The impact of MDM implementation on data governance maturity models in hybrid environments
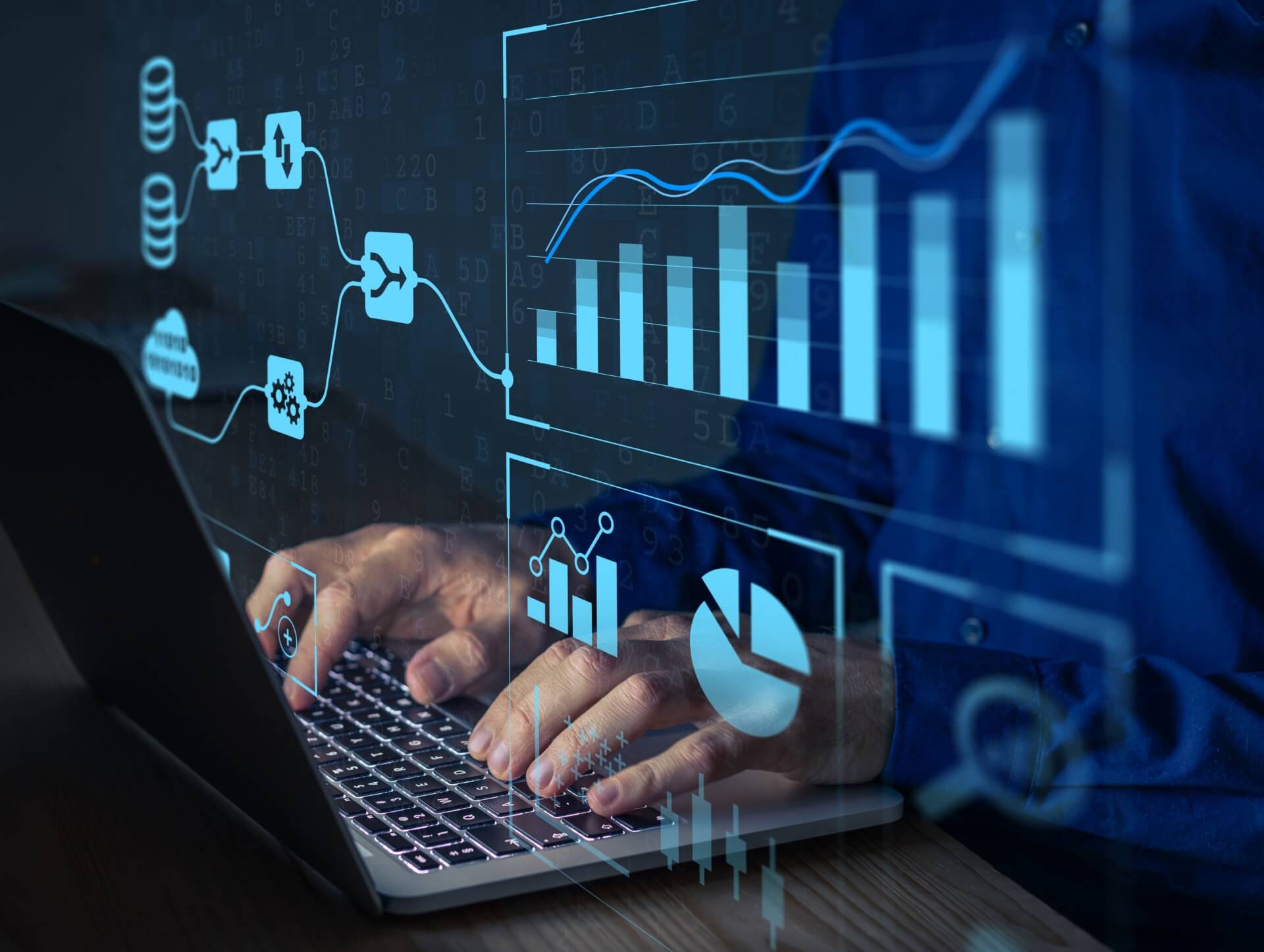
06/09/2023
Master Data Management (MDM) has become an essential component of modern data management strategies. It involves the processes, tools, and technologies used to create and maintain a single, trusted, and accurate view of master data within an organization. Master data includes critical business information such as customer data, product data, employee data, and more. By implementing MDM solutions, organizations can improve data quality, enhance decision-making, and achieve operational efficiency.
The Benefits of MDM
Implementing a robust MDM strategy brings numerous benefits to organizations:
- Improved Data Quality: MDM solutions enable organizations to cleanse and standardize their data, ensuring accuracy and consistency across systems. This leads to improved data quality and reliability.
- Enhanced Decision-Making: With a single, unified view of master data, organizations can make more informed decisions, as they have access to accurate and up-to-date information.
- Operational Efficiency: MDM streamlines business processes by eliminating duplicate and inconsistent data, reducing errors, and ensuring data integrity. This leads to improved operational efficiency and productivity.
- Regulatory Compliance: MDM helps organizations comply with data governance regulations by ensuring data accuracy, integrity, and security.
- Improved Customer Experience: By having a complete and accurate view of customer data, organizations can deliver personalized experiences, improve customer satisfaction, and drive customer loyalty.
MDM Implementation Process
The implementation process of MDM involves several key steps:
- Define Objectives: Clearly define the objectives and goals of the MDM initiative. Identify the master data domains that need to be managed and determine the scope of the implementation.
- Assess Data Quality: Evaluate the quality of existing data by conducting a data quality assessment. Identify data issues and establish data quality rules to be enforced by the MDM solution.
- Design Data Model: Design a data model that represents the master data entities and their relationships. Define data attributes, hierarchies, and data governance rules.
- Select MDM Solution: Choose an MDM platform or solution that aligns with the organization's requirements and objectives. Consider factors such as scalability, flexibility, integration capabilities, and vendor support.
- Data Integration: Integrate the MDM solution with existing systems and data sources. Ensure smooth data flow between systems to establish a single source of truth for master data.
- Data Governance: Establish data governance policies and processes to govern the creation, maintenance, and usage of master data. Define roles, responsibilities, and workflows for data stewardship and data governance activities.
- Data Migration: Migrate existing data into the MDM solution, ensuring data accuracy and integrity. Validate and cleanse data during the migration process.
- Deploy and Test: Deploy the MDM solution in a test environment and conduct thorough testing to ensure its functionality and performance. Address any issues or bugs identified during testing.
- Training and Change Management: Provide training to users and stakeholders on how to use the MDM solution effectively. Implement change management strategies to ensure smooth adoption of the MDM initiative.
- Go Live: Launch the MDM solution in a production environment. Monitor its performance, address any issues, and continuously improve the solution based on feedback and evolving business requirements.
Data Governance in MDM
Data governance is a critical component of MDM implementation. It refers to the overall management of data within an organization, including data policies, standards, processes, and controls. Data governance ensures that data is accurate, consistent, and secure throughout its lifecycle. In the context of MDM, data governance plays a crucial role in establishing and maintaining the integrity of master data.
Effective data governance in MDM requires:
- Data Governance Framework: Define a data governance framework that outlines the roles, responsibilities, and processes for managing master data. This framework should align with the organization's overall data governance strategy.
- Data Governance Policies: Develop data governance policies that govern the creation, maintenance, and usage of master data. These policies should establish data standards, data quality rules, and data security protocols.
- Data Stewardship: Appoint data stewards who are responsible for ensuring the quality, integrity, and security of master data. Data stewards collaborate with data owners and users to enforce data governance policies and resolve data-related issues.
- Data Governance Tools: Implement data governance tools and technologies that facilitate data governance activities, such as data profiling, data cleansing, data lineage, and metadata management.
- Continuous Monitoring and Improvement: Continuously monitor the quality and usage of master data. Implement data governance metrics and KPIs to measure the effectiveness of data governance efforts. Regularly review and update data governance policies and processes based on evolving business needs and regulatory requirements.
Challenges in MDM and Data Governance
Implementing MDM and establishing effective data governance can be challenging for organizations. Some common challenges include:
- Data Complexity: Organizations often deal with large volumes of complex data from various sources. Managing and integrating this data can be challenging.
- Data Quality: Poor data quality is a common issue that organizations face. Data inconsistencies, duplications, and inaccuracies can hinder the success of MDM and data governance initiatives.
- Organizational Alignment: MDM and data governance require strong organizational alignment and collaboration. Organizations may face challenges in getting buy-in from stakeholders and establishing clear roles and responsibilities.
- Change Management: Implementing MDM and data governance involves significant changes to existing processes and workflows. Organizations need to manage change effectively to ensure smooth adoption and minimize resistance.
- Technology Integration: Integrating MDM solutions with existing systems and technologies can be complex. Organizations need to ensure seamless data integration and interoperability.
- Data Security: Protecting master data from unauthorized access and ensuring data privacy are critical considerations in MDM and data governance.
Best Practices for MDM and Data Governance
To overcome the challenges and maximize the benefits of MDM and data governance, organizations should follow these best practices:
- Develop a Comprehensive MDM Strategy: Define a clear MDM strategy that aligns with the organization's goals and objectives. Consider the specific master data domains that need to be managed and the desired outcomes.
- Start with a Pilot Project: Begin with a small-scale pilot project to demonstrate the value and ROI of MDM and data governance initiatives. Use the pilot project to identify challenges, refine processes, and gain stakeholder buy-in.
- Establish Data Governance Policies: Develop data governance policies that address data quality, data security, data privacy, and data compliance. These policies should be aligned with industry standards and regulatory requirements.
- Involve Data Stewards: Appoint dedicated data stewards who have the knowledge and expertise to manage master data effectively. Data stewards should collaborate with data owners and users to enforce data governance policies and resolve data-related issues.
- Invest in Data Quality Management: Implement data quality management tools and processes to ensure data accuracy, consistency, and integrity. Regularly monitor and cleanse master data to maintain data quality.
- Ensure Data Integration: Establish seamless data integration between the MDM solution and existing systems. Enable data flow and synchronization to maintain a single source of truth for master data.
- Implement Metadata Management: Metadata management is crucial for understanding and managing master data. Implement metadata management tools and processes to capture and maintain metadata throughout the data lifecycle.
- Focus on Data Security: Implement robust data security measures to protect master data from unauthorized access, data breaches, and data leaks. Encrypt sensitive data, implement access controls, and regularly audit data security processes.
- Monitor and Measure: Continuously monitor the quality and usage of master data. Establish data governance metrics and KPIs to track the effectiveness of MDM and data governance initiatives. Regularly review and update processes based on feedback and evolving business needs.
Conclusion
Master Data Management (MDM) and effective data governance are essential for organizations to manage and leverage their master data assets. By implementing MDM solutions and establishing robust data governance practices, organizations can improve data quality, enhance decision-making, and achieve operational efficiency. However, implementing MDM and data governance comes with its own set of challenges. Organizations should follow best practices, involve data stewards, invest in data quality management, ensure data integration, and focus on data security to overcome these challenges and maximize the benefits of MDM and data governance.
Contact us
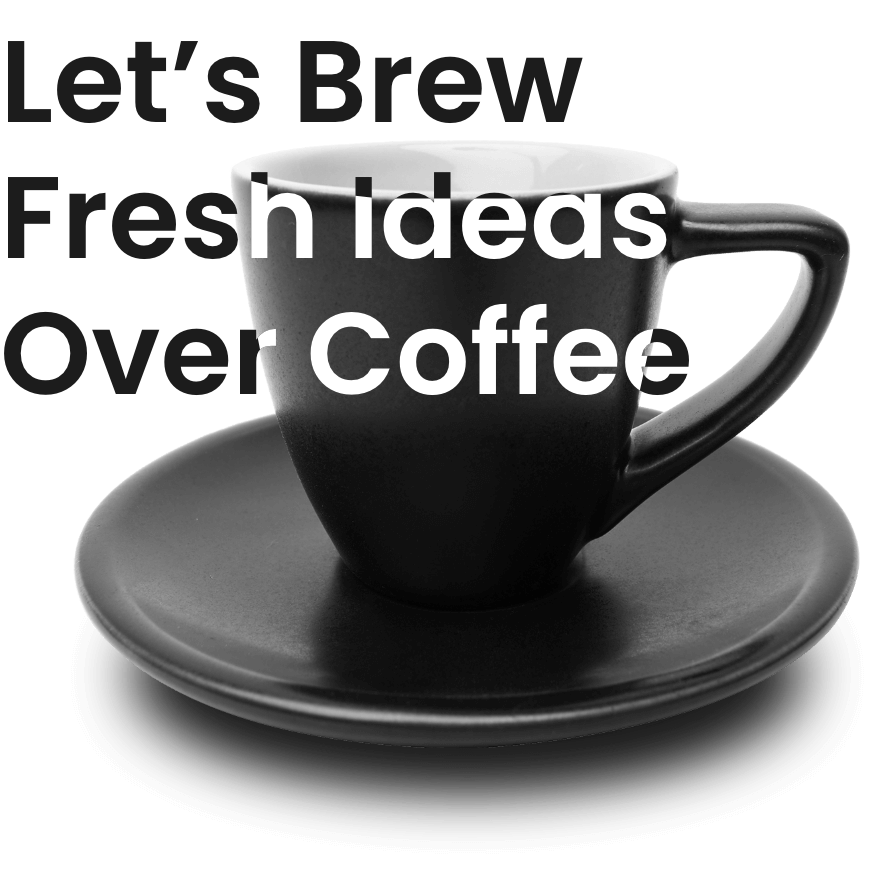
Spanning 8 cities worldwide and with partners in 100 more, we’re your local yet global agency.
Fancy a coffee, virtual or physical? It’s on us – let’s connect!