The impact of master data modeling on data quality
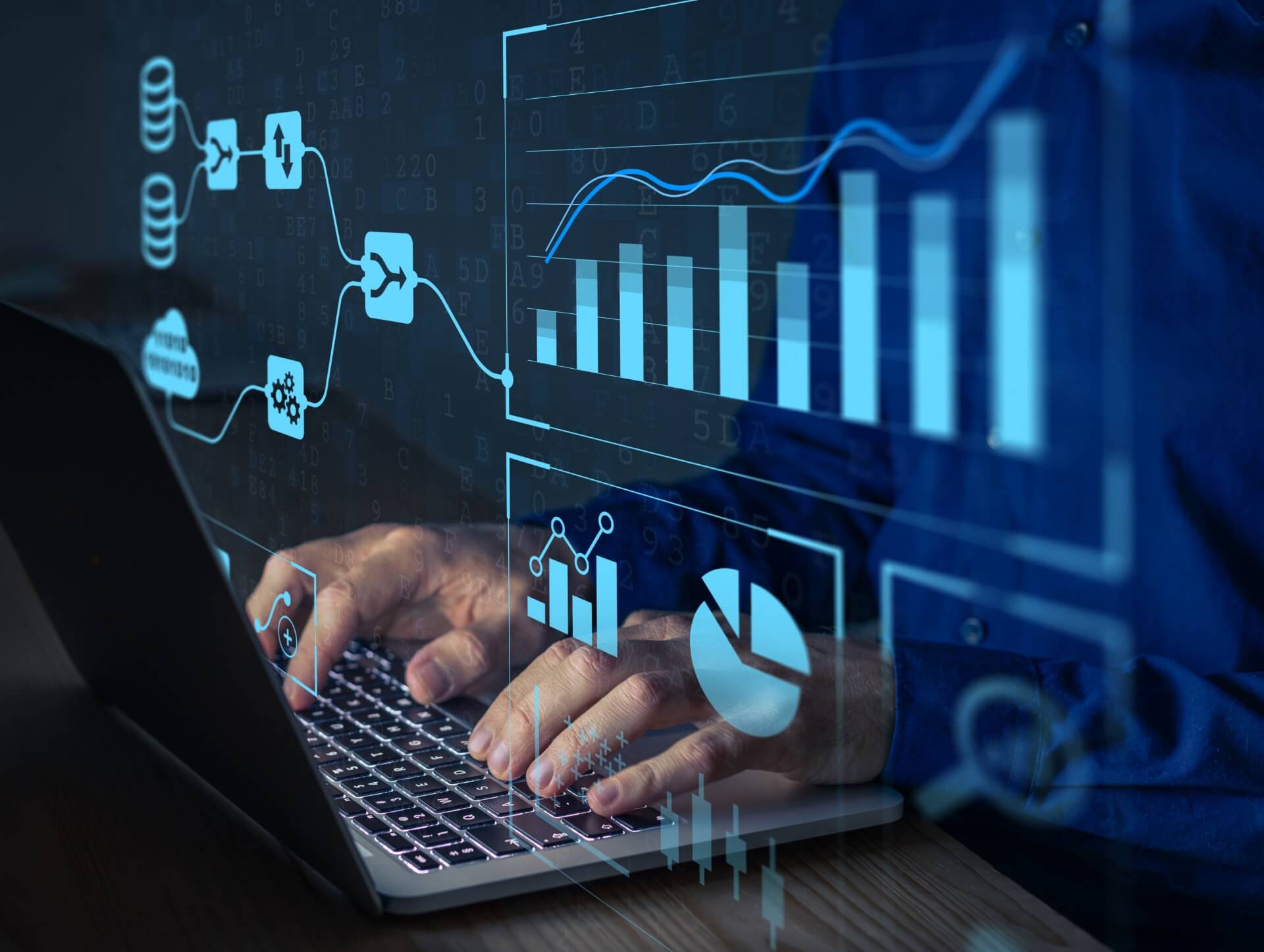
06/09/2023
Master Data Management (MDM) is a critical component of any organization's data strategy. It involves the management and governance of an organization's key data assets, known as master data, to ensure data consistency, accuracy, and reliability across various systems and processes. One of the key aspects of MDM is master data modeling, which plays a significant role in improving data quality. In this article, we will explore the impact of master data modeling on data quality and its significance in MDM.
The Significance of Data Quality
Data quality is crucial for organizations as it directly affects business decisions, operations, and customer satisfaction. Poor data quality can lead to erroneous insights, inefficient processes, and compromised customer experiences. Therefore, organizations need to invest in strategies and solutions to improve data quality. Master data modeling is one such strategy that can greatly contribute to enhancing data quality in MDM.
What is Master Data Modeling?
Master data modeling is the process of designing and creating a logical representation of an organization's key data entities and their relationships. It involves defining the structure, attributes, and hierarchies of master data entities such as customers, products, suppliers, and locations. Master data modeling provides a standardized and consistent framework for organizing and managing master data, ensuring data integrity and quality.
The Impact of Master Data Modeling on Data Quality
Master data modeling has a significant impact on data quality in MDM. Let's explore some of the key ways in which master data modeling improves data quality:
1. Standardization and Consistency
Master data modeling establishes a standardized and consistent structure for master data entities. It defines the attributes, relationships, and validation rules for each entity, ensuring data consistency across different systems and processes. By enforcing data standardization, master data modeling eliminates data redundancies, inconsistencies, and errors, resulting in improved data quality.
2. Data Integrity and Accuracy
Master data modeling enables the implementation of data integrity constraints and validation rules. These constraints ensure that only accurate and valid data is stored in the master data repository. By enforcing data integrity, master data modeling prevents data discrepancies, duplicates, and inaccuracies, leading to higher data quality.
3. Data Governance and Control
Master data modeling supports effective data governance and control in MDM. It provides a framework for defining data ownership, access controls, and data stewardship responsibilities. With clear data governance policies and controls in place, organizations can ensure that data is managed and maintained in accordance with regulatory requirements and industry best practices, resulting in improved data quality.
4. Data Integration and Interoperability
Master data modeling facilitates seamless data integration and interoperability across different systems and applications. By defining the relationships and dependencies between master data entities, organizations can ensure that data is accurately shared and synchronized across various systems. This enhances data consistency and reliability, leading to improved data quality.
Benefits of Master Data Modeling
Implementing master data modeling in MDM brings several benefits to organizations. Let's explore some of the key benefits:
1. Improved Data Consistency
Master data modeling establishes a standardized and consistent framework for organizing and managing master data. This ensures that data is consistently represented and used across different systems and processes, leading to improved data consistency.
2. Enhanced Data Accuracy
By enforcing data integrity constraints and validation rules, master data modeling helps maintain accurate and reliable data. This eliminates data discrepancies, duplicates, and inaccuracies, resulting in enhanced data accuracy.
3. Efficient Data Integration
Master data modeling facilitates seamless data integration and interoperability across various systems and applications. This enables organizations to efficiently share and synchronize data, leading to improved data integration.
4. Effective Data Governance
Master data modeling supports effective data governance in MDM. It provides a framework for defining data ownership, access controls, and data stewardship responsibilities. This ensures that data is managed and maintained in accordance with regulatory requirements and industry best practices.
Challenges in Master Data Modeling
While master data modeling offers numerous benefits, organizations may face certain challenges in its implementation. Let's discuss some of the key challenges:
1. Complex Data Structures
Organizations often deal with complex data structures and hierarchies. Designing a comprehensive master data model that accurately represents these complex structures can be challenging.
2. Data Governance and Stewardship
Establishing data governance policies and defining data stewardship responsibilities requires careful planning and coordination. Organizations need to ensure that the necessary resources and processes are in place to effectively govern master data.
3. Data Integration and Interoperability
Integrating master data with various systems and applications can be complex, especially when dealing with legacy systems or disparate data sources. Ensuring seamless data integration and interoperability requires careful mapping and transformation of data.
4. Change Management
Implementing master data modeling often involves significant changes in data management processes and systems. Organizations need to effectively manage the change and ensure proper training and support for users.
Best Practices for Master Data Modeling
To ensure successful implementation of master data modeling, organizations should follow these best practices:
1. Understand Business Requirements
Before designing a master data model, organizations should thoroughly understand their business requirements and data dependencies. This ensures that the model accurately represents the organization's data landscape.
2. Involve Stakeholders
Engage stakeholders from different business units and IT teams in the master data modeling process. This ensures that the model reflects the needs of various stakeholders and promotes collaboration.
3. Prioritize Data Quality
Data quality should be a top priority in master data modeling. Implement data validation rules, integrity constraints, and quality checks to ensure that only accurate and reliable data is stored in the master data repository.
4. Establish Data Governance
Define clear data governance policies and processes to ensure that master data is managed and maintained effectively. Assign data ownership, establish access controls, and implement data stewardship responsibilities.
The Future of Master Data Modeling
As organizations continue to recognize the importance of data quality and effective data management, the role of master data modeling will become even more critical. With advancements in technology and the increasing availability of master data management solutions, organizations can expect more sophisticated and automated approaches to master data modeling.
Conclusion
Master data modeling has a significant impact on data quality in MDM. By providing a standardized and consistent framework for organizing and managing master data, master data modeling improves data consistency, accuracy, and integrity. It enables efficient data integration, effective data governance, and seamless interoperability. Despite the challenges, organizations can benefit from implementing best practices in master data modeling to enhance data quality and drive business success.
Contact us
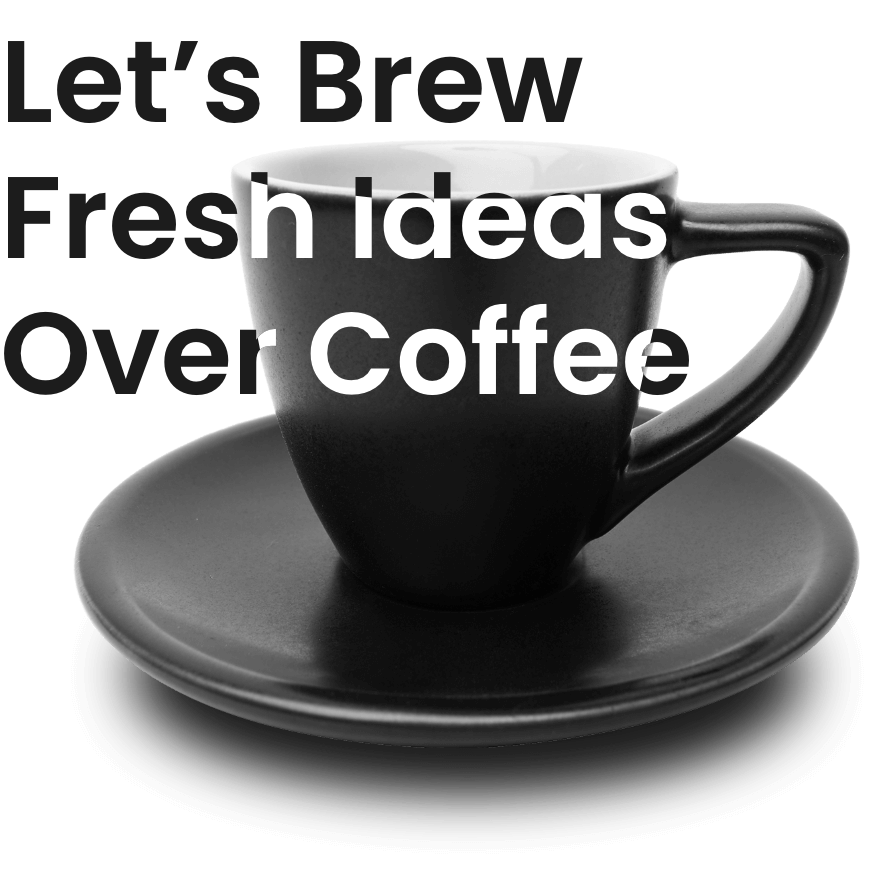
Spanning 8 cities worldwide and with partners in 100 more, we’re your local yet global agency.
Fancy a coffee, virtual or physical? It’s on us – let’s connect!