The ethical implications of data quality management
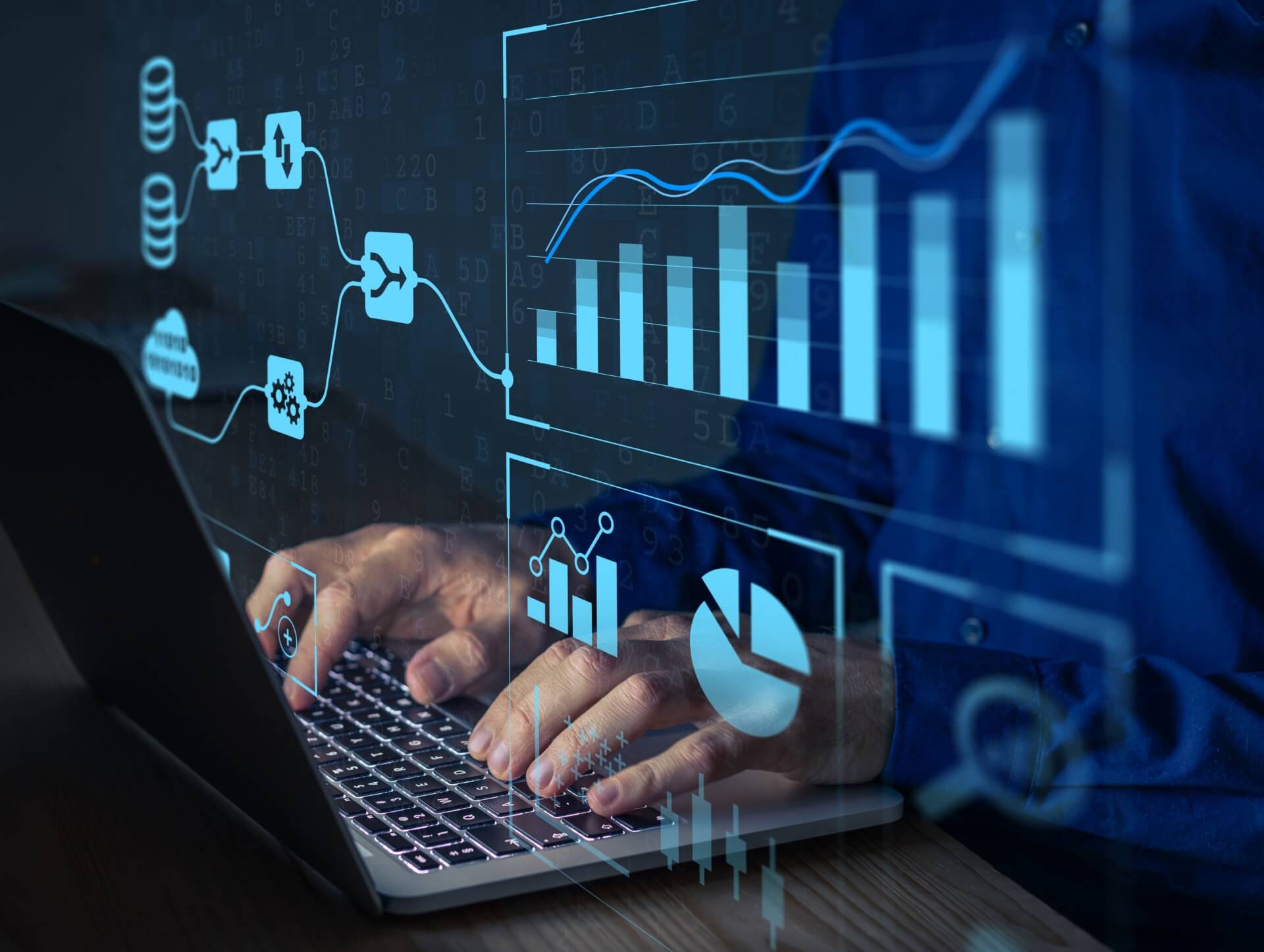
06/09/2023
Data quality management is a crucial aspect of any organization's data strategy. It involves the processes and methodologies used to ensure the accuracy, consistency, and reliability of data throughout its lifecycle. In today's data-driven world, where organizations rely heavily on data for decision-making, data quality management plays a vital role in ensuring that the data used is trustworthy and meets the required standards.
Why Data Quality Management is Important
Effective data quality management is essential for several reasons:
- Trustworthy Decision-Making: High-quality data ensures that decisions made based on that data are reliable and accurate. Organizations heavily rely on data-driven insights to make informed decisions, and if the underlying data is of poor quality, the decisions made may be flawed.
- Operational Efficiency: Poor data quality can lead to inefficiencies in business operations. Inaccurate or inconsistent data can result in delays, errors, and additional costs in various processes, such as order fulfillment, customer service, and financial reporting.
- Regulatory Compliance: Many industries have strict regulations regarding data quality and privacy. Ensuring data quality is not only important for internal operations but also for complying with legal and regulatory requirements.
The Ethical Implications of Poor Data Quality
Poor data quality can have significant ethical implications for organizations:
- Misleading Decision-Making: If data quality is compromised, decision-makers may unknowingly rely on inaccurate or incomplete information, leading to poor decision-making. This can have serious consequences for both the organization and its stakeholders.
- Unfair Treatment of Customers: Inaccurate customer data can result in unfair treatment of customers. For example, if a customer's information is incorrectly recorded, they may receive incorrect billing statements or be denied access to certain services. This can lead to customer dissatisfaction and potential legal issues.
- Privacy Violations: Poor data quality can also lead to privacy violations. If sensitive customer information is not properly secured or is shared with unauthorized individuals, it can result in breaches of privacy regulations and harm to individuals whose data is compromised.
- Unintended Discrimination: Biases and discriminatory practices can be inadvertently perpetuated if data quality issues are not addressed. For example, if certain demographic data is consistently inaccurate or incomplete, it may result in biased decision-making that disproportionately affects certain groups.
Best Practices for Data Quality Management
Implementing effective data quality management requires a comprehensive strategy and adherence to best practices:
- Data Governance: Establishing a robust data governance framework is crucial for ensuring data quality. This involves defining data ownership, establishing data quality standards, and implementing processes for data validation and cleansing.
- Data Profiling: Conducting data profiling helps identify data quality issues and inconsistencies. By analyzing the structure, content, and relationships within the data, organizations can gain insights into the overall quality of their data and take appropriate actions to resolve any identified issues.
- Data Cleansing: Regular data cleansing activities, such as removing duplicate records, correcting inaccuracies, and standardizing data formats, are essential for maintaining data quality. Automated tools and technologies can be used to streamline the data cleansing process.
- Data Integration: Integrating data from various sources can pose challenges to data quality. Implementing robust data integration processes and ensuring data consistency across different systems can help maintain data quality throughout the organization.
- Data Quality Metrics: Defining and tracking data quality metrics is important for measuring the effectiveness of data quality management efforts. Metrics such as data accuracy, completeness, and timeliness can provide insights into the overall health of an organization's data.
The Role of Data Governance in Data Quality Management
Data governance plays a crucial role in ensuring data quality. It encompasses the policies, processes, and technologies used to define and enforce data-related standards and practices within an organization. Effective data governance provides the framework for data quality management by:
- Defining Data Quality Standards: Data governance establishes the standards and guidelines for data quality, ensuring that all data within the organization meets the required level of accuracy, completeness, and consistency.
- Assigning Data Ownership: Data governance assigns responsibilities for data quality to specific individuals or teams within the organization. This ensures accountability and ownership of data quality issues.
- Implementing Data Quality Processes: Data governance defines the processes and workflows for data quality management, including data validation, cleansing, and monitoring. These processes are essential for maintaining data integrity and consistency.
- Ensuring Regulatory Compliance: Data governance ensures that the organization complies with data-related regulations and standards, including data privacy laws. This is crucial for protecting customer privacy and avoiding legal and reputational risks.
The Impact of Data Quality Management on Customer Experience
Data quality management has a direct impact on the overall customer experience. Customers expect organizations to have accurate and up-to-date information about them, and any data quality issues can negatively affect their experience. Here are some ways data quality management impacts customer experience:
- Personalization: Accurate customer data enables organizations to personalize their interactions and offers based on individual preferences and needs. This enhances the customer experience and increases customer satisfaction.
- Efficient Service: High-quality data ensures that customer requests and inquiries are handled efficiently. For example, if a customer calls customer service, having accurate and complete customer information readily available can help resolve issues quickly and effectively.
- Consistent Communication: Consistent and accurate customer data allows organizations to maintain a cohesive and personalized communication strategy across different channels. This helps build trust and strengthens the customer relationship.
- Proactive Issue Resolution: Data quality management enables organizations to identify and address issues proactively. By analyzing customer data, organizations can identify patterns or trends that may indicate potential issues and take preventive measures.
Conclusion
Data quality management is not only crucial for the efficient functioning of organizations but also has significant ethical implications. Poor data quality can lead to misleading decision-making, unfair treatment of customers, privacy violations, and unintended discrimination. Implementing best practices, such as data governance, data profiling, data cleansing, data integration, and data quality metrics, can help organizations ensure data quality throughout its lifecycle. By prioritizing data quality, organizations can enhance customer experience, make informed decisions, and comply with regulatory requirements.
Contact us
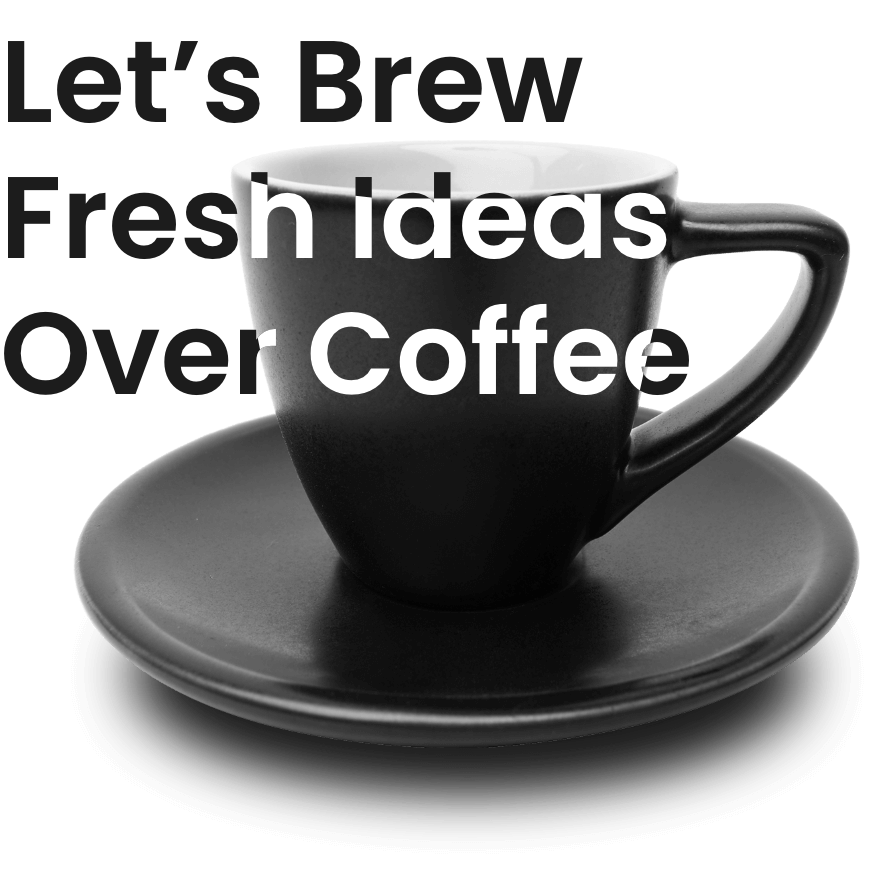
Spanning 8 cities worldwide and with partners in 100 more, we’re your local yet global agency.
Fancy a coffee, virtual or physical? It’s on us – let’s connect!