The challenges of scaling MDM in organizations with diverse data formats and structures
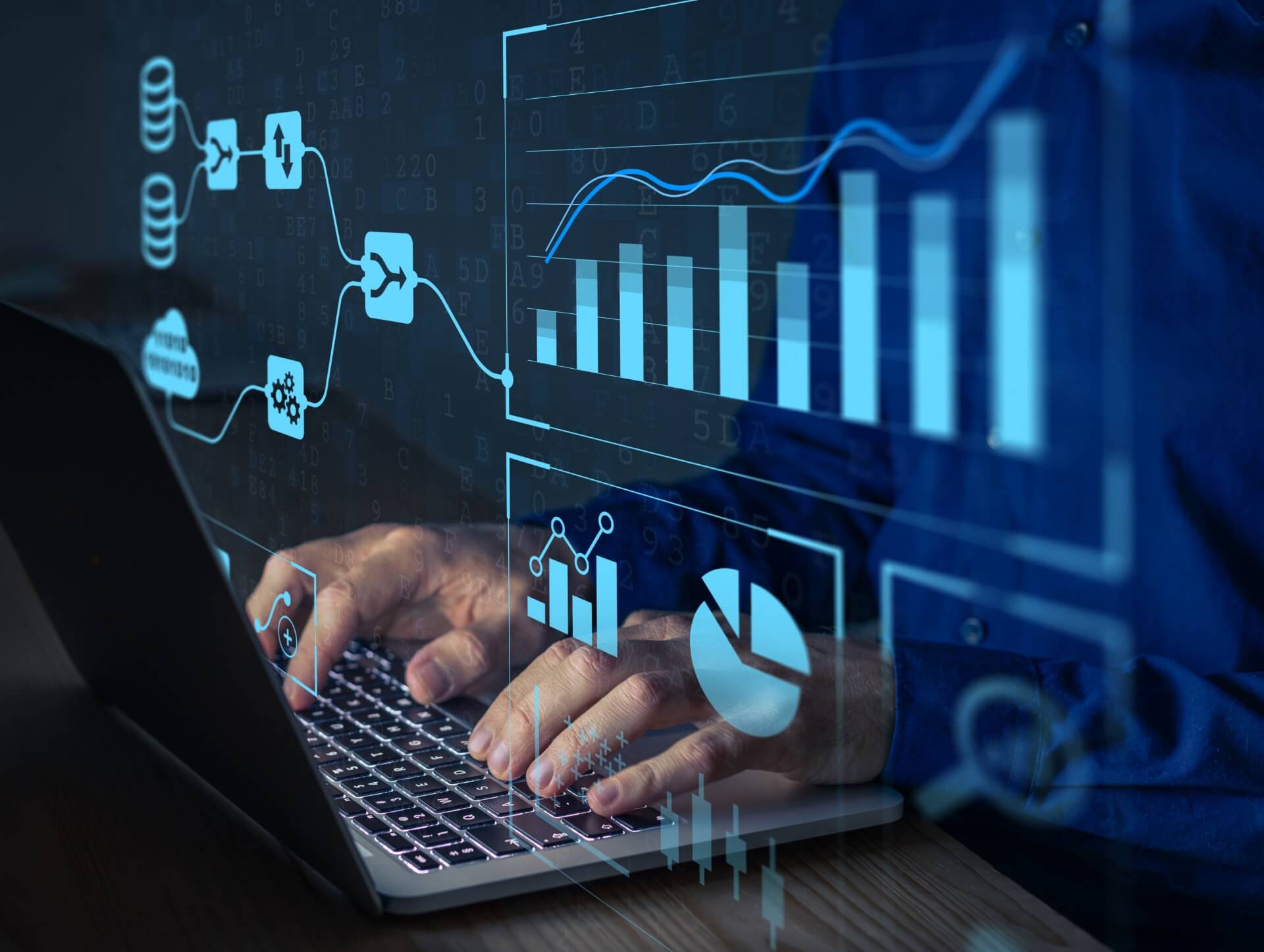
06/09/2023
Master Data Management (MDM) is a crucial process for organizations looking to effectively manage and utilize their data assets. It involves the consolidation, cleansing, and synchronization of data from multiple sources to create a single, reliable version of the truth. However, scaling MDM in organizations with diverse data formats and structures presents unique challenges that need to be addressed for successful implementation and utilization of MDM solutions.
The Importance of MDM in Today's Data-Driven World
In today's data-driven world, organizations need to make informed decisions based on accurate and reliable data. MDM plays a vital role in ensuring data quality, consistency, and reliability across different systems and business functions. By implementing a robust MDM strategy, organizations can achieve a unified view of their data, improve data governance, and enable better decision-making.
The Challenges of Scaling MDM
Scaling MDM in organizations with diverse data formats and structures presents several challenges that need to be addressed. These challenges include:
Data Integration
One of the major challenges in scaling MDM is integrating data from various sources with different formats and structures. Organizations often have data stored in different systems, databases, and file formats, making it difficult to consolidate and synchronize the data for MDM purposes. This requires careful planning and the use of appropriate tools and technologies to ensure seamless data integration.
Data Quality Management
Poor data quality can significantly impact the effectiveness of MDM. In organizations with diverse data formats and structures, ensuring data quality becomes even more challenging. Data may have inconsistencies, errors, and duplicates, which can lead to inaccurate insights and decisions. Implementing data quality management processes and tools is essential for addressing these challenges and improving the overall data quality in MDM.
Data Governance
Data governance is crucial for maintaining data integrity, security, and compliance throughout the MDM process. However, in organizations with diverse data formats and structures, establishing and enforcing data governance policies becomes more complex. It requires defining data governance frameworks, roles, and responsibilities, and implementing tools and technologies to ensure compliance and data security.
Master Data Modeling
Master data modeling is a critical aspect of MDM that involves defining the structure, relationships, and attributes of master data entities. In organizations with diverse data formats and structures, developing a comprehensive and flexible master data model becomes challenging. It requires understanding the different data formats and structures, mapping them to a common model, and accommodating future changes and additions.
Best Practices for Scaling MDM in Organizations
While scaling MDM in organizations with diverse data formats and structures may pose challenges, following best practices can help overcome these challenges and ensure successful implementation and utilization of MDM solutions:
Data Profiling and Analysis
Prior to implementing MDM, organizations should conduct data profiling and analysis to understand the quality, consistency, and structure of their data. This helps identify potential challenges and areas for improvement, allowing organizations to develop appropriate strategies and solutions.
Data Standardization
Standardizing data formats and structures is crucial for successful MDM implementation. Organizations should define data standards and guidelines that ensure consistency and compatibility across different systems and sources. This includes standardizing data attributes, naming conventions, and data validation rules.
Data Mapping and Transformation
Data mapping and transformation is a key step in integrating and synchronizing data from diverse sources. Organizations should invest in tools and technologies that enable efficient data mapping and transformation, allowing them to convert data from different formats and structures into a common format that can be used for MDM.
Data Governance Framework
Establishing a robust data governance framework is essential for ensuring data integrity, security, and compliance in MDM. This framework should define clear data governance policies, roles, and responsibilities, and should be supported by appropriate tools and technologies that enable data governance processes.
Continuous Data Quality Management
Data quality management should be an ongoing process in MDM. Organizations should implement data quality management tools and processes that enable continuous monitoring, profiling, and improvement of data quality. This includes identifying and resolving data quality issues, and implementing data quality metrics and KPIs.
Change Management
Implementing MDM in organizations with diverse data formats and structures requires effective change management. Organizations should develop change management strategies that involve training, communication, and stakeholder engagement to ensure smooth adoption and utilization of MDM solutions.
Case Studies: Successful MDM Implementation in Organizations with Diverse Data Formats and Structures
Several organizations have successfully implemented MDM solutions in environments with diverse data formats and structures. These case studies highlight the benefits and challenges of scaling MDM:
Case Study 1: Company XYZ
Company XYZ, a multinational corporation, faced the challenge of consolidating customer data from multiple sources, including CRM systems, ERP systems, and external data providers. By implementing an MDM solution, Company XYZ was able to achieve a single, unified view of customer data, resulting in improved customer service, targeted marketing campaigns, and increased sales.
Case Study 2: Organization ABC
Organization ABC, a healthcare provider, had data stored in various formats and structures across different departments and systems. By implementing an MDM platform, Organization ABC was able to standardize data formats, streamline data integration, and improve data quality. This enabled the organization to make better-informed decisions and improve patient care.
Case Study 3: Company DEF
Company DEF, a retail organization, faced the challenge of managing product data from multiple suppliers with different data formats and structures. By implementing an MDM solution, Company DEF was able to streamline product data management, improve data accuracy, and enhance the customer experience. This resulted in increased sales and customer satisfaction.
The Future of MDM in Organizations with Diverse Data Formats and Structures
As organizations continue to generate and collect vast amounts of data, the need for effective MDM solutions will only increase. Advancements in technologies such as artificial intelligence, machine learning, and automation will play a crucial role in addressing the challenges of scaling MDM in organizations with diverse data formats and structures.
Conclusion
Scaling MDM in organizations with diverse data formats and structures may present challenges, but by following best practices and leveraging appropriate tools and technologies, organizations can overcome these challenges and reap the benefits of effective MDM. With a unified view of data, improved data quality, and enhanced decision-making capabilities, organizations can gain a competitive advantage in today's data-driven world.
Contact us
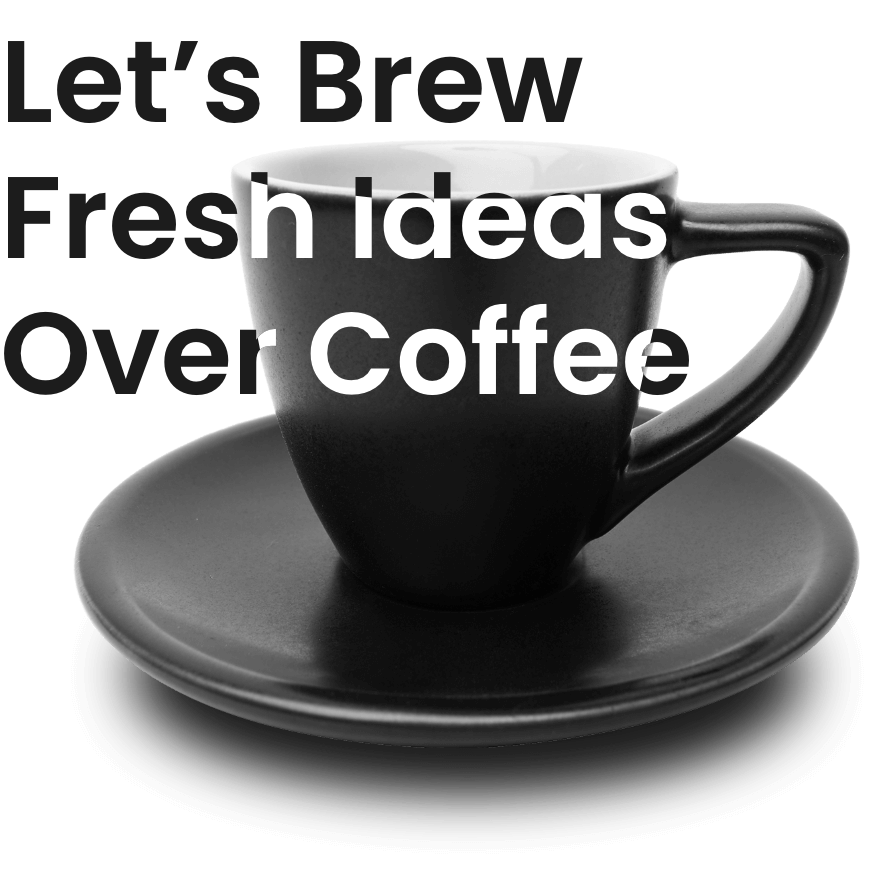
Spanning 8 cities worldwide and with partners in 100 more, we’re your local yet global agency.
Fancy a coffee, virtual or physical? It’s on us – let’s connect!