The impact of customer master data management on customer sentiment analysis
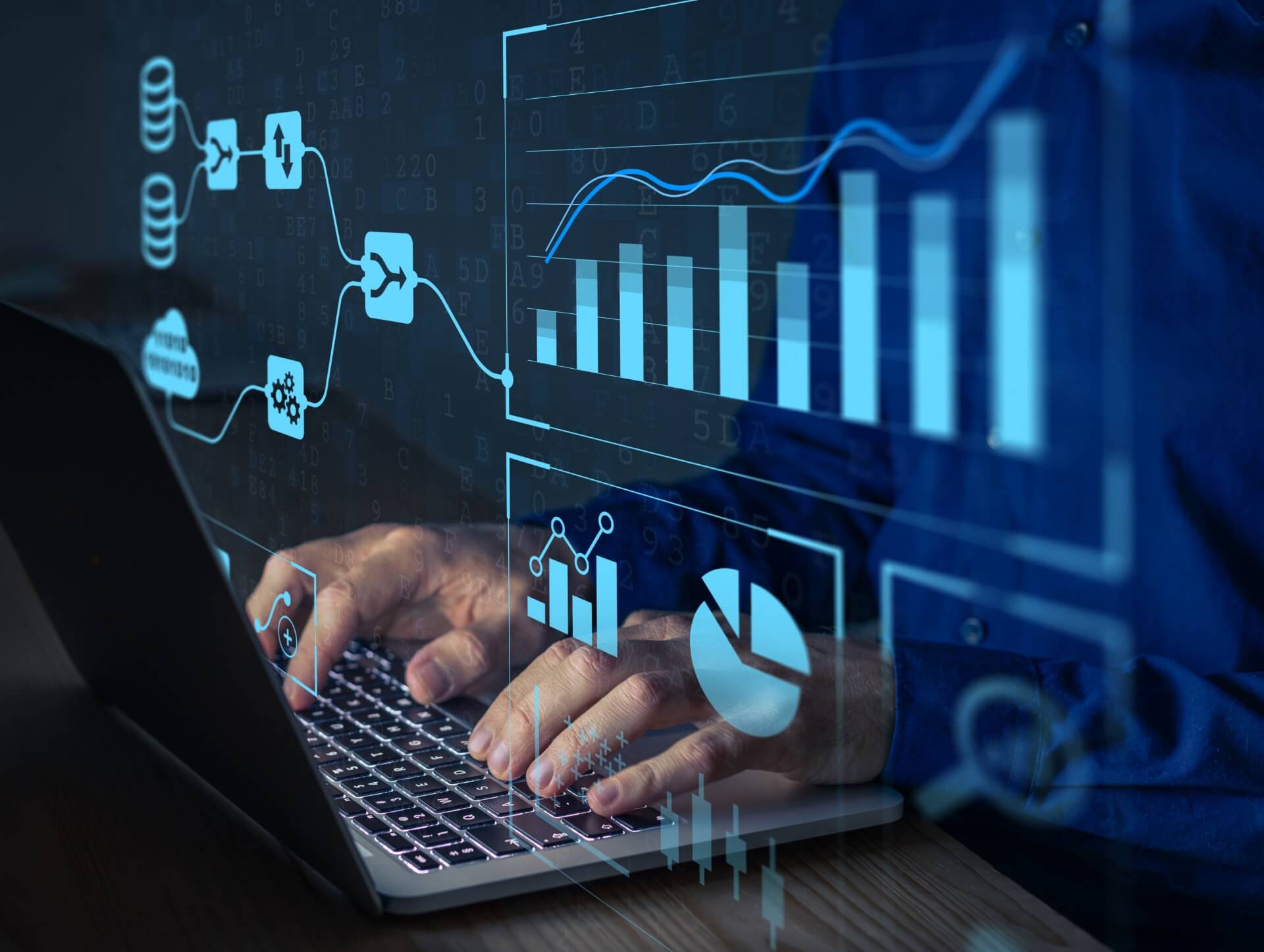
06/09/2023
Customer sentiment analysis has become increasingly important for businesses in today's data-driven world. It involves analyzing customer feedback, opinions, and emotions to gain valuable insights into customer satisfaction, brand perception, and overall customer experience. However, the accuracy and effectiveness of sentiment analysis heavily rely on the quality and completeness of customer data. This is where customer master data management (MDM) plays a crucial role.
What is Customer Master Data Management?
Customer master data management is the process of collecting, organizing, and maintaining customer data to ensure its accuracy, consistency, and reliability across an organization. It involves creating a single, trusted view of customer data that can be shared across different systems and departments. A comprehensive customer MDM strategy enables businesses to have a holistic understanding of their customers, improve data quality, enhance customer segmentation, and enable effective customer sentiment analysis.
The Benefits of Customer Master Data Management
Implementing a robust customer MDM solution offers several benefits to businesses:
- Improved Data Quality: Customer MDM ensures that the customer data is accurate, complete, and up-to-date. This helps in eliminating duplicate records, resolving inconsistencies, and maintaining data integrity.
- Enhanced Customer Segmentation: By consolidating and standardizing customer data, businesses can segment their customer base more effectively. This enables targeted marketing campaigns, personalized customer experiences, and improved customer satisfaction.
- Efficient Customer Sentiment Analysis: Customer MDM provides a unified view of customer data, making it easier to analyze customer sentiment across different touchpoints. This analysis helps in identifying trends, patterns, and areas for improvement, ultimately leading to better decision-making and enhanced customer experience.
- Streamlined Business Processes: With accurate and consistent customer data, businesses can streamline their sales, marketing, and customer service processes. This leads to improved operational efficiency, reduced costs, and increased customer loyalty.
The Role of Customer Master Data Management in Customer Sentiment Analysis
Customer sentiment analysis relies on the availability of high-quality customer data. Customer MDM ensures that the data used for sentiment analysis is accurate, complete, and relevant. Let's explore how customer MDM impacts different aspects of sentiment analysis:
Data Governance in Customer MDM
Data governance is a critical component of customer MDM. It involves defining policies, procedures, and standards for data management, ensuring data quality, and establishing data ownership. Effective data governance in customer MDM ensures that the customer data used for sentiment analysis is trustworthy and reliable.
MDM Best Practices for Data Quality Management
Implementing MDM best practices for data quality management is essential for accurate sentiment analysis. These best practices include data profiling, data cleansing, data standardization, and data enrichment. By following these practices, businesses can improve the quality of customer data, leading to more reliable sentiment analysis results.
MDM Strategy for Effective Sentiment Analysis
A well-defined MDM strategy is crucial for effective sentiment analysis. This strategy should include identifying the relevant customer data sources, integrating data from disparate systems, and establishing data governance processes. By aligning MDM with sentiment analysis goals, businesses can maximize the value of customer data and extract meaningful insights.
The MDM Implementation Process
Implementing a customer MDM solution involves several stages, including data discovery, data modeling, data integration, data validation, and data migration. Each stage plays a crucial role in ensuring the accuracy and completeness of customer data, which directly impacts the quality of sentiment analysis.
Customer Master Data Governance
Customer master data governance refers to the processes, policies, and controls put in place to manage customer data throughout its lifecycle. It involves defining data ownership, roles, and responsibilities, establishing data stewardship practices, and enforcing data quality standards. Effective customer master data governance ensures the availability of reliable and accurate customer data for sentiment analysis purposes.
MDM Tools and Technologies
Several MDM tools and technologies are available in the market to support customer MDM initiatives. These tools provide functionalities for data cleansing, data integration, data matching, and data quality management. Businesses can leverage these tools to streamline their customer MDM processes and improve the quality of customer data for sentiment analysis.
Data Integration in Customer MDM
Data integration plays a crucial role in customer MDM. It involves consolidating and integrating customer data from various sources, such as CRM systems, marketing databases, and customer support platforms. By integrating data from disparate systems, businesses can create a unified view of customer data, which is essential for accurate sentiment analysis.
MDM for Enterprise Scalability
Customer MDM is not limited to small or medium-sized businesses. It is equally important for enterprise-scale organizations. With a large customer base and complex data landscape, enterprise-level MDM solutions help in managing customer data at scale, ensuring data consistency, and enabling accurate sentiment analysis across the organization.
MDM and Data Security
Data security is a critical aspect of customer MDM. It involves implementing security measures to protect customer data from unauthorized access, ensuring data privacy, and complying with data protection regulations. By prioritizing data security in customer MDM, businesses can build trust with their customers and mitigate the risk of data breaches that can impact sentiment analysis.
MDM and Data Analytics
Customer MDM and data analytics go hand in hand. By leveraging customer data collected through MDM processes, businesses can gain valuable insights into customer behavior, preferences, and sentiments. These insights can be used to optimize marketing strategies, improve product offerings, and enhance the overall customer experience.
Challenges in Customer MDM
Implementing an effective customer MDM strategy comes with its own set of challenges. Some common challenges include:
- Data Quality: Ensuring data quality is a constant challenge in customer MDM. Duplicate, incomplete, and inconsistent customer data can impact the accuracy of sentiment analysis results.
- Data Integration: Integrating customer data from various sources can be complex and time-consuming. Data integration challenges can hinder the creation of a unified view of customer data.
- Data Governance: Establishing and maintaining effective data governance processes require organizational alignment, clear roles, and responsibilities, and ongoing data stewardship efforts.
- MDM ROI and Value: Demonstrating the return on investment (ROI) and value of customer MDM initiatives can be challenging. Businesses need to align MDM goals with tangible business outcomes and measure the impact of MDM on sentiment analysis and overall business performance.
Customer Sentiment Analysis Case Studies
Several organizations have successfully implemented customer sentiment analysis using customer MDM. These case studies highlight the benefits and impact of customer MDM on sentiment analysis:
- Case Study 1: Retail Industry - A retail company implemented customer MDM to consolidate customer data from multiple sales channels. By analyzing customer sentiment across different touchpoints, the company identified areas for improvement in customer service, resulting in increased customer satisfaction and loyalty.
- Case Study 2: Healthcare Industry - A healthcare organization implemented customer MDM to centralize patient data from various systems. By analyzing patient sentiment and feedback, the organization improved patient care processes, leading to better patient outcomes and higher patient satisfaction scores.
- Case Study 3: Hospitality Industry - A hotel chain implemented customer MDM to create a single view of customer data across its properties. By analyzing customer sentiment and preferences, the chain personalized its marketing campaigns, resulting in increased bookings and revenue.
Customer MDM in Multi-Cloud Environments
With the increasing adoption of cloud computing, businesses are leveraging multi-cloud environments for their IT infrastructure. Customer MDM solutions are evolving to support multi-cloud environments, enabling businesses to manage customer data across different cloud platforms securely. This ensures data consistency and accessibility for sentiment analysis purposes, regardless of the underlying cloud infrastructure.
ROI and Value of Effective Customer MDM
Implementing an effective customer MDM strategy can deliver significant ROI and value to businesses:
- Improved Customer Experience: By analyzing customer sentiment and feedback, businesses can identify areas for improvement and deliver personalized experiences, resulting in increased customer satisfaction and loyalty.
- Increased Operational Efficiency: With accurate and consistent customer data, businesses can streamline their processes, reduce manual effort, and improve operational efficiency, ultimately leading to cost savings.
- Better Decision-Making: Customer MDM provides businesses with reliable and accurate customer data, enabling informed decision-making based on data-driven insights.
- Competitive Advantage: Businesses that effectively manage their customer data and leverage sentiment analysis for strategic decision-making gain a competitive edge in the market.
Data Lifecycle Management and Customer MDM
Data lifecycle management encompasses the end-to-end management of data throughout its lifecycle, from creation to deletion. Customer MDM plays a vital role in data lifecycle management by ensuring the accuracy, consistency, and security of customer data at each stage:
Data Security throughout the Lifecycle
Customer MDM ensures that customer data is protected at each stage of the data lifecycle. This includes implementing appropriate security measures, access controls, and data encryption to prevent unauthorized access and data breaches.
Data Compliance in Customer Data Management
Customer MDM helps businesses comply with data protection regulations and privacy laws. By establishing data governance processes, businesses can ensure that customer data is managed in accordance with applicable regulations, such as GDPR or CCPA.
Master Data in Lifecycle Management
Master data, including customer data, is a critical component of data lifecycle management. By managing customer data effectively through MDM, businesses can ensure its accuracy, relevance, and availability throughout its lifecycle.
Metadata Management in Data Lifecycle
Metadata management is crucial for effective data lifecycle management. By managing metadata associated with customer data, businesses can ensure proper data classification, data lineage, and data integration, enabling accurate sentiment analysis.
Automation in Data Lifecycle Management
Automation plays a significant role in data lifecycle management. By leveraging automation tools and technologies, businesses can streamline data processes, reduce manual effort, and ensure the timely availability of customer data for sentiment analysis.
Cloud-Based Data Lifecycle Management
Cloud-based solutions are increasingly being adopted for data lifecycle management. Cloud-based customer MDM platforms offer scalability, flexibility, and cost-efficiency, enabling businesses to manage customer data effectively and perform sentiment analysis in a cloud environment.
Analytics in Data Lifecycle Management
Analytics plays a crucial role in data lifecycle management. By leveraging analytics tools and techniques, businesses can gain insights from customer data throughout its lifecycle, enabling proactive decision-making and continuous improvement.
Challenges in Data Lifecycle Management
Data lifecycle management comes with its own set of challenges:
- Data Volume and Velocity: Managing large volumes of customer data and handling its high velocity pose challenges in data lifecycle management.
- Data Integration and Interoperability: Integrating customer data from various sources and ensuring interoperability between systems can be complex and time-consuming.
- Data Privacy and Security: Protecting customer data throughout its lifecycle and ensuring compliance with data privacy regulations is a constant challenge.
- Legacy Systems and Data Migration: Migrating customer data from legacy systems to modern MDM platforms can be challenging, requiring careful planning and execution.
Best Practices for Data Lifecycle Management
Implementing best practices for data lifecycle management can help businesses overcome challenges and maximize the value of customer data:
- Data Governance: Establish clear data governance processes, roles, and responsibilities to ensure data quality and compliance throughout the data lifecycle.
- Data Integration and Interoperability: Implement robust data integration and interoperability frameworks to enable seamless data flow across systems and departments.
- Data Security and Privacy: Prioritize data security and privacy by implementing encryption, access controls, and data anonymization techniques.
- Data Quality Management: Implement data quality management processes to ensure the accuracy, completeness, and reliability of customer data throughout its lifecycle.
- Continuous Improvement: Continuously monitor and evaluate data lifecycle processes, identify areas for improvement, and adapt to evolving business needs and regulatory requirements.
ROI of Effective Data Lifecycle Management
Implementing effective data lifecycle management practices can deliver significant ROI to businesses:
- Improved Data Quality: By managing customer data effectively throughout its lifecycle, businesses can improve data quality, leading to more reliable sentiment analysis results and better decision-making.
- Enhanced Data Security: Prioritizing data security throughout the data lifecycle mitigates the risk of data breaches and protects customer data, fostering trust and credibility with customers.
- Operational Efficiency: Streamlining data processes, automating data management tasks, and ensuring data accessibility improves operational efficiency and reduces costs.
- Compliance and Risk Management: Implementing data lifecycle management practices ensures compliance with data protection regulations and minimizes the risk of non-compliance penalties.
Conclusion
Customer master data management plays a significant role in enabling accurate and effective customer sentiment analysis. By implementing robust customer MDM strategies, businesses can ensure the accuracy, completeness, and reliability of customer data, leading to improved data quality, enhanced customer segmentation, and efficient sentiment analysis. With the right MDM tools, technologies, and best practices, businesses can leverage customer data to gain valuable insights, improve customer experience, and gain a competitive edge in the market.
Contact us
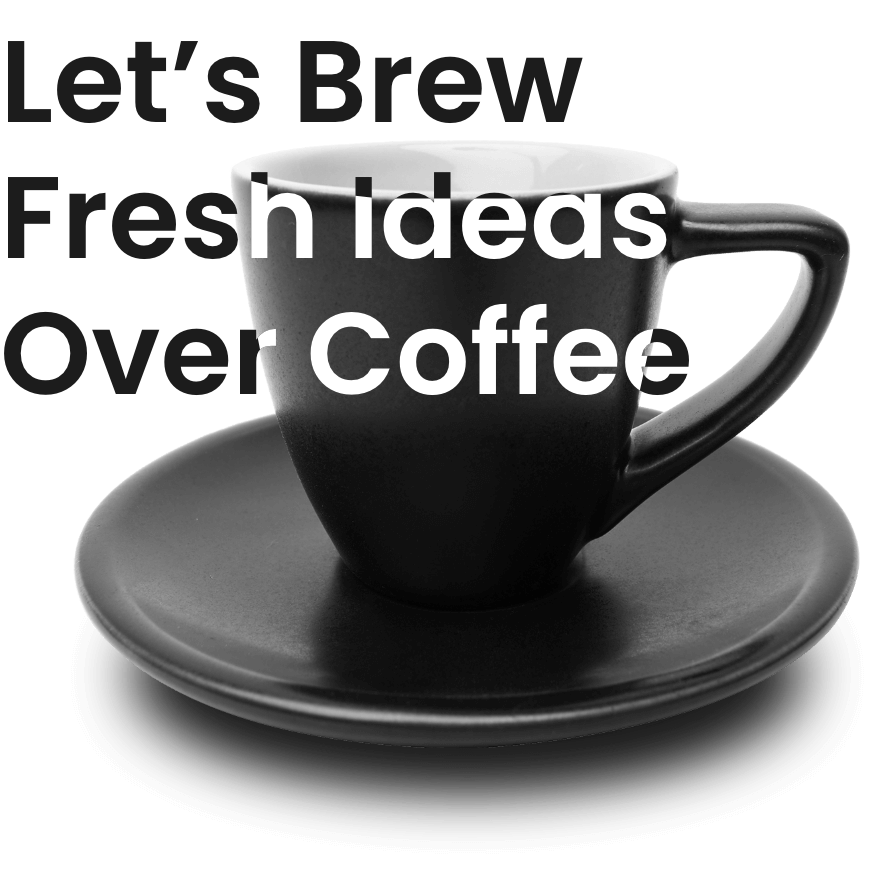
Spanning 8 cities worldwide and with partners in 100 more, we’re your local yet global agency.
Fancy a coffee, virtual or physical? It’s on us – let’s connect!