The impact of MDM implementation on data governance maturity models in cloud environments
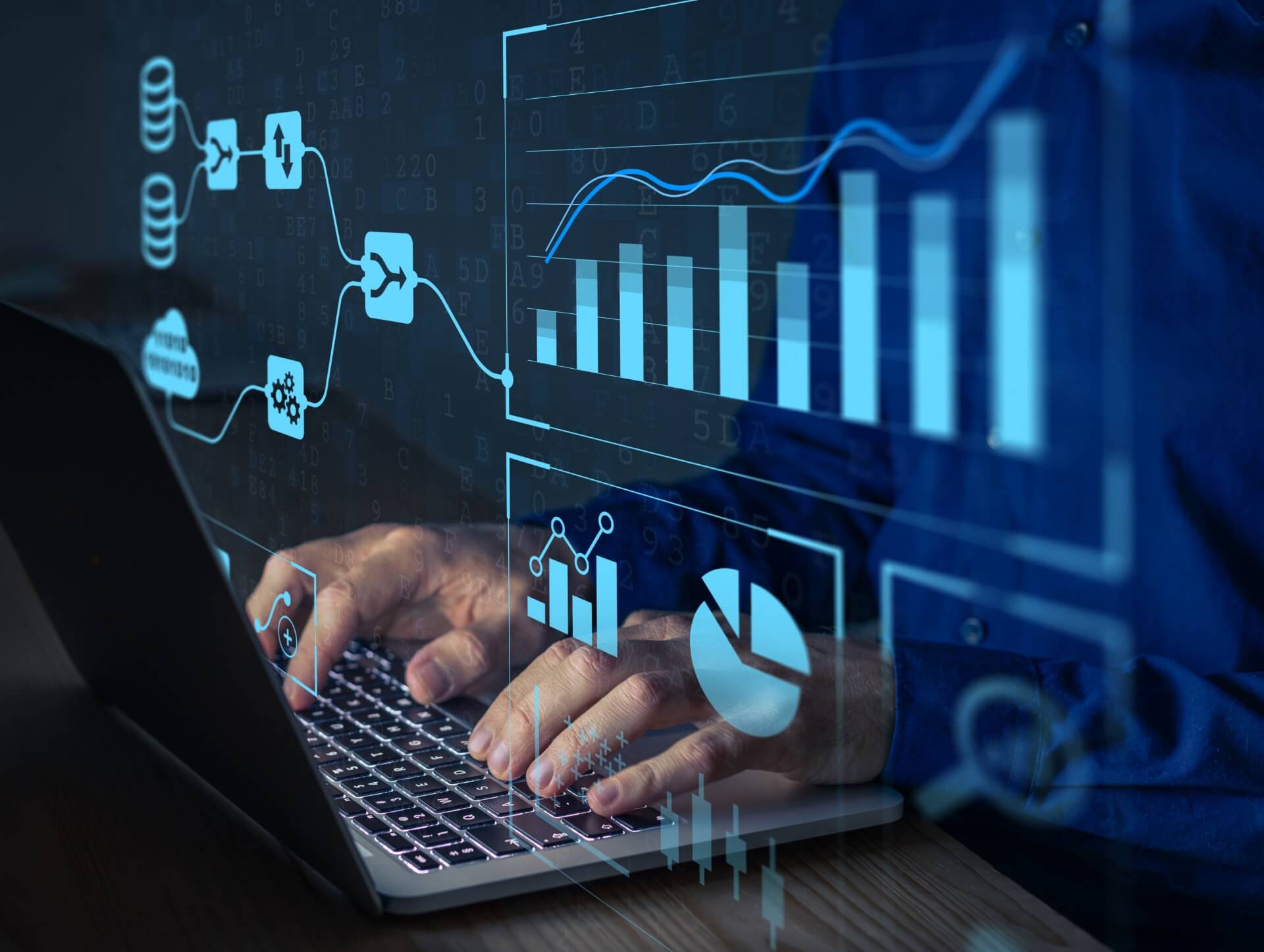
06/09/2023
In today's data-driven world, organizations are constantly dealing with vast amounts of data that need to be managed effectively. A key aspect of data management is master data management (MDM), which involves the consolidation, cleansing, and synchronization of data from various sources to create a single, unified view of the data.
Cloud computing has revolutionized the way organizations store, access, and manage their data. With the increasing adoption of cloud-based solutions, it is crucial to understand the impact of MDM implementation on data governance maturity models in cloud environments.
Benefits of Master Data Management
Implementing a robust master data management strategy offers several benefits to organizations:
- Improved data quality: MDM ensures that data is accurate, consistent, and up-to-date, leading to better decision-making and operational efficiency.
- Enhanced data governance: MDM enables organizations to establish data governance policies and procedures, ensuring data compliance and security throughout its lifecycle.
- Increased customer satisfaction: By having a single, accurate view of customer data, organizations can provide personalized and consistent experiences, leading to improved customer satisfaction and loyalty.
- Streamlined business processes: MDM eliminates data silos and enables data integration across systems, resulting in streamlined business processes and improved productivity.
- Cost savings: By reducing data redundancy and improving data quality, MDM helps organizations save costs associated with data management and maintenance.
MDM Implementation Process
Implementing an MDM solution involves several key steps:
- Assessment and Planning: Organizations need to assess their data management needs, identify data sources, and define their MDM goals and objectives.
- Data Profiling and Cleansing: Data profiling is performed to understand the quality and structure of the data. Cleansing processes are then applied to remove duplicates, correct errors, and standardize data.
- Data Integration: Data from various sources is integrated into a centralized MDM platform, ensuring data consistency and accuracy.
- Data Governance: Data governance policies and procedures are established to ensure data compliance, security, and privacy.
- Master Data Modeling: Organizations define their master data models, including entities, attributes, and relationships.
- Data Synchronization: MDM solutions enable data synchronization across systems, ensuring that all systems have access to the most up-to-date and accurate data.
- Data Quality Management: Data quality is continuously monitored and measures are taken to improve data accuracy and consistency.
- Change Management: Organizations need to manage changes to data structures, processes, and policies to ensure the smooth functioning of the MDM solution.
Data Governance in MDM
Data governance plays a critical role in MDM implementation and ensures the effective management and control of data throughout its lifecycle. It encompasses the processes, policies, and standards that govern how data is created, stored, used, and shared within an organization.
Effective data governance in MDM involves:
- Defining data governance roles and responsibilities: Organizations need to identify data stewards and data owners who are responsible for managing and maintaining the quality and integrity of the data.
- Establishing data governance policies and procedures: Policies and procedures need to be defined to ensure data compliance, security, and privacy. This includes data classification, access controls, and data retention policies.
- Implementing data governance tools and technologies: MDM platforms provide data governance capabilities, such as data lineage, data quality monitoring, and data access controls.
- Ensuring data quality management: Data quality management processes need to be established to monitor and improve the quality of the data. This includes data profiling, data cleansing, and data enrichment.
- Enabling data governance throughout the data lifecycle: Data governance should be integrated into each phase of the data lifecycle, from data creation to data archiving. This ensures that data is managed consistently and in compliance with regulatory requirements.
- Continuous monitoring and improvement: Data governance processes and policies should be continuously monitored and improved to adapt to changing business needs and regulatory requirements.
Challenges in MDM Implementation
Implementing an MDM solution in a cloud environment comes with its own set of challenges:
- Data integration in a multi-cloud environment: Organizations may have data stored in different cloud platforms, making data integration complex and challenging.
- Data security and privacy: Organizations need to ensure that data stored in the cloud is secure and compliant with data protection regulations.
- Data governance across cloud platforms: Managing data governance policies and procedures across multiple cloud platforms can be challenging, as each platform may have its own data governance capabilities.
- Data quality management: Ensuring data quality in a cloud environment requires robust data quality management processes and tools.
- Data compliance and regulatory requirements: Organizations need to ensure that data stored in the cloud is compliant with regulatory requirements, such as GDPR and HIPAA.
- Data scalability: MDM solutions need to be scalable to handle large volumes of data in a cloud environment.
- Change management: Implementing an MDM solution requires changes to data structures, processes, and policies, which can be challenging to manage in a cloud environment.
MDM and Data Governance Maturity Models
Data governance maturity models provide a framework for organizations to assess their data governance capabilities and identify areas for improvement. The implementation of an MDM solution can have a significant impact on the maturity level of an organization's data governance.
MDM enables organizations to establish a solid foundation for data governance by providing a centralized platform for data management. It ensures data consistency, accuracy, and integrity, which are essential for effective data governance.
MDM also enables organizations to automate data governance processes, such as data quality monitoring, data lineage tracking, and data access controls. This improves the efficiency and effectiveness of data governance, leading to higher maturity levels.
MDM and Data Lifecycle Management
Data lifecycle management (DLM) involves the management of data from its creation to its archival or disposal. MDM plays a crucial role in data lifecycle management by ensuring the quality, consistency, and integrity of master data throughout its lifecycle.
Effective data lifecycle management with MDM involves:
- Data security throughout the lifecycle: MDM ensures that master data is secure and protected from unauthorized access or data breaches throughout its lifecycle.
- Data compliance in management: MDM helps organizations comply with data protection regulations and industry standards throughout the data lifecycle.
- Master data in lifecycle management: MDM ensures that master data is managed consistently and in compliance with data governance policies and procedures throughout its lifecycle.
- Metadata management in data lifecycle: MDM enables organizations to effectively manage metadata, such as data definitions, data lineage, and data relationships, throughout the data lifecycle.
- Automation in data lifecycle: MDM automates data management processes, such as data quality monitoring and data synchronization, throughout the data lifecycle, improving efficiency and reducing manual effort.
- Cloud-based data lifecycle: MDM in a cloud environment enables organizations to leverage the scalability, flexibility, and cost-effectiveness of cloud computing for data lifecycle management.
- Analytics in data lifecycle: MDM provides organizations with accurate and consistent data for analytics and reporting throughout the data lifecycle, enabling better decision-making and insights.
- Challenges in data lifecycle management: Implementing effective data lifecycle management with MDM comes with challenges, such as managing data across multiple systems and platforms, ensuring data quality, and addressing data privacy concerns.
- Best practices for lifecycle management: Organizations should follow best practices, such as defining clear data governance policies, implementing data quality management processes, and leveraging automation and analytics tools, to ensure effective data lifecycle management with MDM.
- ROI of effective data lifecycle: Implementing effective data lifecycle management with MDM can result in higher ROI by improving data quality, reducing data management costs, enabling better decision-making, and ensuring regulatory compliance.
Conclusion
Master data management plays a crucial role in data governance maturity models in cloud environments. It provides organizations with a robust platform for data management, ensuring data quality, consistency, and integrity. MDM enables organizations to establish effective data governance policies and procedures, ensuring data compliance, security, and privacy throughout the data lifecycle.
Implementing an MDM solution in a cloud environment comes with its own set of challenges, such as data integration, data security, and data governance across multiple cloud platforms. However, by following best practices and leveraging MDM tools and technologies, organizations can overcome these challenges and realize the benefits of effective MDM and data governance in cloud environments.
Contact us
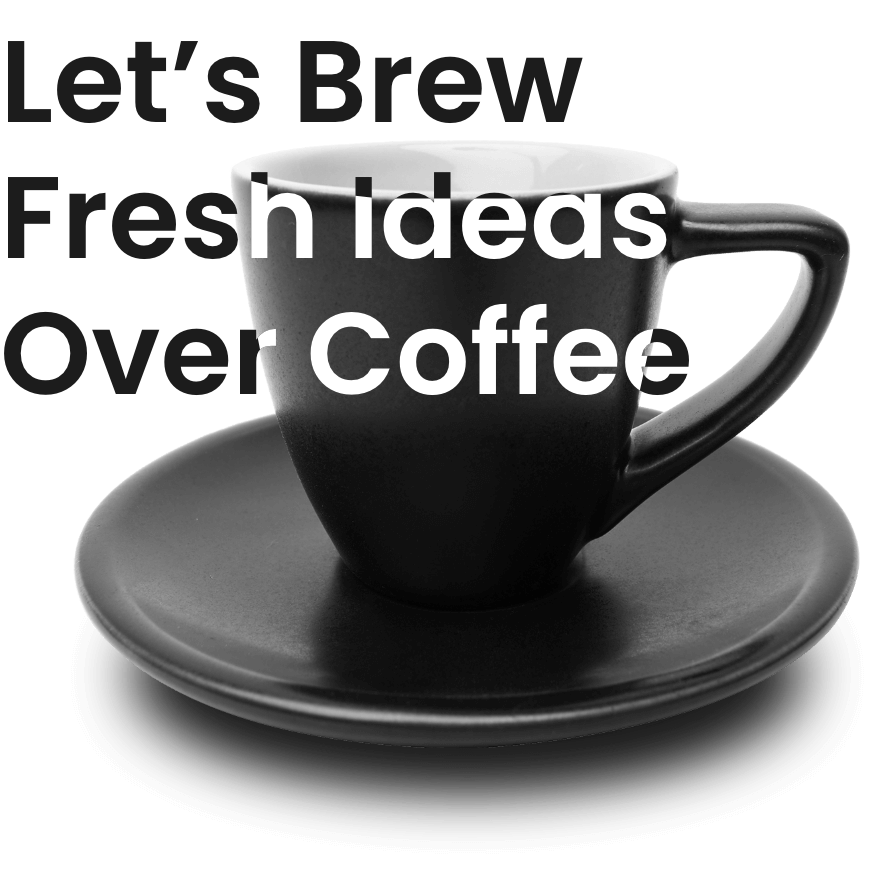
Spanning 8 cities worldwide and with partners in 100 more, we’re your local yet global agency.
Fancy a coffee, virtual or physical? It’s on us – let’s connect!