The impact of data governance tools on master data management strategy
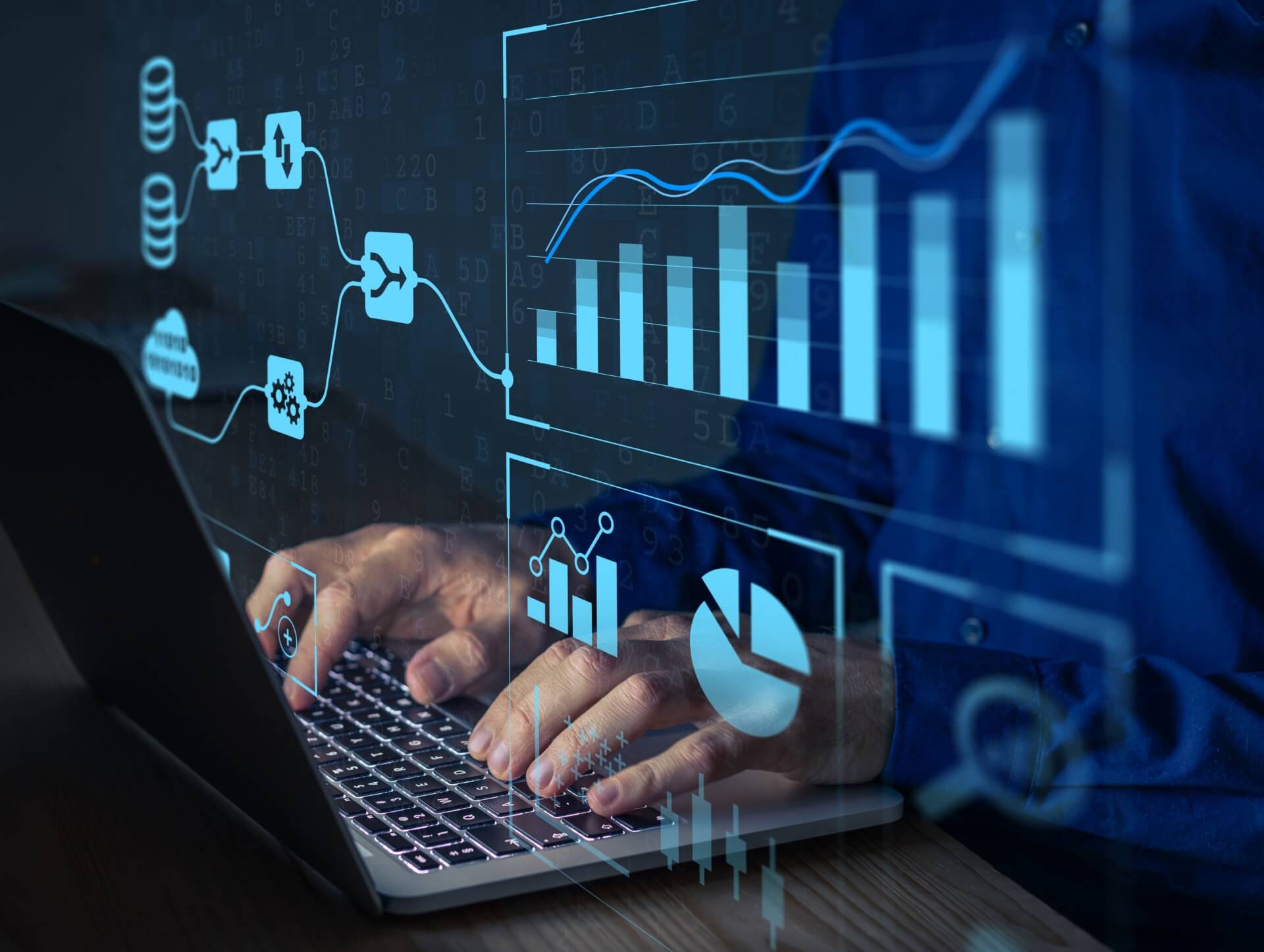
06/09/2023
In today's data-driven world, businesses are increasingly recognizing the importance of effectively managing their data assets. Master Data Management (MDM) refers to the processes, tools, and technologies used to create and maintain consistent and accurate master data throughout an organization. It involves managing critical data entities, such as customers, products, and suppliers, to ensure data quality, consistency, and reliability across various systems and applications. Data governance, on the other hand, focuses on establishing and maintaining policies and procedures for managing data assets.
The Need for Data Governance in MDM
Data governance plays a crucial role in the success of any MDM strategy. Without proper data governance, organizations may struggle with data inconsistencies, duplication, and poor data quality. By implementing data governance practices and tools, businesses can ensure that their master data is accurate, complete, and up-to-date, leading to improved decision-making, operational efficiency, and customer satisfaction.
Benefits of Data Governance in MDM
Implementing data governance tools in the MDM strategy brings several benefits to businesses. Firstly, it helps in establishing clear ownership and accountability for data. With defined roles and responsibilities, organizations can ensure that data is managed by the right people with the necessary expertise. This leads to improved data quality and reliability.
Secondly, data governance enables organizations to establish data standards and guidelines. By defining data definitions, naming conventions, and data classification, businesses can ensure consistency and uniformity across systems and applications. This, in turn, improves data integration and data sharing capabilities.
Thirdly, data governance facilitates compliance with regulatory requirements and data privacy laws. With data governance tools, organizations can enforce data protection policies, monitor data access and usage, and track data lineage. This helps in maintaining data security and ensuring compliance with industry regulations, such as GDPR and CCPA.
Lastly, data governance tools provide organizations with the ability to monitor and measure data quality. By implementing data quality management processes and tools, businesses can identify and resolve data issues, such as duplicates, inconsistencies, and inaccuracies. This leads to improved data accuracy and reliability, enabling better decision-making and operational efficiency.
Best Practices for Data Governance in MDM
Implementing data governance in MDM requires a well-defined strategy and adherence to best practices. Here are some key best practices to consider:
- Establish Data Governance Framework: Define a data governance framework that outlines the organizational structure, roles, responsibilities, and processes for managing data assets. This framework should align with the business objectives and support the MDM strategy.
- Define Data Ownership and Stewardship: Clearly define data ownership and stewardship roles and responsibilities. Assign data stewards who will be responsible for managing and maintaining the quality of master data.
- Develop Data Governance Policies and Procedures: Create data governance policies and procedures that define data standards, guidelines, and processes for data management. These policies should be communicated across the organization and regularly reviewed and updated.
- Implement Data Quality Management: Establish data quality management processes and tools to monitor, measure, and improve data quality. This includes data profiling, data cleansing, and data validation techniques.
- Ensure Data Privacy and Security: Implement data privacy and security measures to protect sensitive data. This includes data encryption, access controls, and regular data backups.
- Provide Data Governance Training and Awareness: Train employees on data governance principles, policies, and processes. Create awareness about the importance of data governance and its impact on business outcomes.
- Monitor and Measure Data Governance: Establish metrics and Key Performance Indicators (KPIs) to measure the effectiveness of data governance practices. Regularly monitor and evaluate data quality, compliance, and data governance performance.
Challenges in Data Governance and MDM
Implementing data governance in MDM is not without its challenges. Some common challenges include:
- Data Silos: Data silos can hinder effective data governance and MDM. When different departments and systems maintain their own data repositories, it becomes difficult to ensure data consistency and accuracy.
- Data Quality: Poor data quality can be a major challenge in MDM. Inaccurate, incomplete, and inconsistent data can lead to unreliable insights and decision-making.
- Data Governance Adoption: Getting buy-in from stakeholders and ensuring widespread adoption of data governance practices can be a challenge. Resistance to change and lack of awareness about the benefits of data governance can hinder successful implementation.
- Data Privacy and Security: Ensuring data privacy and security throughout the MDM lifecycle can be challenging. With the increasing complexity of data privacy regulations, organizations need to implement robust security measures and ensure compliance.
- Data Governance Maturity: Building a mature data governance program takes time and effort. It requires continuous monitoring, improvement, and alignment with evolving business needs and regulatory requirements.
Data Governance Tools and Technologies
Several tools and technologies are available in the market to support data governance and MDM initiatives. These tools provide functionalities such as data profiling, data cleansing, data lineage tracking, and metadata management. Some popular data governance tools include:
- Informatica MDM
- IBM InfoSphere MDM
- Talend Master Data Management
- Oracle Master Data Management
- SAP Master Data Governance
- Collibra Data Governance
- Stibo Systems STEP
Case Studies: Successful Implementation of Data Governance in MDM
Let's take a look at some real-world case studies where organizations have successfully implemented data governance in their MDM strategy:
Case Study 1: Company A
Company A, a global manufacturing company, implemented a data governance program to improve the quality of its customer master data. By implementing data governance tools and processes, they were able to eliminate duplicate customer records, standardize customer data, and ensure data consistency across their systems. This led to improved customer satisfaction, streamlined order processing, and reduced operational costs.
Case Study 2: Company B
Company B, a leading retail chain, implemented a data governance framework to address data quality issues in its product master data. By defining data ownership and stewardship roles, implementing data quality management processes, and leveraging data governance tools, they were able to improve data accuracy, reduce product data errors, and streamline their supply chain operations. This resulted in improved inventory management, reduced stockouts, and increased sales.
Case Study 3: Company C
Company C, a financial services company, implemented data governance and MDM to address data privacy and compliance challenges. By implementing data governance tools and processes, they were able to ensure compliance with industry regulations, such as GDPR and CCPA. They improved data security, implemented data masking techniques, and established data access controls. This led to enhanced customer trust, reduced risks of data breaches, and avoided regulatory penalties.
The Future of Data Governance in MDM
The future of data governance in MDM looks promising. With advancements in technology, such as artificial intelligence and machine learning, data governance tools and practices are becoming more sophisticated. Organizations are increasingly adopting cloud-based master data management solutions, which provide scalability, flexibility, and cost-efficiency. The integration of data governance with advanced analytics and data visualization tools allows businesses to derive valuable insights from their master data, enabling data-driven decision-making and improving customer experience.
Conclusion
Data governance plays a vital role in the success of master data management strategies. By implementing data governance tools and best practices, businesses can ensure data quality, consistency, and compliance throughout the MDM lifecycle. With the right data governance framework and technologies in place, organizations can unlock the full potential of their master data, leading to improved operational efficiency, better decision-making, and enhanced customer experience. As data continues to grow exponentially, the importance of data governance in MDM will only continue to increase, making it a crucial aspect of any organization's data management strategy.
Contact us
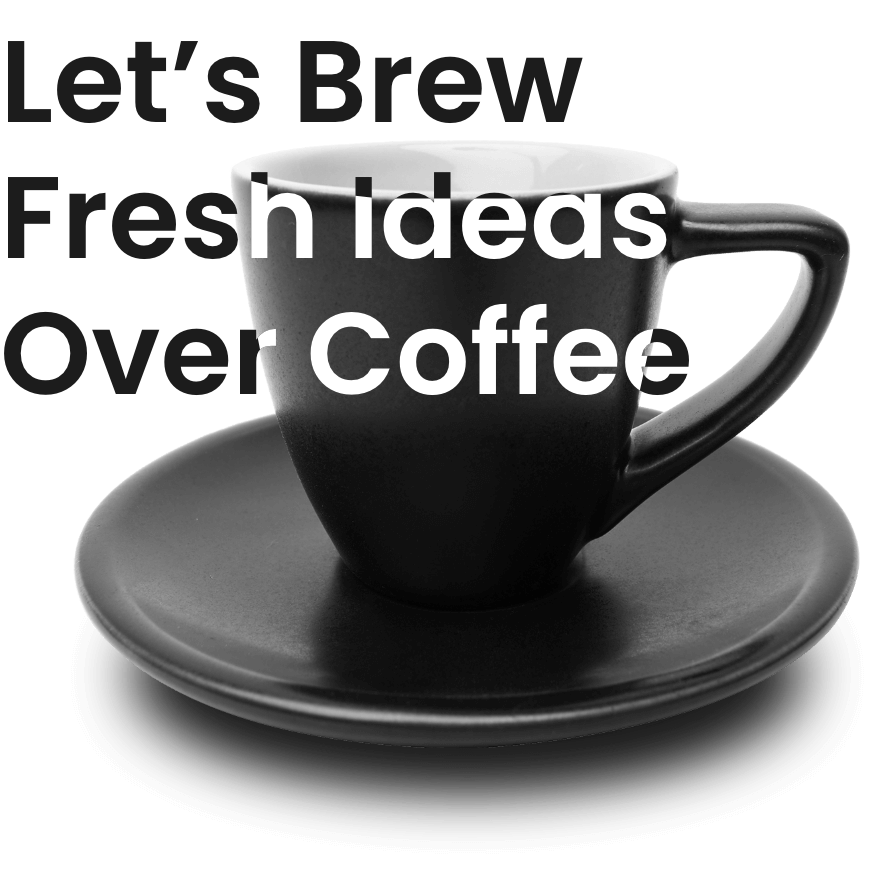
Spanning 8 cities worldwide and with partners in 100 more, we’re your local yet global agency.
Fancy a coffee, virtual or physical? It’s on us – let’s connect!