The challenges of scaling MDM in organizations with data-intensive energy operations
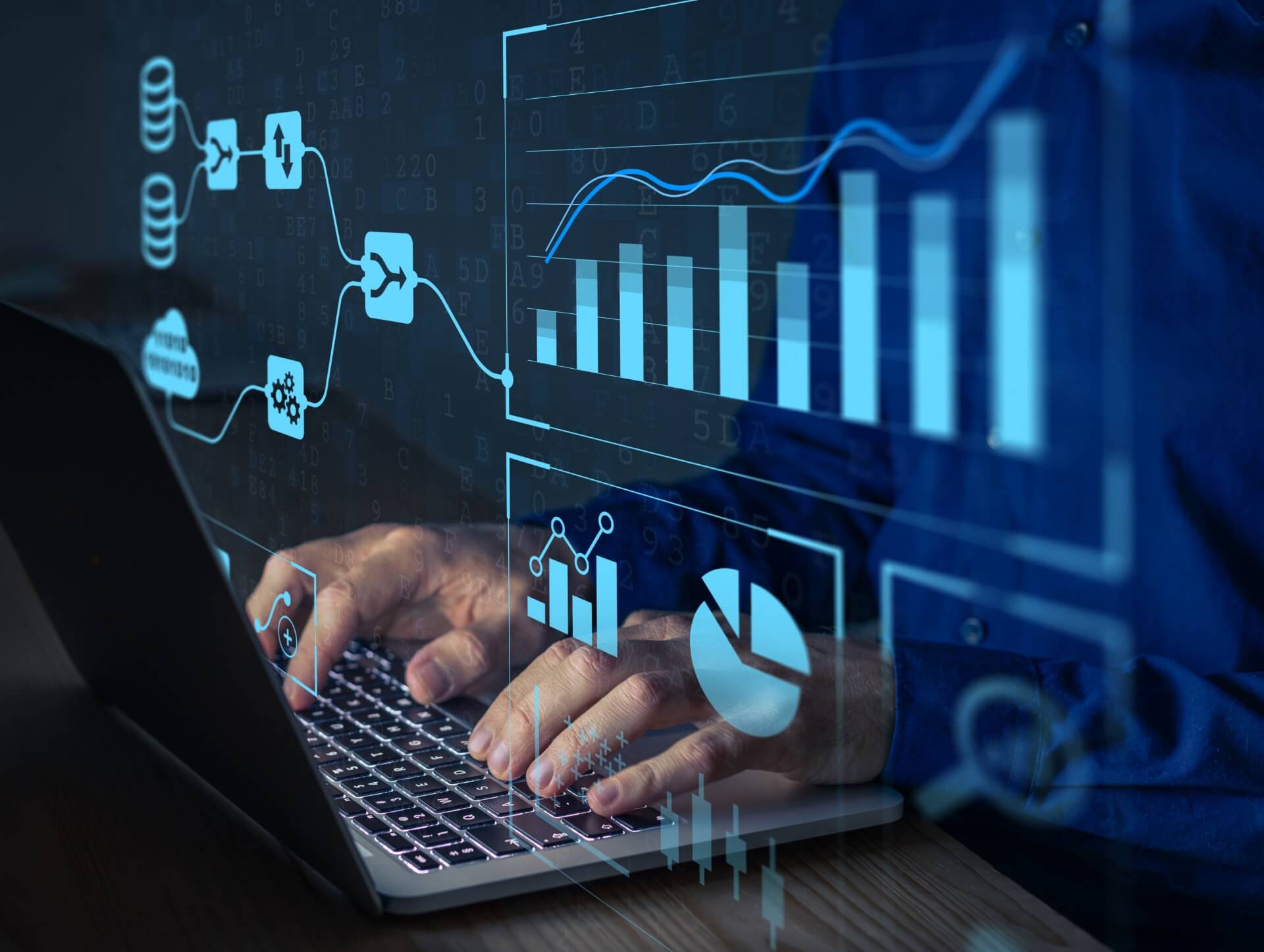
06/09/2023
Master Data Management (MDM) is a critical process for organizations with data-intensive energy operations. It involves the management and governance of the master data, which includes data about customers, products, assets, suppliers, and more. MDM ensures data consistency, accuracy, and reliability across the organization, enabling better decision-making and operational efficiency.
However, scaling MDM in organizations with data-intensive energy operations can present several challenges. In this article, we will explore these challenges and discuss best practices and strategies to overcome them.
Challenges in Scaling MDM
1. Data Volume and Complexity:
One of the primary challenges in scaling MDM in organizations with data-intensive energy operations is the sheer volume and complexity of the data. Energy operations generate vast amounts of data from various sources, such as smart meters, sensors, IoT devices, and more. This data is often unstructured and needs to be processed and integrated with other data sources.
Organizations need to invest in robust MDM solutions that can handle the volume and complexity of their data. These solutions should have advanced data integration capabilities to consolidate data from multiple sources and ensure data quality and accuracy.
2. Data Quality Management:
Ensuring data quality is crucial for effective MDM. In energy operations, data quality issues can have significant consequences, leading to operational inefficiencies, regulatory non-compliance, and financial losses.
Organizations need to implement robust data quality management processes as part of their MDM strategy. This includes data cleansing, validation, enrichment, and ongoing monitoring. By improving data quality, organizations can rely on accurate and trustworthy data for their operations.
3. Data Governance:
Data governance plays a vital role in MDM, especially in organizations with data-intensive energy operations. It involves defining data ownership, accountability, and access controls to ensure data consistency and compliance.
Organizations need to establish a data governance framework that aligns with their MDM goals. This framework should include clear roles and responsibilities, data policies and standards, and a robust data governance toolset. By implementing effective data governance practices, organizations can overcome challenges related to data ownership and data consistency.
4. Data Security and Privacy:
Data security and privacy are critical concerns in organizations with data-intensive energy operations. Energy-related data often contains sensitive information, such as customer details, financial data, and operational insights.
Organizations need to implement robust data security measures to protect their master data. This includes encryption, access controls, user authentication, and regular security audits. Additionally, organizations should ensure compliance with relevant data privacy regulations, such as GDPR or CCPA.
Best Practices for Scaling MDM
1. Define a Clear MDM Strategy:
A well-defined MDM strategy is crucial for successful scaling. Organizations need to clearly define their MDM objectives, identify the key data domains, and prioritize data management initiatives.
2. Invest in the Right MDM Tools and Technologies:
Choosing the right MDM tools and technologies is essential for scaling MDM. Organizations should evaluate different MDM platforms and select the one that aligns with their requirements.
3. Adopt a Data-Driven Culture:
A data-driven culture is essential for successful MDM implementation. Organizations should promote data literacy and encourage employees to make data-driven decisions.
4. Establish Data Stewardship Roles:
Data stewardship roles are critical for effective MDM. Organizations should identify individuals who will be responsible for data governance, data quality, and data management.
Case Studies
1. Organization A:
Organization A is a leading energy company with data-intensive operations. They faced challenges in scaling their MDM processes due to the large volume and complexity of their data.
By implementing a cloud-based MDM solution and adopting data governance best practices, Organization A was able to overcome these challenges. They achieved improved data quality, streamlined data integration, and enhanced operational efficiency.
2. Organization B:
Organization B is a renewable energy company with multi-cloud environments. They struggled with data governance and data compliance in their MDM processes.
By implementing a comprehensive data governance framework and leveraging MDM tools with built-in compliance features, Organization B successfully addressed these challenges. They achieved data consistency, compliance with regulatory requirements, and minimized data security risks.
Conclusion
Scaling MDM in organizations with data-intensive energy operations can be challenging, but with the right strategies and best practices, these challenges can be overcome. By investing in robust MDM solutions, implementing data governance frameworks, ensuring data quality and security, and fostering a data-driven culture, organizations can achieve efficient and effective MDM at scale.
Read
More Stories
Contact us
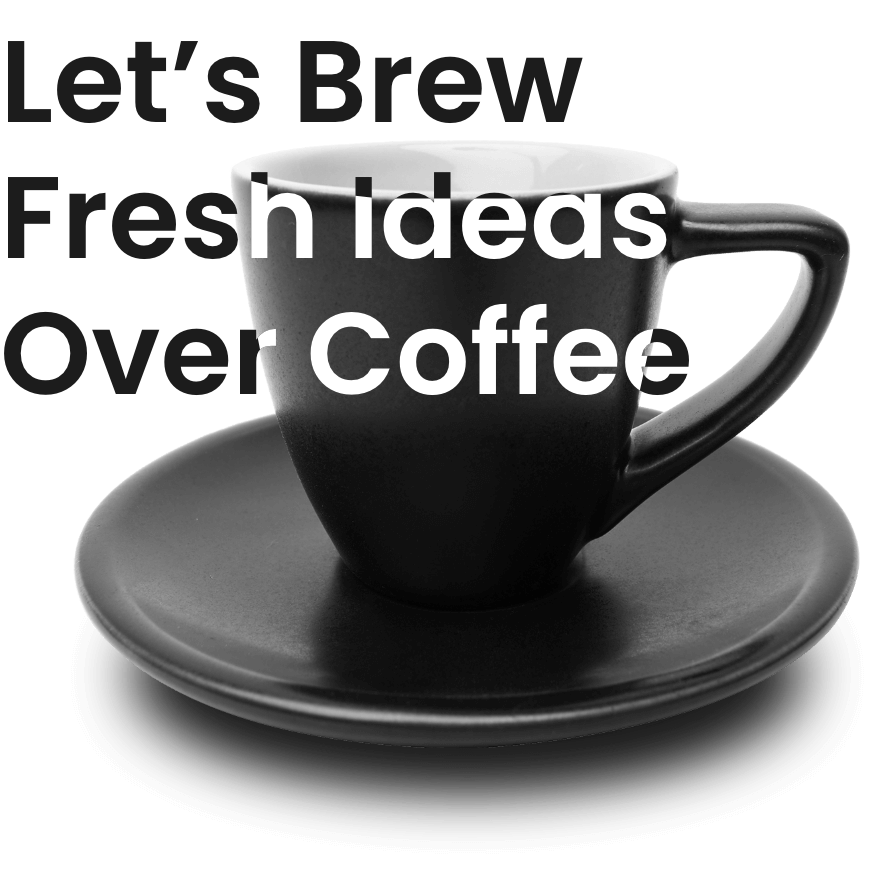
Spanning 8 cities worldwide and with partners in 100 more, we’re your local yet global agency.
Fancy a coffee, virtual or physical? It’s on us – let’s connect!