ownership, data stewardship, and data management processes. This framework should be supported by technology solutions that enable data governance, such as data governance tools and metadata management systems.
4. MDM and data security
Data security is a paramount concern for global enterprises, especially when it comes to managing master data. Master data often includes sensitive information, such as customer details, financial data, and intellectual property. Ensuring data security becomes challenging in a global MDM environment due to the need to comply with different data protection regulations and the presence of multiple access points.
To address this challenge, organizations need to implement robust data security measures throughout the MDM lifecycle. This includes data encryption, access control mechanisms, data masking, and regular security audits. Additionally, organizations should ensure compliance with relevant data protection regulations, such as GDPR and CCPA, to protect the privacy rights of individuals.
Overcoming the challenges
While scaling MDM in global enterprises with diverse data sources may seem daunting, there are several strategies and best practices that can help overcome these challenges:
1. Define a comprehensive MDM strategy
A well-defined MDM strategy is essential for successful MDM implementation. The strategy should outline the goals, objectives, and scope of the MDM initiative. It should also consider the specific challenges and requirements of scaling MDM in a global enterprise. The strategy should be aligned with the organization's overall data strategy and business objectives.
2. Invest in the right MDM tools and technologies
Choosing the right MDM platform is crucial for scaling MDM. Organizations should evaluate different MDM solutions and select the one that best fits their requirements. This includes considering factors such as data integration capabilities, data quality management features, scalability, and flexibility. Open-source MDM solutions and cloud-based MDM platforms are gaining popularity due to their cost-effectiveness and scalability.
3. Establish a strong data governance framework
A robust data governance framework is essential for successful MDM implementation. The framework should define clear roles, responsibilities, and processes for data management. It should also ensure compliance with data protection regulations and industry standards. Regular data governance audits and reviews can help identify and address any gaps or issues in the data governance framework.
4. Implement data integration and data quality management processes
Effective data integration and data quality management processes are crucial for scaling MDM. Organizations should invest in data integration tools and technologies that can automate the data integration process and ensure data consistency. Similarly, implementing data profiling, data cleansing, and data enrichment techniques can help improve data quality and accuracy.
5. Ensure data security throughout the MDM lifecycle
Data security should be a top priority in MDM implementation. Organizations should implement robust data security measures, such as encryption, access control, and data masking, to protect master data from unauthorized access or breaches. Regular security audits and vulnerability assessments can help identify and address any security vulnerabilities.
6. Leverage data analytics for insights
Data analytics plays a crucial role in deriving insights from master data. By leveraging analytics tools and techniques, organizations can uncover patterns, trends, and correlations within their master data. This can help in making informed business decisions, identifying new revenue opportunities, and improving customer experience.
Conclusion
Scaling MDM in global enterprises with diverse data sources is a complex task, but with the right strategies and best practices, it can be overcome. By investing in the right MDM tools and technologies, establishing a strong data governance framework, implementing data integration and data quality management processes, ensuring data security throughout the MDM lifecycle, and leveraging data analytics for insights, organizations can successfully scale MDM and reap the benefits of accurate, consistent, and reliable master data.
Contact us
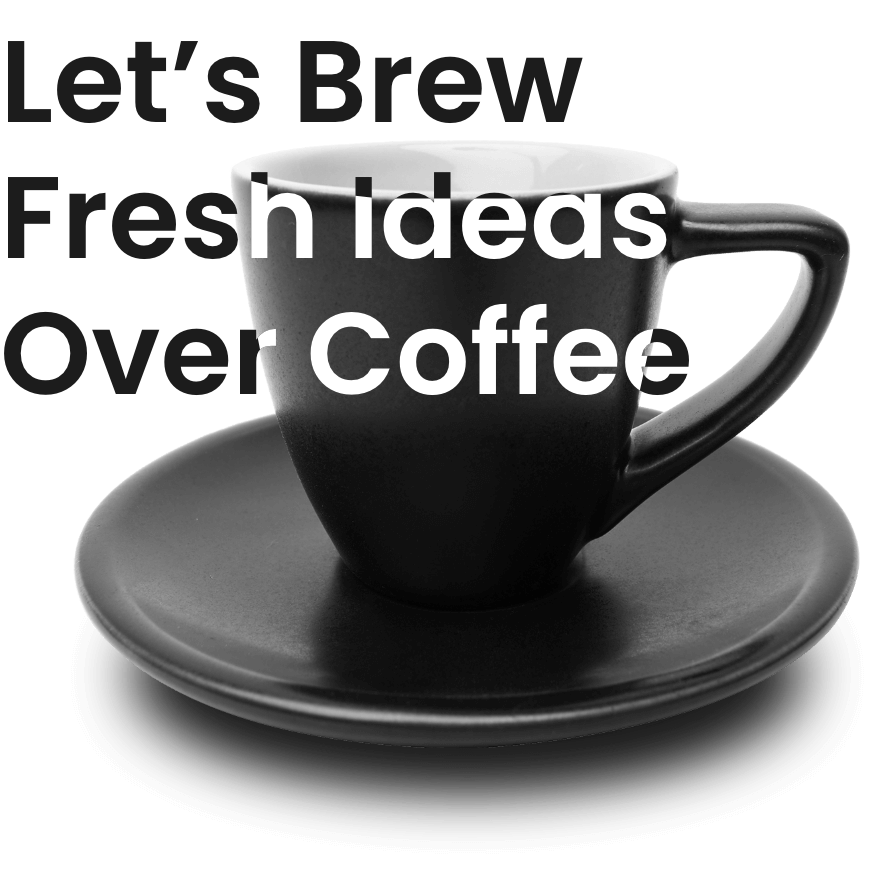
Spanning 8 cities worldwide and with partners in 100 more, we’re your local yet global agency.
Fancy a coffee, virtual or physical? It’s on us – let’s connect!