The impact of master data modeling on data quality in the manufacturing industry
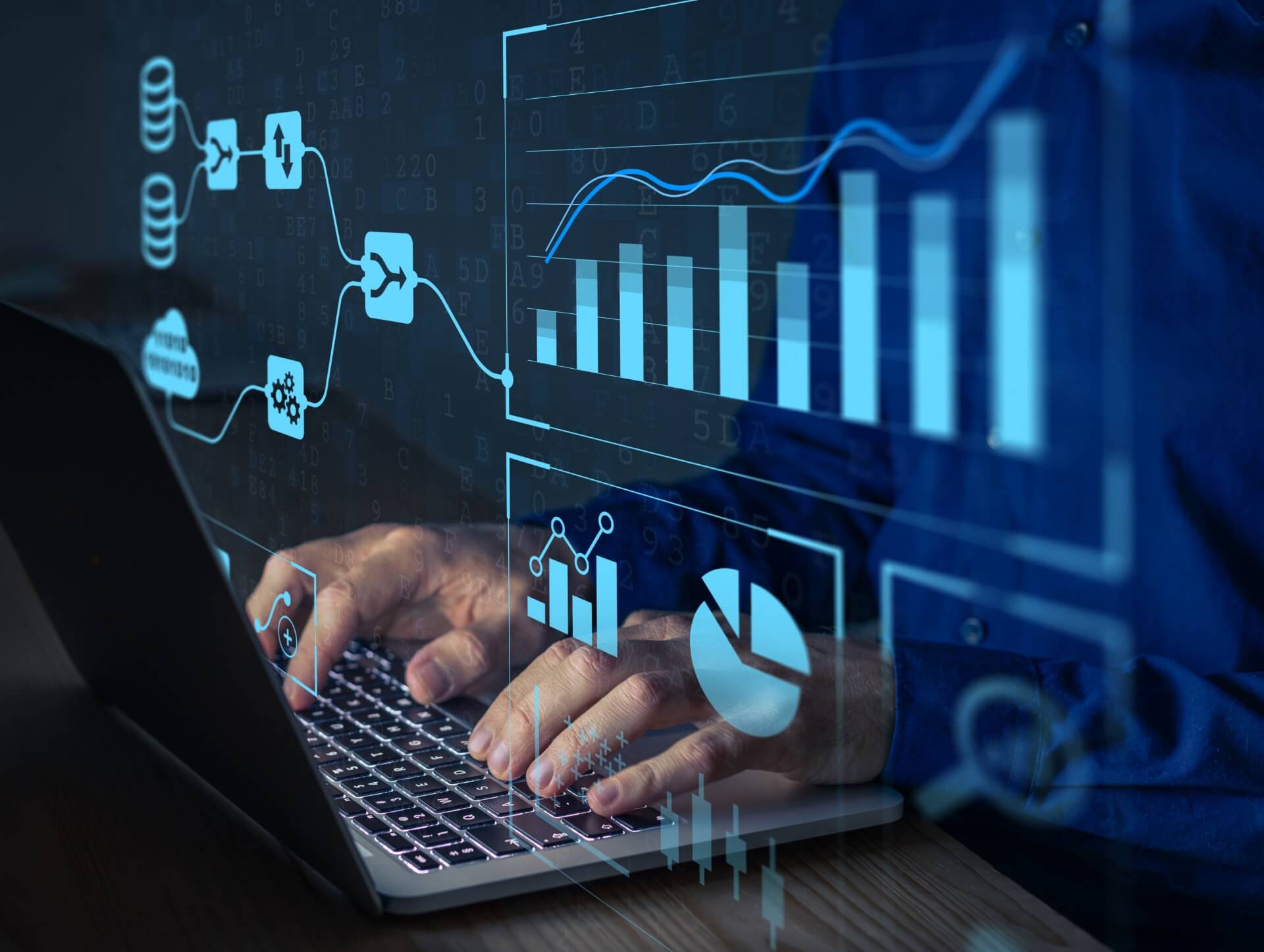
06/09/2023
In today's data-driven world, the manufacturing industry is increasingly relying on accurate and reliable data to make informed decisions, optimize operations, and enhance customer experiences. However, managing large volumes of data from multiple sources can be challenging, especially when it comes to maintaining data quality and consistency. This is where master data modeling plays a crucial role.
What is Master Data Modeling?
Master data modeling is the process of designing and creating a logical representation of an organization's master data. Master data refers to the core data elements that are shared across different business processes and systems, such as product information, customer data, supplier information, and employee records. By creating a standardized and consistent model for master data, organizations can ensure data quality and integrity throughout the data lifecycle.
The Importance of Data Quality in the Manufacturing Industry
Data quality is of utmost importance in the manufacturing industry as it directly impacts the efficiency and effectiveness of various business processes. Poor data quality can lead to production delays, inventory inaccuracies, supply chain disruptions, and ultimately, dissatisfied customers. On the other hand, high-quality data enables manufacturers to make accurate demand forecasts, optimize inventory levels, improve product quality, and provide personalized customer experiences.
Benefits of Master Data Modeling in the Manufacturing Industry
Implementing a robust master data modeling strategy in the manufacturing industry can yield several benefits:
Improved Data Accuracy and Consistency
Master data modeling ensures that data is captured, stored, and maintained consistently across different systems and processes. By eliminating duplicates, inconsistencies, and errors, organizations can rely on accurate and reliable data for decision-making and operational processes.
Enhanced Data Integration and Interoperability
A well-designed master data model enables seamless integration and interoperability between different systems and applications. This allows manufacturers to streamline data flows, facilitate data sharing, and enable real-time data synchronization, leading to improved collaboration and operational efficiency.
Effective Data Governance and Compliance
Master data modeling provides a solid foundation for implementing data governance and compliance frameworks. By defining data ownership, data stewardship roles, and data quality rules, organizations can ensure data privacy, security, and compliance with regulatory requirements.
Increased Operational Efficiency and Cost Savings
By eliminating data inconsistencies and redundancies, master data modeling helps manufacturers streamline their business processes, reduce manual data entry, and improve overall operational efficiency. This can result in significant cost savings and resource optimization.
Challenges in Implementing Master Data Modeling
While the benefits of master data modeling in the manufacturing industry are substantial, implementing an effective master data management (MDM) strategy can be challenging. Some of the common challenges include:
Data Quality Management
Ensuring data quality requires ongoing monitoring, measurement, and improvement efforts. Organizations need to establish data quality metrics, implement data cleansing and enrichment processes, and continuously monitor data quality to maintain the integrity of the master data model.
Data Governance and Ownership
Implementing effective data governance requires defining clear roles, responsibilities, and processes for data ownership, stewardship, and decision-making. Organizations need to establish data governance frameworks and policies to ensure the long-term success of the master data model.
Data Integration and System Compatibility
Integrating data from multiple sources and systems can be complex, especially in large manufacturing organizations with legacy systems and heterogeneous IT landscapes. Ensuring system compatibility, data mapping, and data transformation are critical for successful master data modeling.
Change Management and User Adoption
Implementing a new master data model requires organizational change management and user adoption strategies. Employees need to be trained on new data management processes, tools, and technologies to ensure successful implementation and usage of the master data model.
Best Practices for Master Data Modeling in the Manufacturing Industry
To maximize the benefits of master data modeling and ensure successful implementation, manufacturers should consider the following best practices:
Define a Clear MDM Strategy and Roadmap
Before embarking on master data modeling, organizations should define a clear MDM strategy and roadmap. This includes identifying key business objectives, defining data governance policies, establishing data quality metrics, and outlining the implementation plan.
Engage Stakeholders and Subject Matter Experts
Master data modeling requires cross-functional collaboration and involvement from various stakeholders and subject matter experts. Engaging key stakeholders early in the process ensures that the master data model aligns with business requirements and incorporates diverse perspectives.
Start with a Pilot Project
Implementing master data modeling across an entire organization can be overwhelming. Starting with a pilot project allows organizations to test the effectiveness of the master data model, identify potential challenges, and make necessary adjustments before scaling up.
Leverage MDM Tools and Technologies
There are various MDM tools and technologies available in the market that can streamline the master data modeling process. These tools provide features such as data profiling, data cleansing, data integration, and data governance, making it easier for manufacturers to implement and manage their master data model.
Establish Data Governance and Stewardship Processes
Data governance and stewardship are critical for maintaining the integrity and quality of the master data model. Organizations should establish clear data governance frameworks, define data stewardship roles and responsibilities, and implement processes for data validation, cleansing, and enrichment.
Continuously Monitor and Improve Data Quality
Data quality is an ongoing process that requires continuous monitoring and improvement. Organizations should establish data quality metrics, implement data profiling and cleansing processes, and regularly assess the accuracy, completeness, and consistency of the master data model.
Provide Training and Support to Users
Effective user adoption is crucial for the success of the master data model. Organizations should provide comprehensive training and ongoing support to users to ensure that they understand the benefits of the master data model and are proficient in using the associated tools and technologies.
Case Studies: Successful Implementation of Master Data Modeling in the Manufacturing Industry
Several manufacturing organizations have successfully implemented master data modeling to improve data quality and achieve operational excellence. Here are a few case studies:
Case Study 1: Company X
Company X, a global automotive manufacturer, implemented a master data management solution to address data quality issues across its supply chain. By creating a centralized master data model for its suppliers, products, and materials, Company X was able to eliminate duplicate records, improve data accuracy, and optimize inventory management. This resulted in significant cost savings and improved customer satisfaction.
Case Study 2: Company Y
Company Y, a leading consumer goods manufacturer, adopted a cloud-based master data management platform to streamline its product information management processes. By implementing a standardized master data model for its product catalogs, Company Y was able to improve data consistency, accelerate time-to-market for new products, and enhance the customer experience. This led to increased sales and improved brand reputation.
Case Study 3: Company Z
Company Z, a global electronics manufacturer, implemented an open-source master data management solution to address data integration challenges across its diverse IT landscape. By leveraging the flexibility and scalability of the open-source platform, Company Z was able to integrate data from multiple systems, ensure data consistency, and enable real-time analytics. This resulted in improved operational efficiency and better decision-making.
Conclusion
Master data modeling plays a crucial role in improving data quality and enabling operational excellence in the manufacturing industry. By implementing a robust master data management strategy, manufacturers can ensure data accuracy, enhance data integration, facilitate data governance, and achieve significant cost savings. While there are challenges in implementing master data modeling, following best practices and learning from successful case studies can help organizations overcome these challenges and reap the benefits of effective master data modeling.
Contact us
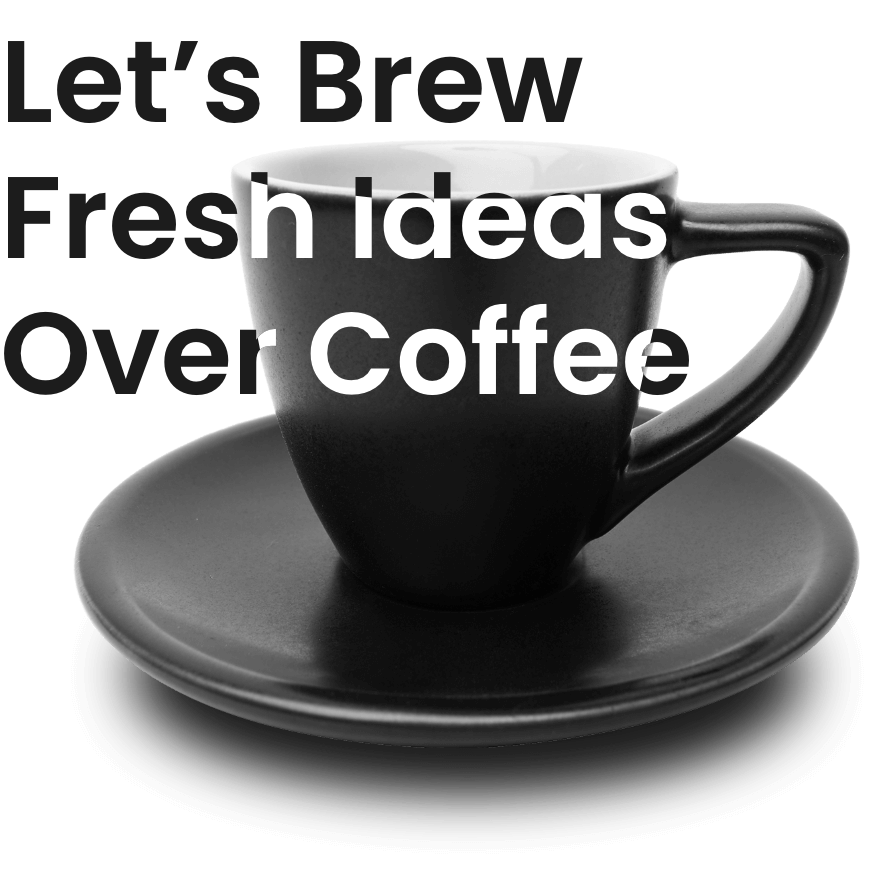
Spanning 8 cities worldwide and with partners in 100 more, we’re your local yet global agency.
Fancy a coffee, virtual or physical? It’s on us – let’s connect!