The impact of MDM implementation on data quality management processes
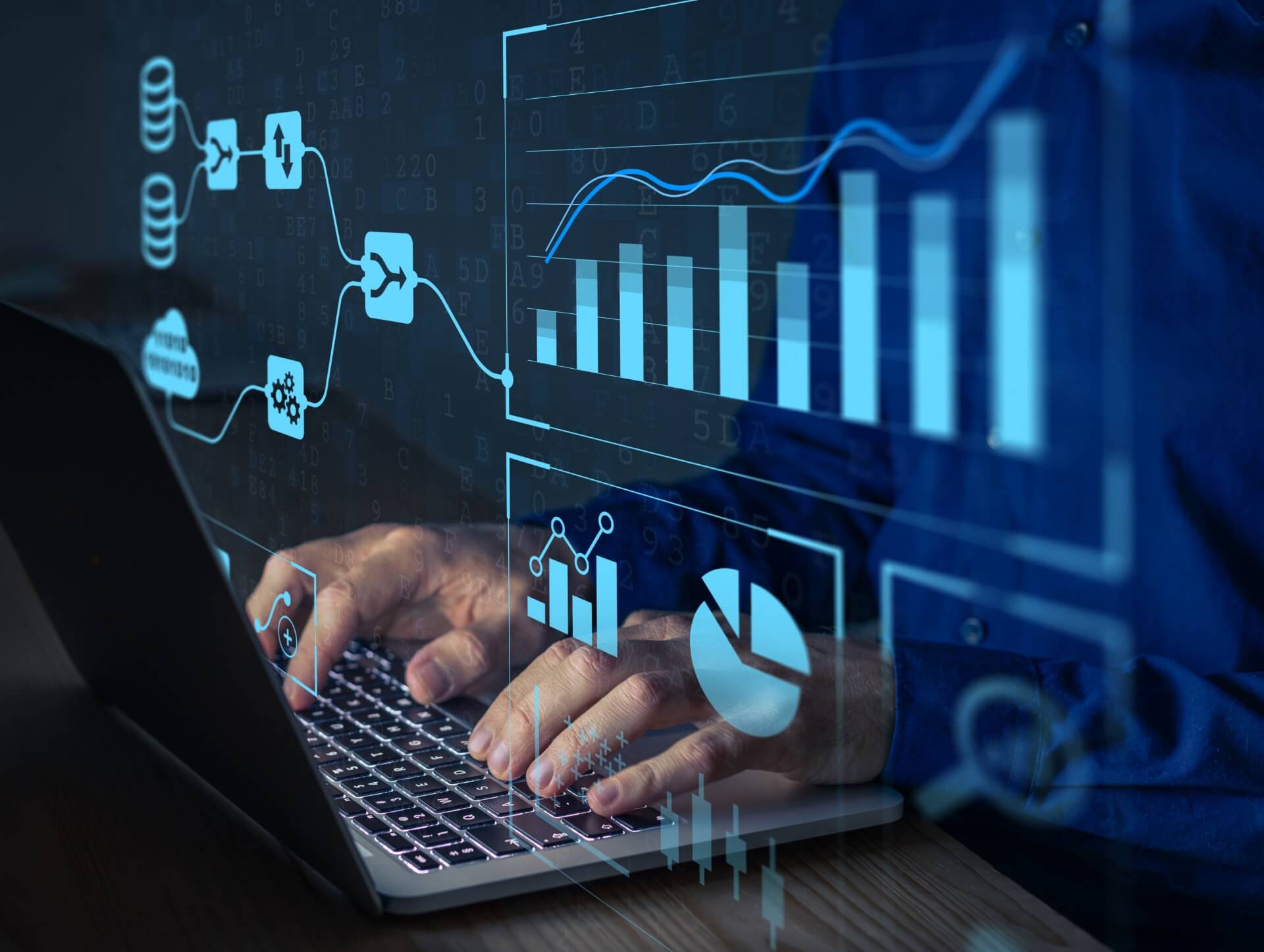
06/09/2023
Master Data Management (MDM) is a comprehensive approach to managing and organizing an organization's critical data assets. It involves establishing a single, authoritative source of data to ensure consistency, accuracy, and reliability across all applications and systems. MDM implementation plays a crucial role in improving data quality management processes, ensuring that businesses can make informed decisions based on reliable and consistent data.
Benefits of MDM in Data Quality Management
Implementing MDM solutions brings numerous benefits to data quality management processes:
- Improved Data Accuracy: MDM ensures that data is accurate, complete, and up-to-date by consolidating data from various sources and resolving any inconsistencies or duplicates.
- Enhanced Data Consistency: MDM establishes data governance policies and procedures to ensure consistent data definitions, standards, and formats across the organization.
- Increased Data Integrity: MDM provides mechanisms for data validation, verification, and cleansing, ensuring that data quality is maintained throughout its lifecycle.
- Streamlined Data Integration: MDM simplifies the process of integrating data from disparate sources, enabling organizations to have a unified view of their data and avoid data silos.
- Better Decision-Making: With accurate and reliable data, organizations can make more informed decisions, leading to improved business outcomes and competitive advantage.
MDM Implementation Process
The implementation process of MDM involves several stages:
- Assessment and Planning: This stage involves assessing the organization's current data management practices, identifying data quality issues, and defining the goals and objectives of the MDM implementation.
- Strategy Development: In this stage, organizations develop a comprehensive MDM strategy that outlines the approach, scope, and timeline of the implementation. This includes selecting the right MDM solution, defining data governance policies, and establishing data quality metrics.
- Data Profiling and Cleansing: Organizations analyze their data to identify any data quality issues, such as duplicates, inconsistencies, or inaccuracies. Data cleansing techniques are then applied to resolve these issues and improve data quality.
- Data Integration: This stage involves integrating data from various sources into the MDM platform. Data integration ensures that a unified view of data is maintained across the organization.
- Data Governance: Establishing data governance processes and policies is crucial for maintaining data quality. This involves defining data ownership, roles, and responsibilities, as well as implementing data governance tools and technologies.
- Continuous Monitoring and Improvement: Once the MDM implementation is complete, organizations need to continuously monitor and measure data quality, identify any new data issues, and make improvements to the MDM solution and processes as necessary.
Data Governance in MDM
Data governance is an essential component of MDM, as it ensures that data is managed effectively throughout its lifecycle. It involves defining data ownership, accountability, and stewardship, as well as establishing policies, procedures, and controls to ensure data quality and compliance. Data governance in MDM includes the following best practices:
- Clearly define data ownership and assign responsibilities to individuals or departments within the organization.
- Establish data governance policies and procedures that define data quality standards, data classification, and data access controls.
- Implement data governance tools and technologies that enable data profiling, data cleansing, and data monitoring.
- Regularly audit and review data governance processes to ensure compliance with regulatory requirements and industry standards.
- Provide training and education to employees to raise awareness about the importance of data governance and data quality management.
MDM and Data Security
Data security is a critical aspect of MDM implementation. Organizations need to ensure that their data is protected from unauthorized access, data breaches, and other security threats. Some key considerations for data security in MDM include:
- Implementing access controls and user authentication mechanisms to restrict access to sensitive data.
- Encrypting data at rest and in transit to protect it from unauthorized disclosure.
- Regularly monitoring and auditing data access and usage to detect any suspicious activities.
- Implementing data masking and anonymization techniques to protect sensitive data during testing and development processes.
- Establishing data security policies and procedures that define data security requirements and controls.
MDM and Data Analytics
MDM plays a crucial role in enabling effective data analytics. By ensuring data quality and consistency, organizations can rely on accurate and reliable data for their analytics initiatives. MDM enables the following benefits in data analytics:
- Unified and Consistent Data: MDM provides a single, unified view of data, ensuring consistency in data definitions, formats, and standards. This enables organizations to perform more accurate and reliable analytics.
- Improved Data Quality: MDM ensures that data is accurate, complete, and up-to-date, improving the quality of data used for analytics. This leads to more reliable and meaningful insights.
- Efficient Data Integration: MDM simplifies the process of integrating data from various sources, enabling organizations to have a comprehensive view of their data. This facilitates more comprehensive and holistic analytics.
- Better Decision-Making: With accurate and reliable data, organizations can make more informed decisions based on data-driven insights, leading to improved business outcomes.
Challenges in MDM Implementation
While MDM implementation brings significant benefits to data quality management processes, it also comes with its own set of challenges:
- Data Complexity: Organizations often have large volumes of complex and diverse data, making it challenging to consolidate and manage effectively.
- Data Integration: Integrating data from disparate sources can be complex and time-consuming, requiring careful planning and coordination.
- Data Governance: Establishing effective data governance processes and policies can be challenging, as it requires collaboration and alignment across different departments and stakeholders.
- Change Management: MDM implementation often requires significant changes to existing processes and systems, which can be met with resistance from employees.
- Cost and Resources: Implementing and maintaining an MDM solution can be costly, requiring investments in technology, resources, and training.
Conclusion
MDM implementation has a significant impact on data quality management processes. It enables organizations to improve data accuracy, consistency, integrity, and integration, leading to better decision-making and improved business outcomes. However, MDM implementation also comes with its own set of challenges, which need to be carefully addressed to ensure successful implementation and adoption. By following best practices, organizations can harness the power of MDM to transform their data quality management processes and gain a competitive edge in the market.
Contact us
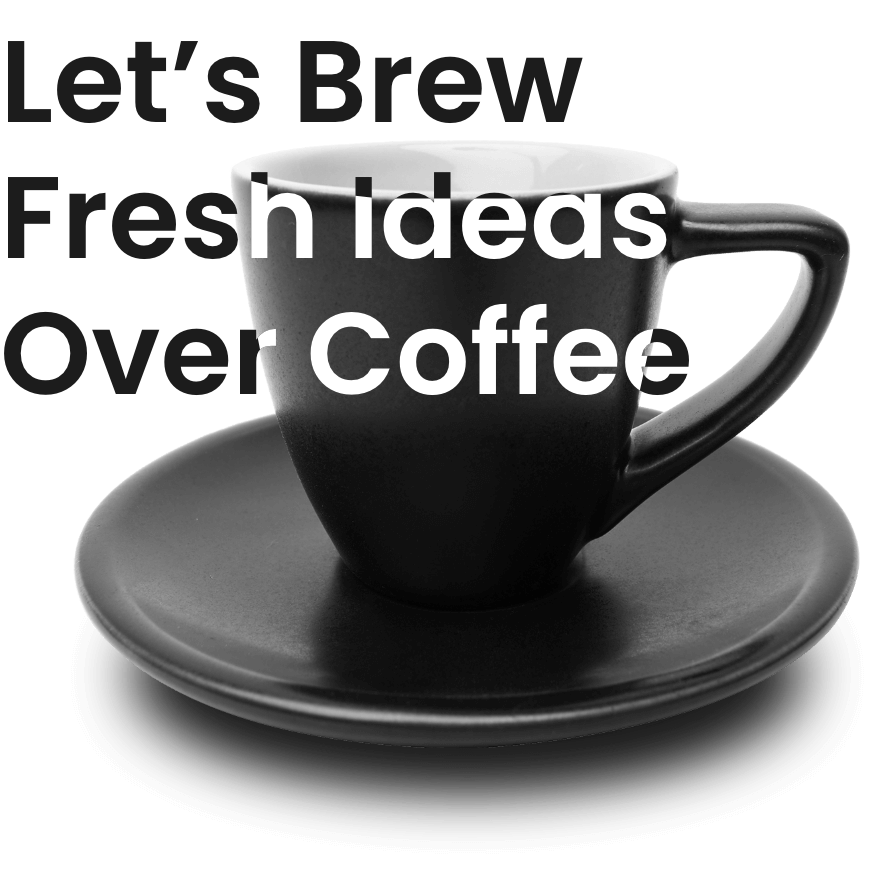
Spanning 8 cities worldwide and with partners in 100 more, we’re your local yet global agency.
Fancy a coffee, virtual or physical? It’s on us – let’s connect!