The impact of data quality on artificial intelligence and machine learning algorithms
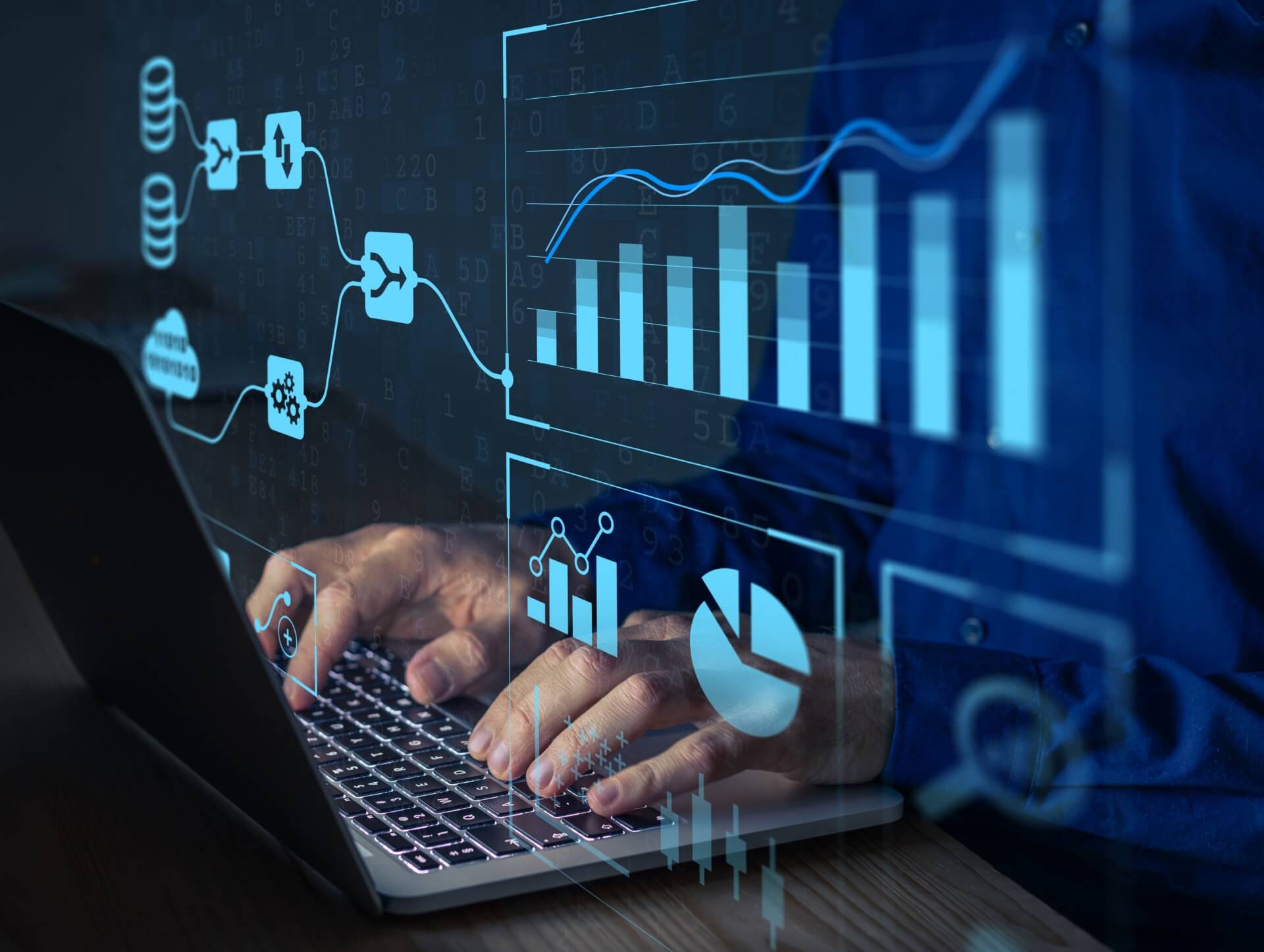
06/09/2023
In today's digital age, data is the driving force behind many technological advancements. With the rise of artificial intelligence (AI) and machine learning (ML), organizations are leveraging vast amounts of data to gain valuable insights and make data-driven decisions. However, the success of AI and ML algorithms heavily relies on the quality of the data used for training and analysis. In this article, we will explore the impact of data quality on AI and ML algorithms and discuss the importance of implementing robust data quality management practices.
The Role of Data Quality in AI and ML
Data quality refers to the accuracy, completeness, consistency, and relevancy of data. In the context of AI and ML, high-quality data is essential for training algorithms to make accurate predictions and generate meaningful insights. Poor data quality can lead to biased or inaccurate results, rendering AI and ML models ineffective or even harmful. Let's delve into some key aspects of data quality and its impact on AI and ML algorithms.
Accuracy and Consistency
Accurate and consistent data is crucial for building reliable AI and ML models. Inaccurate or inconsistent data can introduce errors and biases into the training process, leading to unreliable predictions. For example, if an AI model is trained on faulty customer data, it may make incorrect recommendations or predictions, resulting in a poor user experience and potential financial losses for businesses.
Implementing master data management (MDM) solutions can help ensure the accuracy and consistency of data used in AI and ML algorithms. MDM involves creating a single, trusted source of master data that is shared across different systems and applications. By implementing a robust MDM strategy, organizations can eliminate duplicate or inconsistent data, thereby improving the accuracy and consistency of data used for training AI and ML algorithms.
Data Completeness and Relevancy
Complete and relevant data is essential for training AI and ML algorithms to make accurate predictions. Incomplete data can lead to biased or incomplete insights, while irrelevant data can introduce noise and confusion into the training process. It is crucial for organizations to ensure that the data used for training AI and ML models is complete and relevant to the problem at hand.
MDM plays a significant role in ensuring data completeness and relevancy. By implementing customer master data management, organizations can consolidate and cleanse customer data from various sources, ensuring that the data used for training AI and ML models is complete and relevant. Additionally, MDM platforms provide data governance capabilities that allow organizations to define and enforce data quality standards, ensuring that only high-quality data is used for AI and ML training.
Data Governance and Data Integration
Data governance is the process of managing the availability, usability, integrity, and security of data within an organization. In the context of AI and ML, data governance plays a crucial role in ensuring data quality throughout the data lifecycle. By implementing data governance practices, organizations can establish policies and procedures for data quality management, data integration, and data security.
When it comes to MDM, data governance is essential for maintaining data quality and integrity. MDM solutions provide a centralized platform for managing master data, ensuring that data is consistent, accurate, and up-to-date across different systems and applications. By implementing data governance in MDM, organizations can enforce data quality standards, track data lineage, and ensure compliance with data regulations.
Challenges in Data Quality for AI and ML
While data quality is crucial for the success of AI and ML algorithms, organizations often face challenges in maintaining high-quality data. Some common challenges include:
- Lack of data governance: Without proper data governance practices in place, it can be challenging to ensure data quality and consistency.
- Data integration issues: Integrating data from multiple sources can result in data quality issues, such as duplicate or inconsistent data.
- Data security concerns: Ensuring data security throughout the data lifecycle is crucial, as data breaches can compromise the quality and integrity of data.
- Legacy systems and technologies: Outdated systems and technologies may not have built-in data quality management capabilities, making it difficult to maintain high-quality data.
Organizations need to address these challenges and implement robust data quality management practices to ensure the success of AI and ML initiatives.
Best Practices for Data Quality Management in AI and ML
To overcome the challenges in data quality and maximize the effectiveness of AI and ML algorithms, organizations should follow these best practices:
- Implement a master data management strategy: By implementing a comprehensive MDM strategy, organizations can create a centralized source of high-quality master data that is shared across different systems and applications.
- Invest in MDM tools and technologies: MDM tools and technologies provide the necessary capabilities for data cleansing, data integration, and data governance, ensuring the quality and consistency of data used for AI and ML training.
- Establish data governance practices: Implementing data governance practices, including data quality standards, data lineage tracking, and data compliance, ensures that data used for AI and ML training is accurate, complete, and relevant.
- Ensure data security throughout the data lifecycle: Implementing robust data security measures, such as encryption, access controls, and data masking, helps protect the quality and integrity of data used for AI and ML training.
- Regularly monitor and evaluate data quality: Continuously monitoring and evaluating data quality helps identify and address any issues or anomalies that may affect the accuracy and reliability of AI and ML models.
Conclusion
Data quality plays a critical role in the success of AI and ML algorithms. High-quality data is essential for training accurate and reliable models, while poor data quality can lead to biased or inaccurate results. By implementing robust data quality management practices, such as master data management, data governance, and data security, organizations can ensure the effectiveness of AI and ML initiatives and unlock the full potential of these technologies. Investing in data quality is not only crucial for achieving accurate insights and predictions but also for maintaining customer trust and driving business growth.
Contact us
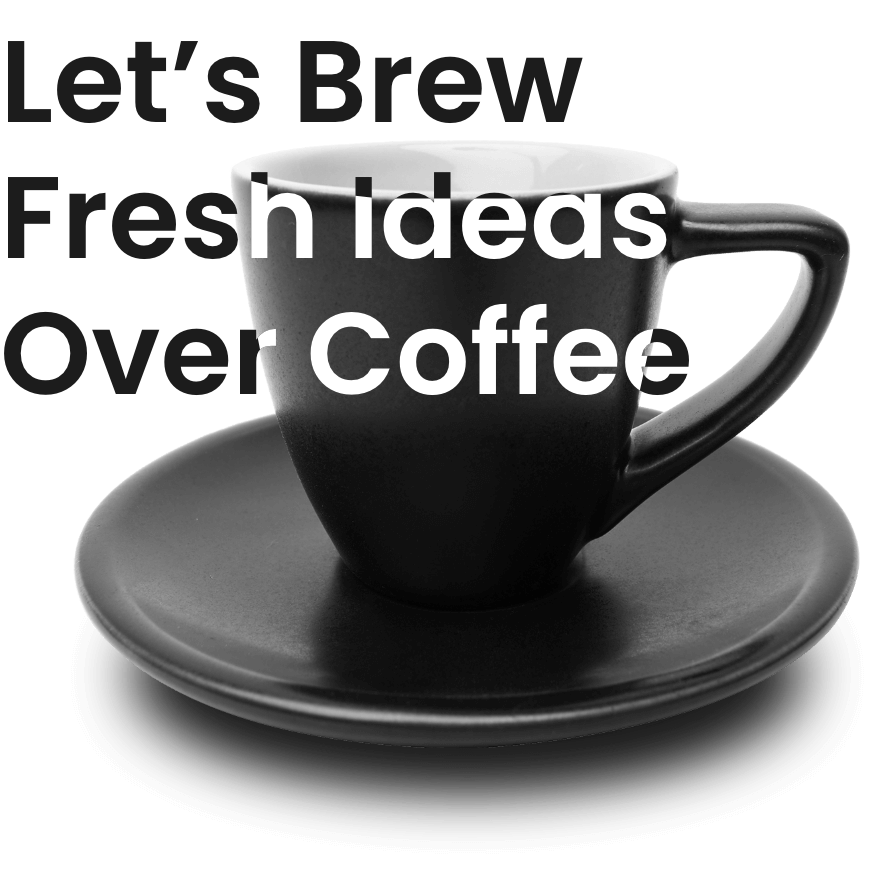
Spanning 8 cities worldwide and with partners in 100 more, we’re your local yet global agency.
Fancy a coffee, virtual or physical? It’s on us – let’s connect!