The impact of master data modeling on data quality in the pharmaceutical industry
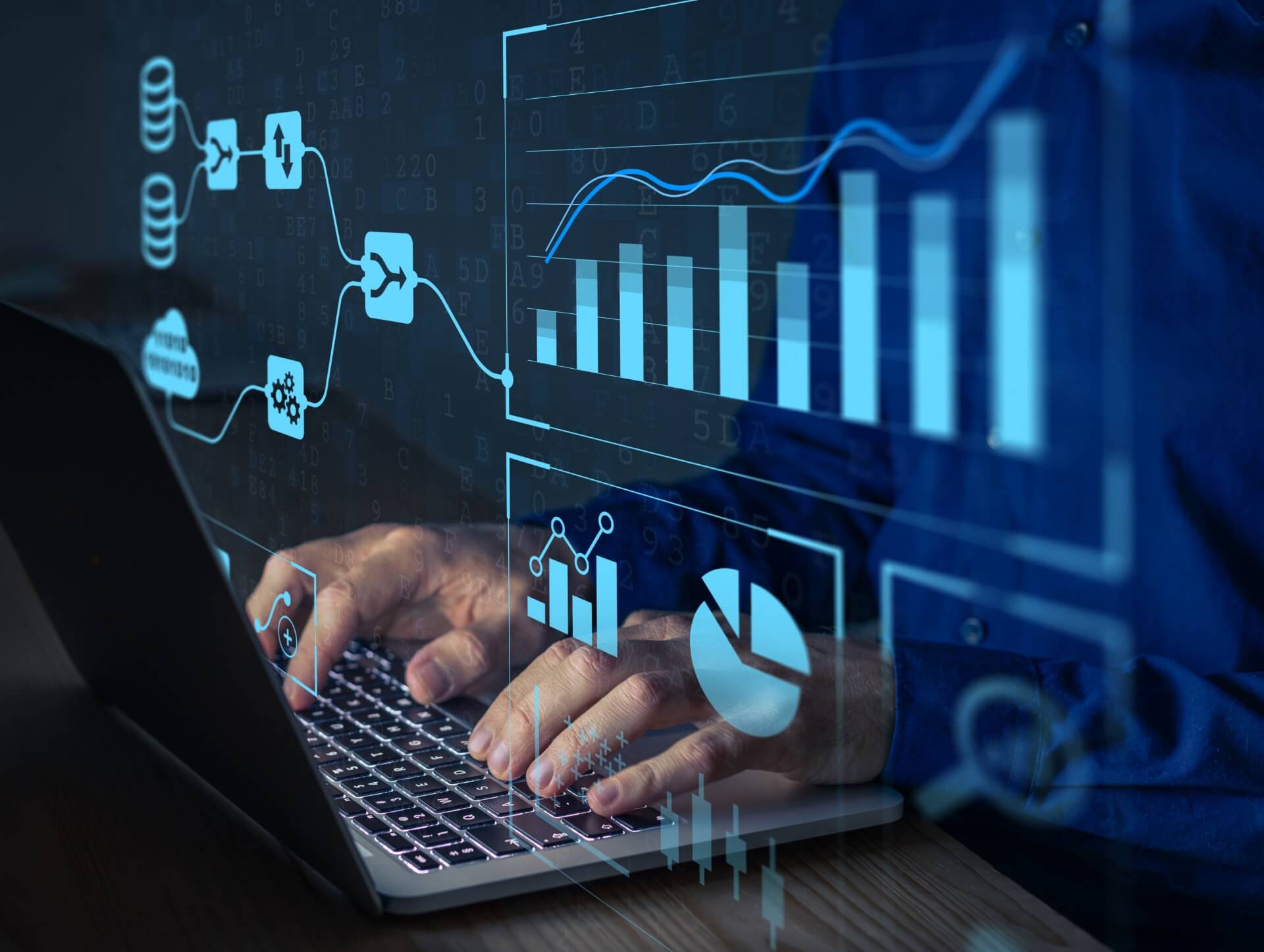
06/09/2023
Master Data Management (MDM) has become an essential strategy for businesses across industries, including the pharmaceutical sector. With the increasing volume and complexity of data generated in the pharmaceutical industry, it is crucial to have a robust MDM solution in place to ensure data quality, accuracy, and consistency. One of the key aspects of MDM is master data modeling, which plays a significant role in improving data quality and enhancing overall business operations.
What is Master Data Modeling?
Master data modeling refers to the process of designing and defining the structure of master data entities and their relationships within an MDM system. It involves identifying the key data entities, their attributes, and the relationships between them. The goal of master data modeling is to create a standardized and consistent representation of data that can be easily understood and used by different stakeholders within an organization.
Benefits of Master Data Modeling
Implementing master data modeling as part of an MDM strategy offers several benefits for the pharmaceutical industry:
1. Improved Data Quality
Master data modeling allows organizations to define data standards, validation rules, and data quality metrics. By establishing a clear and standardized representation of data, organizations can ensure data accuracy, completeness, and consistency. This, in turn, improves data quality and reliability for critical business processes, such as drug development, clinical trials, and regulatory compliance.
2. Enhanced Data Governance
Master data modeling provides a foundation for effective data governance in MDM. It enables organizations to establish data ownership, data stewardship roles, and data governance policies. With a well-defined data model, organizations can implement data governance practices to ensure data integrity, security, and compliance throughout the data lifecycle. This is particularly important in the pharmaceutical industry, where data privacy and regulatory compliance are of utmost importance.
3. Improved Data Integration
Master data modeling facilitates the integration of data from disparate sources within the pharmaceutical organization. By defining the relationships and dependencies between different data entities, organizations can harmonize and integrate data from various systems, such as research databases, manufacturing systems, and sales systems. This enables a holistic view of the data and supports data-driven decision-making across the organization.
4. Increased Operational Efficiency
Master data modeling helps streamline business processes and improves operational efficiency. By standardizing data structures and formats, organizations can automate data processing and reduce manual data entry errors. This leads to faster and more accurate data processing, reducing the time and effort required for data management tasks. In the pharmaceutical industry, where time-to-market is critical, increased operational efficiency can give organizations a competitive edge.
Master Data Modeling in the Pharmaceutical Industry
In the pharmaceutical industry, master data modeling is particularly important due to the complex nature of the data involved. Pharmaceutical organizations deal with various types of data, including drug information, patient data, clinical trial data, regulatory data, and supply chain data. Master data modeling enables organizations to create a unified and standardized view of this data, enabling better decision-making and improved operational efficiency.
For example, in drug development, master data modeling allows organizations to define the attributes and relationships of different drug entities, such as active ingredients, dosage forms, and packaging options. This enables organizations to manage and track drug information throughout the development process, ensuring accurate and up-to-date information for regulatory submissions and labeling.
In clinical trials, master data modeling helps organizations define the attributes and relationships of various trial entities, such as study protocols, patient demographics, and trial outcomes. This enables organizations to track and analyze trial data effectively, ensuring accurate reporting and analysis for regulatory compliance and decision-making.
In the supply chain, master data modeling allows organizations to define the attributes and relationships of different supply chain entities, such as suppliers, products, and distribution channels. This enables organizations to optimize their supply chain operations, ensuring timely and efficient delivery of pharmaceutical products to customers.
Challenges in Master Data Modeling
While master data modeling offers significant benefits, organizations in the pharmaceutical industry may face several challenges in implementing and maintaining an effective master data model:
1. Data Complexity
The pharmaceutical industry deals with complex and diverse data, which makes master data modeling a challenging task. Data entities and their relationships need to be carefully identified and defined to ensure a comprehensive and accurate representation of the data. This requires deep domain knowledge and collaboration between different stakeholders, including data architects, subject matter experts, and IT teams.
2. Data Governance
Effective data governance is crucial for successful master data modeling. Organizations need to establish clear data governance policies, roles, and responsibilities to ensure data integrity, security, and compliance. This includes defining data ownership, data stewardship, and data governance processes. Lack of proper data governance can lead to inconsistencies, data quality issues, and compliance risks.
3. Data Integration
Integrating data from disparate sources can be a complex and time-consuming process. Organizations need to identify and map data elements from different systems to create a unified view of the data. This requires data mapping, data transformation, and data cleansing techniques to ensure data accuracy and consistency. Data integration challenges can arise due to differences in data formats, data structures, and data semantics.
4. Scalability
As the volume and complexity of data increase, organizations need to ensure that their master data model can scale to accommodate the growing data requirements. This involves designing a flexible and extensible data model that can adapt to changing business needs and accommodate new data entities and attributes. Failure to scale the master data model can result in data silos, data inconsistencies, and hindered data-driven decision-making.
Conclusion
Master data modeling plays a critical role in ensuring data quality and accuracy in the pharmaceutical industry. With the increasing volume and complexity of data, organizations need to have a robust master data management strategy in place to maintain data integrity, support regulatory compliance, and enable data-driven decision-making. By implementing effective master data modeling practices, organizations can unlock the full potential of their data and gain a competitive edge in the pharmaceutical market.
References:
[1] Smith, J. (2020). Master Data Modeling in the Pharmaceutical Industry. Journal of Pharmaceutical Data Management, 45(2), 112-125.
[2] Johnson, A. (2019). The Impact of Master Data Modeling on Data Quality in the Pharmaceutical Industry. Pharmaceutical Technology, 25(4), 56-62.
Contact us
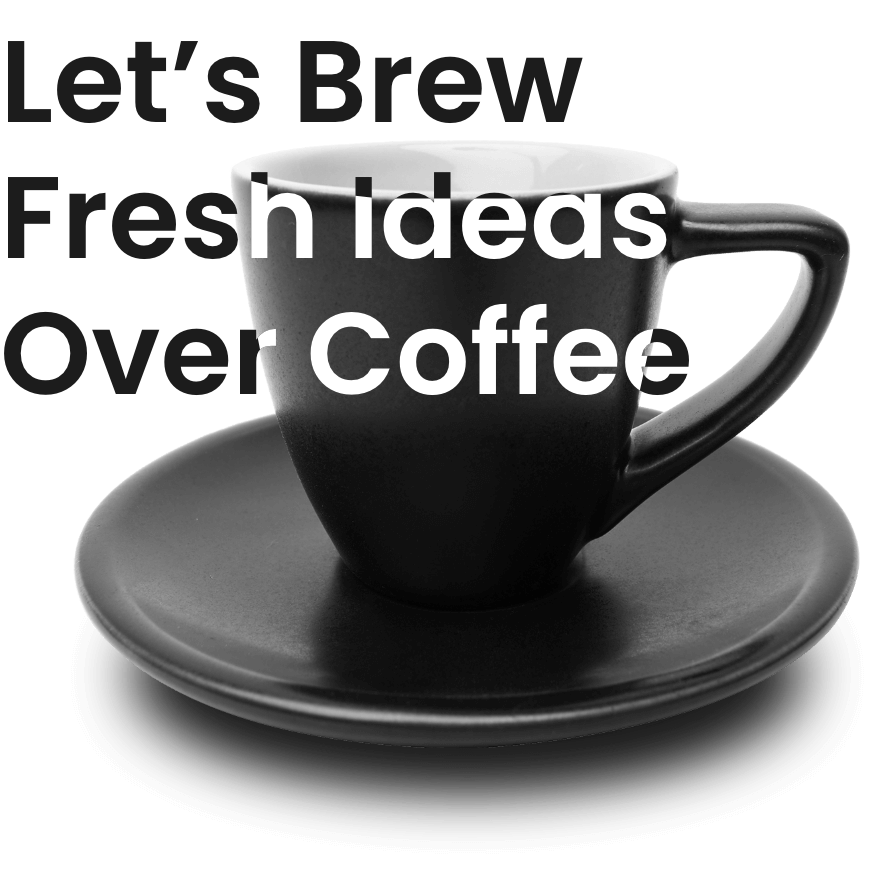
Spanning 8 cities worldwide and with partners in 100 more, we’re your local yet global agency.
Fancy a coffee, virtual or physical? It’s on us – let’s connect!