The impact of data governance on data security in MDM
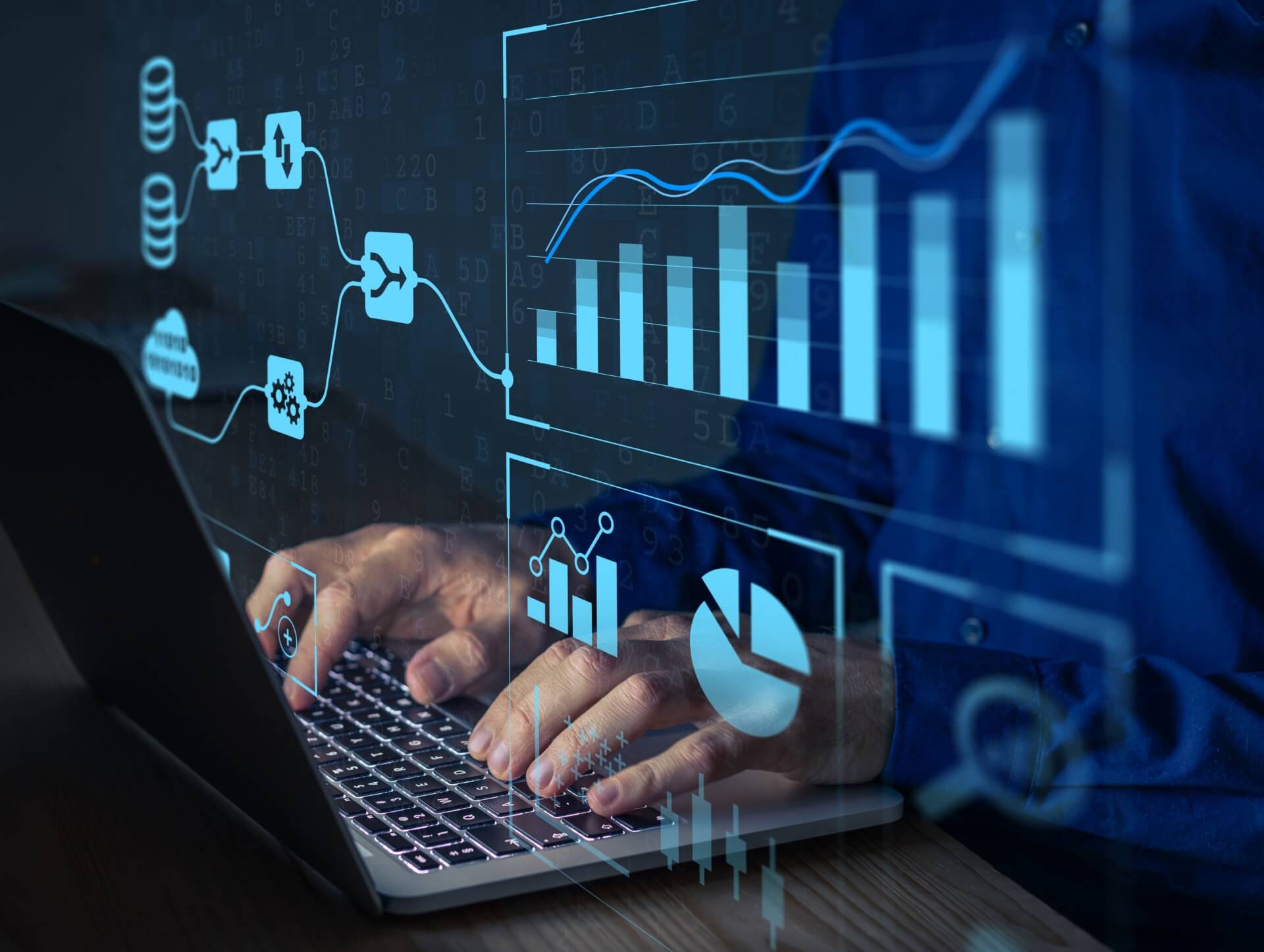
06/09/2023
Master Data Management (MDM) is a critical discipline for businesses looking to maintain accurate and consistent master data across their organization. It involves the implementation of processes, policies, and technologies to ensure the integrity and quality of master data, such as customer information, product data, and financial records.
As businesses increasingly rely on data to make informed decisions, data security has become a top concern. Data breaches and unauthorized access to sensitive information can have severe consequences, including financial losses, reputational damage, and legal implications. Therefore, it is essential to understand the impact of data governance on data security in the context of MDM.
Data Governance in MDM
Data governance refers to the overall management of data within an organization, including the creation and enforcement of policies, procedures, and standards. In the context of MDM, data governance plays a crucial role in ensuring the accuracy, consistency, and security of master data. It involves defining roles and responsibilities, establishing data quality metrics, and implementing processes to monitor and control data throughout its lifecycle.
Effective data governance in MDM requires a comprehensive understanding of the organization's data landscape, including data sources, data flows, and data dependencies. By implementing a robust data governance framework, businesses can minimize the risk of data breaches, ensure compliance with regulatory requirements, and optimize the value of their master data.
Data Security throughout the Lifecycle
Data security is a critical aspect of data governance in MDM. It involves protecting data from unauthorized access, ensuring data confidentiality, integrity, and availability. Data security measures should be implemented throughout the data lifecycle, from data creation to data disposal.
One of the key considerations in data security is access control. Businesses need to implement appropriate access controls to ensure that only authorized individuals can access and modify master data. This can be achieved through user authentication, role-based access control, and encryption of sensitive data.
Data encryption is another crucial aspect of data security in MDM. By encrypting sensitive data, businesses can protect it from unauthorized access even if the data is compromised. Encryption algorithms and key management practices should be implemented to ensure the confidentiality and integrity of encrypted data.
Data governance in MDM also involves implementing data masking techniques to protect sensitive data during non-production environments or when sharing data with external parties. Data masking involves replacing sensitive data with realistic but fictitious data, ensuring that the original data cannot be easily identified or reconstructed.
Data Compliance in Management
Data compliance refers to the adherence to regulatory requirements and industry standards related to data management. In the context of MDM, data compliance is essential to ensure the privacy and security of master data. Organizations need to comply with various regulations, such as the General Data Protection Regulation (GDPR) and the Health Insurance Portability and Accountability Act (HIPAA), depending on the nature of their business and the data they handle.
Data governance plays a crucial role in ensuring data compliance in MDM. It involves defining and enforcing data policies and procedures to ensure that master data is handled in accordance with regulatory requirements. Data governance frameworks should include mechanisms for monitoring and reporting data compliance, as well as processes for addressing non-compliance issues.
By integrating data compliance into their data governance practices, businesses can mitigate the risk of non-compliance penalties, reputational damage, and legal implications. It also demonstrates a commitment to data privacy and security, which can enhance customer trust and loyalty.
Master Data in Lifecycle Management
Master data is a critical component of an organization's data lifecycle. It refers to the core data entities that are shared across multiple systems and business processes. Managing master data throughout its lifecycle involves various activities, such as data creation, data maintenance, data integration, and data archival.
Data governance in MDM ensures the effective management of master data throughout its lifecycle. It involves defining data ownership, establishing data quality metrics, and implementing processes for data creation, validation, and maintenance. By implementing a robust master data governance framework, businesses can ensure the accuracy, consistency, and usability of master data throughout its lifecycle.
Metadata management is also an essential aspect of master data lifecycle management. Metadata provides information about the structure, context, and meaning of data. By managing metadata effectively, businesses can enhance data discoverability, traceability, and understanding. Metadata management should be integrated into the data governance framework to ensure the effective management of master data throughout its lifecycle.
Automation plays a vital role in master data lifecycle management. By implementing automated workflows and processes, businesses can streamline data creation, validation, and maintenance activities. Automation also helps to reduce the risk of human error and ensure consistency and accuracy in master data management.
Data Analytics in Lifecycle
Data analytics is a valuable tool for businesses looking to derive insights and make informed decisions. In the context of master data lifecycle management, data analytics can provide valuable insights into data quality, data usage patterns, and data dependencies.
By analyzing master data throughout its lifecycle, businesses can identify data quality issues, such as duplicate records, incomplete data, or inconsistent data. Data analytics can also help organizations understand how master data is used across different systems and processes, enabling them to optimize data integration and data flow.
Data analytics can also help businesses identify trends and patterns in master data, enabling them to make data-driven decisions and improve business performance. For example, analyzing customer master data can provide insights into customer behavior, preferences, and buying patterns, which can be used to personalize marketing campaigns and enhance the customer experience.
Challenges in Data Lifecycle Management
Data lifecycle management in MDM is not without its challenges. Organizations face various challenges in managing master data throughout its lifecycle, including:
- Data quality: Ensuring the accuracy, consistency, and completeness of master data can be challenging, especially when dealing with large volumes of data from multiple sources.
- Data integration: Integrating master data from different sources and systems can be complex, requiring careful mapping and transformation of data.
- Data governance: Establishing a robust data governance framework and ensuring compliance with data policies and procedures can be challenging, especially in large organizations with multiple stakeholders.
- Data security: Protecting master data from unauthorized access and ensuring data confidentiality, integrity, and availability can be challenging, especially in the face of evolving cybersecurity threats.
- Data scalability: Managing master data at scale can be challenging, especially when dealing with growing volumes of data and increasing data complexity.
Addressing these challenges requires a combination of technology, processes, and people. Organizations need to invest in robust MDM tools and technologies, establish clear data governance policies and procedures, and provide ongoing training and support to employees involved in master data management.
Best Practices for Lifecycle Management
To ensure the effective management of master data throughout its lifecycle, organizations should consider the following best practices:
- Define clear data governance policies: Establish clear policies and procedures for master data management, including data creation, data validation, data integration, and data archival.
- Implement data quality management: Define data quality metrics and implement processes to monitor and improve data quality throughout the data lifecycle.
- Establish data ownership: Clearly define data ownership and accountability to ensure that master data is managed by the appropriate stakeholders.
- Invest in MDM tools and technologies: Leverage MDM solutions and technologies to automate master data management processes, improve data accuracy, and enhance data governance.
- Provide ongoing training and support: Ensure that employees involved in master data management receive proper training and support to effectively carry out their roles and responsibilities.
- Regularly review and update data policies: Regularly review and update data policies and procedures to ensure alignment with changing business requirements and regulatory standards.
ROI of Effective Data Lifecycle
Implementing effective data lifecycle management practices can deliver several benefits to organizations, including:
- Improved data quality: Effective data lifecycle management can help improve the accuracy, consistency, and completeness of master data, enabling organizations to make better-informed decisions.
- Enhanced data governance: By implementing robust data governance practices, organizations can ensure compliance with regulatory requirements, mitigate the risk of data breaches, and optimize the value of their master data.
- Increased operational efficiency: Streamlining data creation, validation, integration, and archival processes can help organizations improve operational efficiency and reduce costs.
- Better decision-making: By analyzing master data throughout its lifecycle, organizations can derive valuable insights that can drive better decision-making and improve business performance.
- Enhanced customer experience: By managing customer master data effectively, organizations can personalize customer interactions, improve customer service, and enhance the overall customer experience.
While the return on investment (ROI) of effective data lifecycle management may vary depending on the organization's size, industry, and specific business requirements, the benefits outlined above can contribute to improved business performance, increased competitiveness, and enhanced customer satisfaction.
Conclusion
Data governance plays a critical role in ensuring the security of master data in MDM. By implementing robust data governance practices, organizations can minimize the risk of data breaches, ensure compliance with regulatory requirements, and optimize the value of their master data. Data security measures should be implemented throughout the data lifecycle, and organizations should also consider data compliance, master data in lifecycle management, data analytics, and the challenges and best practices for effective data lifecycle management. By implementing these practices, organizations can achieve a positive ROI and derive maximum value from their master data.
Contact us
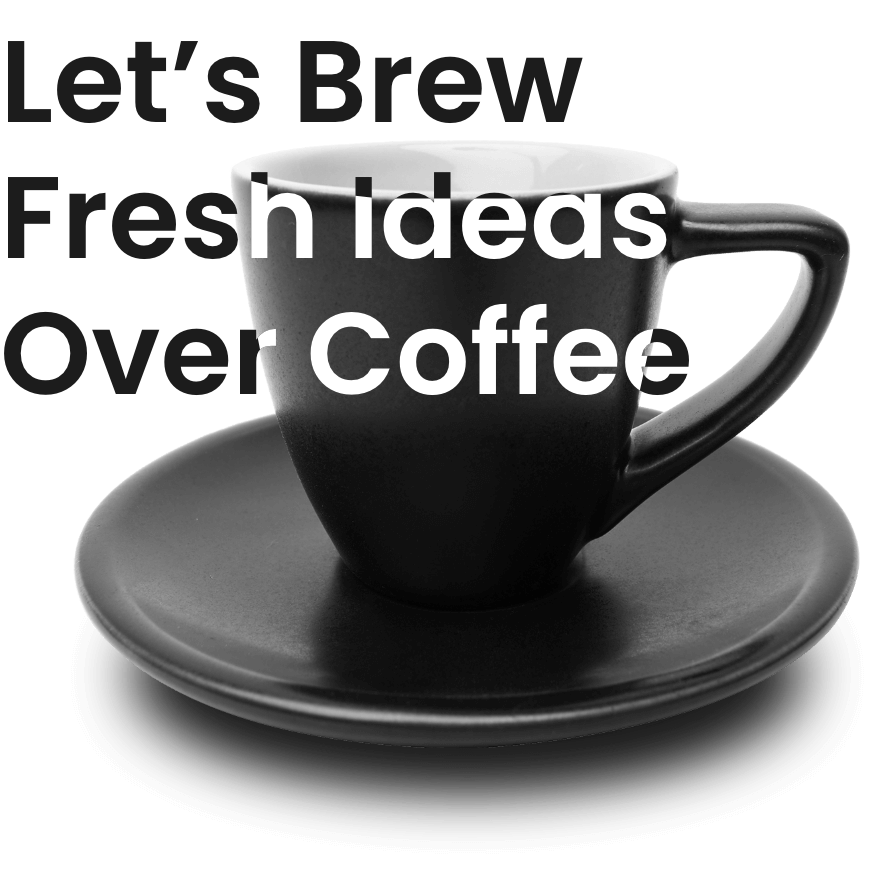
Spanning 8 cities worldwide and with partners in 100 more, we’re your local yet global agency.
Fancy a coffee, virtual or physical? It’s on us – let’s connect!