The impact of master data modeling on data quality in the automotive industry
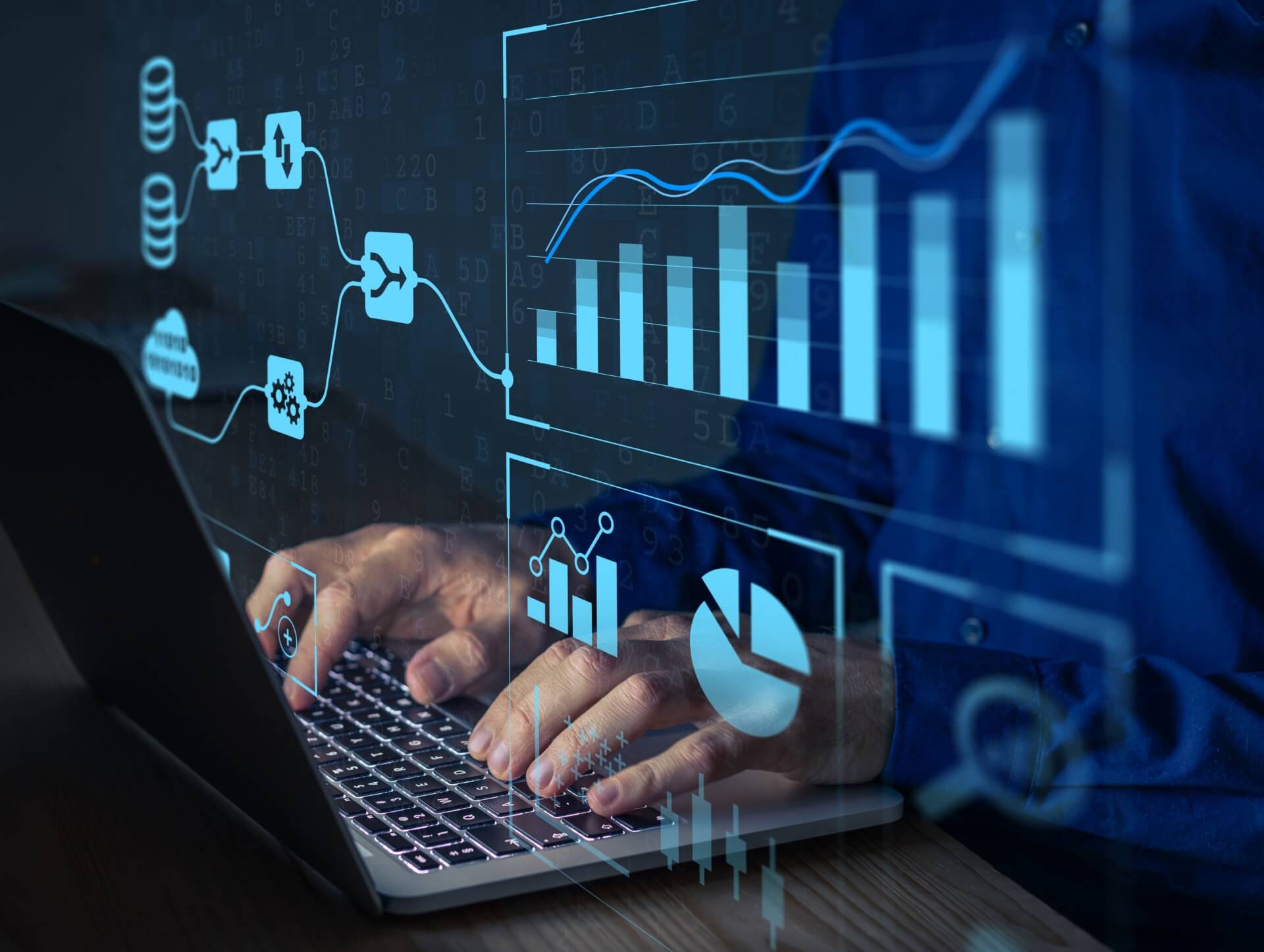
06/09/2023
In today's data-driven world, businesses across industries are recognizing the importance of quality data to drive informed decision-making and achieve operational excellence. The automotive industry is no exception, as it relies heavily on accurate and reliable data for various processes, including supply chain management, customer relationship management, and product development. One of the key strategies employed by automotive companies to ensure data quality is master data modeling.
What is Master Data Modeling?
Master data modeling refers to the process of designing and organizing the structure of master data entities within an organization. Master data represents the critical business entities such as products, customers, suppliers, and locations that are shared across multiple systems and applications. By creating a standardized and consistent model for master data, automotive companies can ensure that the data is accurate, up-to-date, and accessible to all relevant stakeholders.
The Benefits of Master Data Modeling
Implementing a comprehensive master data modeling strategy offers several benefits to the automotive industry:
1. Improved Data Quality
Master data modeling enables automotive companies to establish data quality standards and processes. By defining data attributes, validation rules, and data governance policies, organizations can ensure that the master data is accurate, complete, and consistent. This, in turn, enhances decision-making and reduces the risk of errors and inefficiencies in business operations.
2. Enhanced Data Integration
Master data modeling facilitates seamless integration of data across different systems and applications within the automotive enterprise. By creating a centralized master data management platform, organizations can eliminate data silos and ensure that all relevant stakeholders have access to consistent and reliable data. This enables better collaboration, improves efficiency, and supports data-driven decision-making.
3. Streamlined Business Processes
Master data modeling enables automotive companies to streamline their business processes by providing a single source of truth for critical data entities. By establishing clear data ownership, data governance processes, and data stewardship roles, organizations can ensure that data is accurate, reliable, and up-to-date. This streamlines processes such as order management, inventory management, and customer service, leading to improved operational efficiency and customer satisfaction.
4. Enhanced Data Security and Compliance
Master data modeling helps automotive companies to implement robust data security measures and ensure compliance with data privacy regulations. By defining access controls, data encryption standards, and data classification policies, organizations can protect sensitive information and mitigate the risk of data breaches. This helps build trust with customers and partners, and avoids potential legal and financial consequences of non-compliance.
Master Data Modeling and Data Quality Management
Master data modeling is closely linked to data quality management in the automotive industry. By implementing a robust master data management strategy, organizations can address common data quality challenges, such as:
1. Data Duplication
Master data modeling helps identify and eliminate data duplication within the automotive enterprise. By creating a centralized repository for master data, organizations can ensure that each data entity is unique and avoids redundancy. This reduces the risk of inconsistent and conflicting data, and improves data quality and integrity.
2. Incomplete or Inaccurate Data
Master data modeling enables organizations to define data attributes and validation rules, ensuring that data entities are complete and accurate. By implementing data governance processes and data stewardship roles, organizations can enforce data quality standards and resolve any issues related to incomplete or inaccurate data. This improves the reliability and usefulness of the data for decision-making.
3. Data Inconsistency
Master data modeling helps establish consistent data formats and structures across the automotive enterprise. By defining data standards and data validation rules, organizations can ensure that data is consistent and compatible across different systems and applications. This reduces the risk of data inconsistencies and improves data quality and reliability.
4. Data Integration Challenges
Master data modeling enables seamless integration of data across different systems and applications within the automotive enterprise. By creating a standardized model for master data, organizations can ensure that data is compatible and can be easily integrated and shared across various systems. This improves data quality and supports efficient data-driven processes.
Best Practices for Master Data Modeling in the Automotive Industry
Implementing an effective master data modeling strategy requires careful planning and adherence to best practices. Here are some key considerations for automotive companies:
1. Define Clear Data Governance Policies
Establishing clear data governance policies is crucial for effective master data modeling. This includes defining data ownership, data stewardship roles, and data governance processes. By having clear guidelines and responsibilities, organizations can ensure that data is managed effectively and consistently across the enterprise.
2. Engage Stakeholders
Involve relevant stakeholders from different departments and functions in the master data modeling process. This includes representatives from IT, business units, data governance teams, and data stewards. By engaging stakeholders early on, organizations can ensure that the master data model reflects the needs and requirements of the entire enterprise.
3. Establish Data Quality Metrics
Define data quality metrics and key performance indicators (KPIs) to measure the effectiveness of the master data modeling strategy. This includes metrics such as data accuracy, data completeness, and data consistency. Regularly monitor and report on these metrics to identify areas for improvement and ensure continuous data quality management.
4. Invest in Training and Education
Provide training and education to employees on the importance of master data modeling and data quality management. This includes educating employees on data governance processes, data stewardship roles, and data quality standards. By building a data-driven culture within the organization, organizations can ensure that data is managed effectively and consistently.
Conclusion
Master data modeling plays a critical role in ensuring data quality in the automotive industry. By implementing a comprehensive master data management strategy and adhering to best practices, organizations can improve data quality, enhance data integration, streamline business processes, and ensure data security and compliance. This, in turn, enables informed decision-making, operational excellence, and improved customer satisfaction. As the automotive industry continues to embrace digital transformation, master data modeling will become increasingly important for driving success in a data-driven world.
Contact us
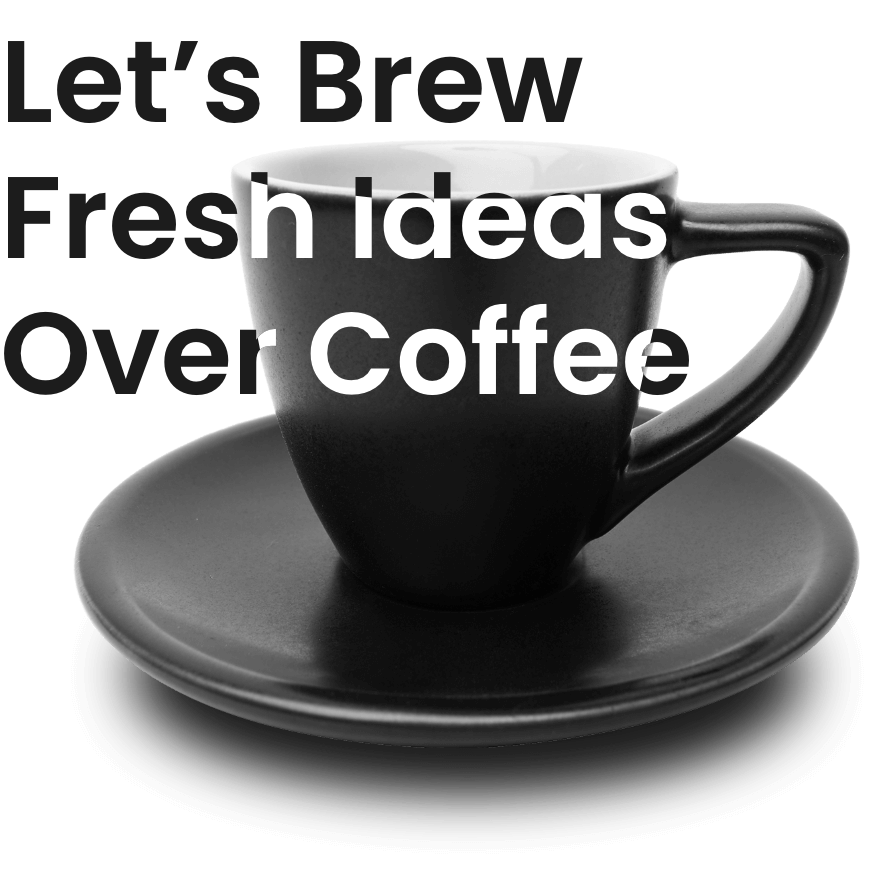
Spanning 8 cities worldwide and with partners in 100 more, we’re your local yet global agency.
Fancy a coffee, virtual or physical? It’s on us – let’s connect!