The impact of MDM on data governance and data stewardship in enterprise scalability
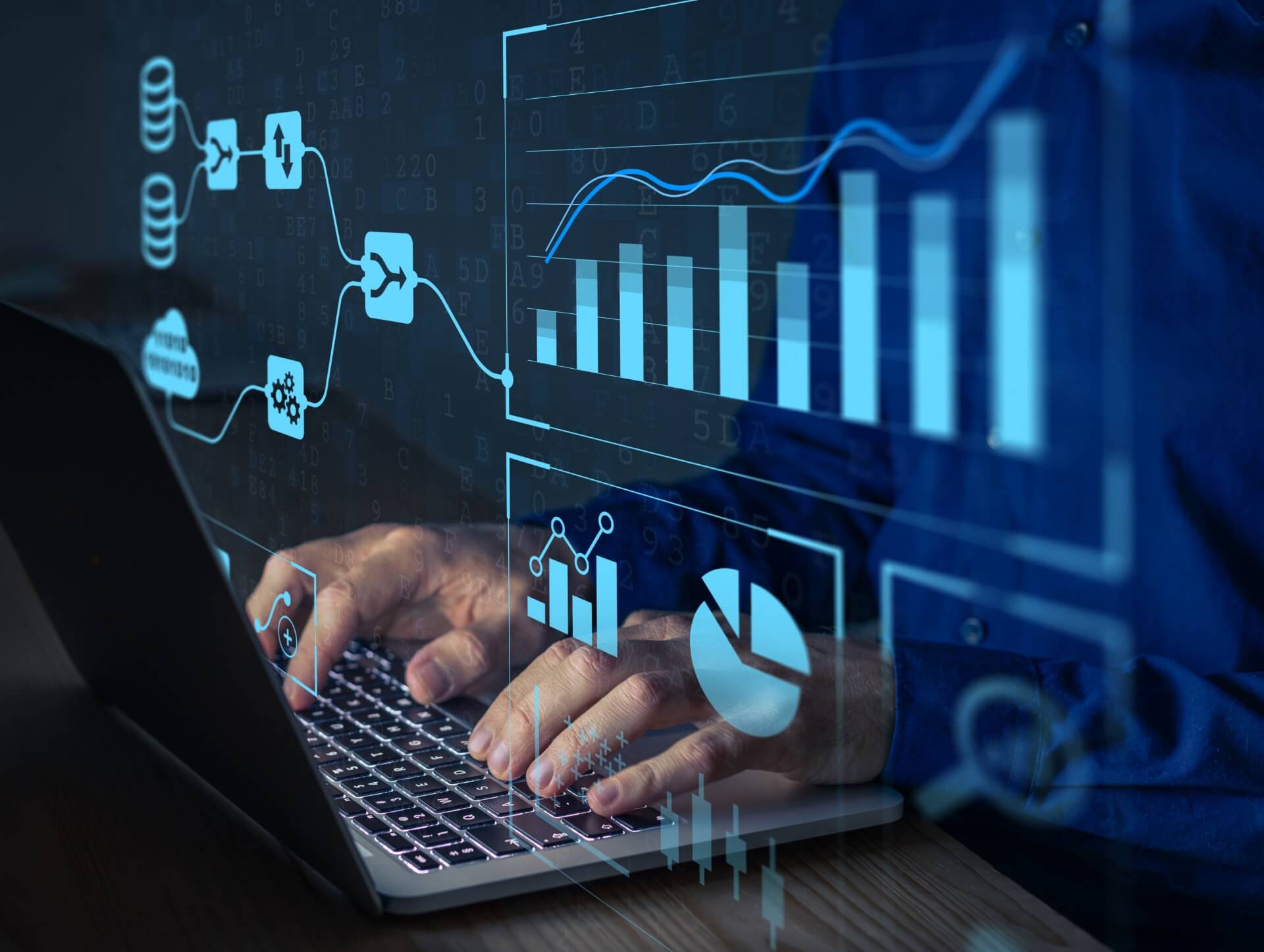
06/09/2023
Master Data Management (MDM) has emerged as a critical component in the efficient and effective management of data within organizations. With the exponential growth of data, businesses are facing challenges in maintaining data quality, consistency, and accuracy. MDM provides a comprehensive solution to these challenges by establishing a single source of truth for master data, ensuring data governance, and enabling data stewardship. In this article, we will explore the impact of MDM on data governance and data stewardship in enterprise scalability.
The Importance of Data Governance in MDM
Data governance plays a crucial role in MDM. It encompasses the processes, policies, and guidelines for managing data assets within an organization. Data governance ensures that data is accurate, consistent, and reliable, which is essential for making informed business decisions. MDM provides a framework for data governance by establishing a centralized and standardized approach to data management. It enables organizations to define data ownership, data quality standards, and data access controls. By implementing MDM, organizations can improve data governance and ensure data integrity throughout the enterprise.
MDM Best Practices for Data Governance and Data Stewardship
Implementing MDM requires adherence to best practices to maximize its effectiveness. Here are some best practices for data governance and data stewardship in the context of MDM:
1. Define Clear Data Governance Roles and Responsibilities
Assigning clear roles and responsibilities is crucial for effective data governance and data stewardship. Define roles such as data stewards, data owners, and data custodians, and clearly outline their responsibilities. Data stewards are responsible for data quality, while data owners are accountable for the accuracy and completeness of the data. Data custodians are responsible for data storage, security, and access controls. By clearly defining these roles, organizations can ensure accountability and streamline data governance processes.
2. Establish Data Quality Management Processes
Data quality is essential for reliable decision-making. Implement data quality management processes to ensure that data is accurate, complete, and consistent. This includes data profiling, data cleansing, and data validation. MDM provides tools and technologies to automate these processes and improve data quality. By continuously monitoring data quality and addressing any issues, organizations can maintain high-quality data and ensure its integrity in MDM.
3. Implement Data Integration in MDM
Data integration is a critical aspect of MDM. It involves integrating data from various sources and systems into a single master data repository. This ensures data consistency and eliminates data silos. MDM platforms provide data integration capabilities, allowing organizations to harmonize and consolidate data from multiple sources. By implementing data integration in MDM, organizations can achieve a unified view of their data and enhance data governance.
4. Ensure Data Security and Privacy
Data security and privacy are paramount in MDM. Organizations must ensure that data is protected from unauthorized access, breaches, and data loss. Implement robust security measures, such as access controls, encryption, and data masking. Compliance with data privacy regulations, such as GDPR and CCPA, is also essential. By prioritizing data security and privacy in MDM, organizations can build trust and confidence among their customers and stakeholders.
The Role of Data Stewardship in MDM
Data stewardship is the process of managing and overseeing data assets within an organization. It involves defining data standards, ensuring data quality, and resolving data-related issues. MDM enables effective data stewardship by providing a centralized platform for data management and governance. It allows data stewards to have a holistic view of data across the organization and ensures that data is accurate, complete, and consistent. Data stewards can collaborate with other stakeholders and make informed decisions based on reliable data. MDM also enables data stewards to define data policies, enforce data governance rules, and monitor data quality. By leveraging MDM for data stewardship, organizations can improve data management practices and drive better business outcomes.
MDM and Enterprise Scalability
Enterprise scalability refers to the ability of an organization to handle the increasing volume, variety, and velocity of data. MDM plays a vital role in enabling enterprise scalability by providing a scalable and flexible platform for data management. Here's how MDM contributes to enterprise scalability:
1. Centralized and Standardized Data Management
MDM establishes a centralized and standardized approach to data management. It consolidates data from various sources into a single master data repository, ensuring data consistency and eliminating data silos. This centralized approach enables organizations to scale their data management capabilities without the need for multiple systems and databases. By standardizing data management processes, organizations can easily accommodate growing data volumes and ensure scalability.
2. Streamlined Data Governance Processes
Effective data governance is essential for managing growing data volumes. MDM provides a framework for data governance by defining data ownership, data quality standards, and data access controls. This streamlines data governance processes and ensures that data is managed consistently across the organization. By implementing MDM, organizations can efficiently manage large volumes of data and navigate the complexities of data governance.
3. Enhanced Data Quality and Accuracy
As data volumes increase, maintaining data quality becomes challenging. MDM enables organizations to improve data quality by implementing data quality management processes. These processes include data profiling, data cleansing, and data validation. By ensuring high-quality data, organizations can make reliable business decisions and achieve better outcomes. MDM also provides tools and technologies for data quality monitoring and remediation, further enhancing data accuracy and scalability.
4. Agile Data Integration
Data integration is a critical aspect of managing large volumes of data. MDM platforms provide data integration capabilities, allowing organizations to integrate data from various sources into a single master data repository. This agile data integration enables organizations to scale their data management capabilities and accommodate new data sources and systems. By leveraging MDM for data integration, organizations can achieve scalability and agility in managing data.
Challenges in MDM and Data Governance
While MDM and data governance offer significant benefits, organizations may face challenges in their implementation. Here are some common challenges in MDM and data governance:
1. Data Quality Management
Ensuring data quality is a persistent challenge in MDM and data governance. Organizations may struggle with data cleansing, data validation, and data standardization processes. Data quality issues can impact the reliability of the master data and hinder data-driven decision-making. Implementing robust data quality management processes and leveraging MDM tools can help address these challenges.
2. Data Integration Complexity
Data integration can be complex, especially when dealing with data from multiple sources and systems. Organizations may face challenges in mapping data attributes, resolving data conflicts, and maintaining data consistency. MDM platforms provide data integration capabilities that simplify the data integration process. However, organizations need to invest in data integration expertise and ensure proper data mapping and transformation.
3. Data Governance Framework
Establishing a robust data governance framework can be challenging, especially in large organizations. Organizations may struggle with defining clear roles and responsibilities, enforcing data governance rules, and ensuring data compliance. It is essential to have strong executive sponsorship, effective communication, and continuous monitoring to overcome these challenges.
4. Data Security and Privacy
Data security and privacy are critical considerations in MDM and data governance. Organizations need to implement robust security measures, ensure compliance with data privacy regulations, and protect data from unauthorized access. Data breaches and non-compliance can lead to reputational damage and legal consequences. Investing in data security technologies and regularly assessing security measures can help mitigate these challenges.
Case Studies: Successful MDM Implementations
Several organizations have successfully implemented MDM and achieved significant benefits. Here are a few case studies:
1. Company A: Streamlining Customer Data Management
Company A, a global retail company, implemented an MDM solution to streamline customer data management. They had customer data scattered across multiple systems, resulting in data inconsistencies and duplicate records. By implementing MDM, they achieved a single view of customer data, eliminated duplicate records, and improved data accuracy. This enabled them to personalize customer experiences, improve customer satisfaction, and drive revenue growth.
2. Company B: Enhancing Product Data Governance
Company B, a manufacturing company, faced challenges in managing product data across multiple business units. They implemented MDM to establish a centralized product data repository and standardize product data management processes. By improving product data governance, they achieved better data quality, reduced time-to-market for new products, and increased operational efficiency. MDM also enabled them to comply with industry regulations and improve data security.
3. Company C: Optimizing Supply Chain Management
Company C, a logistics company, implemented MDM to optimize their supply chain management processes. They had data scattered across multiple systems, making it challenging to track inventory, manage suppliers, and forecast demand. By implementing MDM, they achieved a centralized and standardized view of supply chain data. This enabled them to improve inventory management, reduce stockouts, and enhance supplier collaboration. MDM also provided real-time visibility into supply chain metrics, enabling data-driven decision-making.
The Future of MDM and Data Governance
MDM and data governance will continue to evolve in response to the changing data landscape. Here are some trends that will shape the future of MDM and data governance:
1. Integration with Data Analytics and AI
MDM will increasingly integrate with data analytics and artificial intelligence (AI) technologies. This integration will enable organizations to leverage the power of data analytics and AI for data-driven decision-making. MDM platforms will provide advanced analytics capabilities, allowing organizations to gain insights from master data and make proactive business decisions.
2. Cloud-based MDM Solutions
Cloud-based MDM solutions will gain traction as organizations embrace cloud computing. Cloud-based MDM offers scalability, flexibility, and cost-efficiency. It allows organizations to leverage the benefits of cloud computing while ensuring data governance and data stewardship. Cloud-based MDM also enables easy integration with other cloud-based applications and systems.
3. Data Governance in Multi-Cloud Environments
As organizations adopt multi-cloud environments, data governance will become more complex. Data governance frameworks will need to address the challenges of managing data across multiple clouds, ensuring data consistency and security. MDM platforms will provide solutions for data governance in multi-cloud environments, enabling organizations to maintain control over their data.
4. Automation and AI in Data Governance
Data governance processes will increasingly be automated using AI technologies. AI-powered data governance solutions will streamline data stewardship, data quality management, and data compliance processes. Automation will enable organizations to manage growing data volumes efficiently and ensure data governance throughout the data lifecycle.
Conclusion
MDM has a significant impact on data governance and data stewardship in enterprise scalability. By implementing MDM, organizations can establish a single source of truth for master data, ensure data governance, and enable data stewardship. MDM provides a framework for data governance and enables organizations to define clear roles and responsibilities, implement data quality management processes, integrate data, and ensure data security and privacy. MDM plays a vital role in enabling enterprise scalability by providing a centralized and standardized approach to data management, streamlining data governance processes, enhancing data quality and accuracy, and enabling agile data integration. While challenges exist in implementing MDM and data governance, organizations can overcome them by following best practices and leveraging MDM tools and technologies. The future of MDM and data governance will be shaped by trends such as integration with data analytics and AI, cloud-based MDM solutions, data governance in multi-cloud environments, and automation in data governance.
Contact us
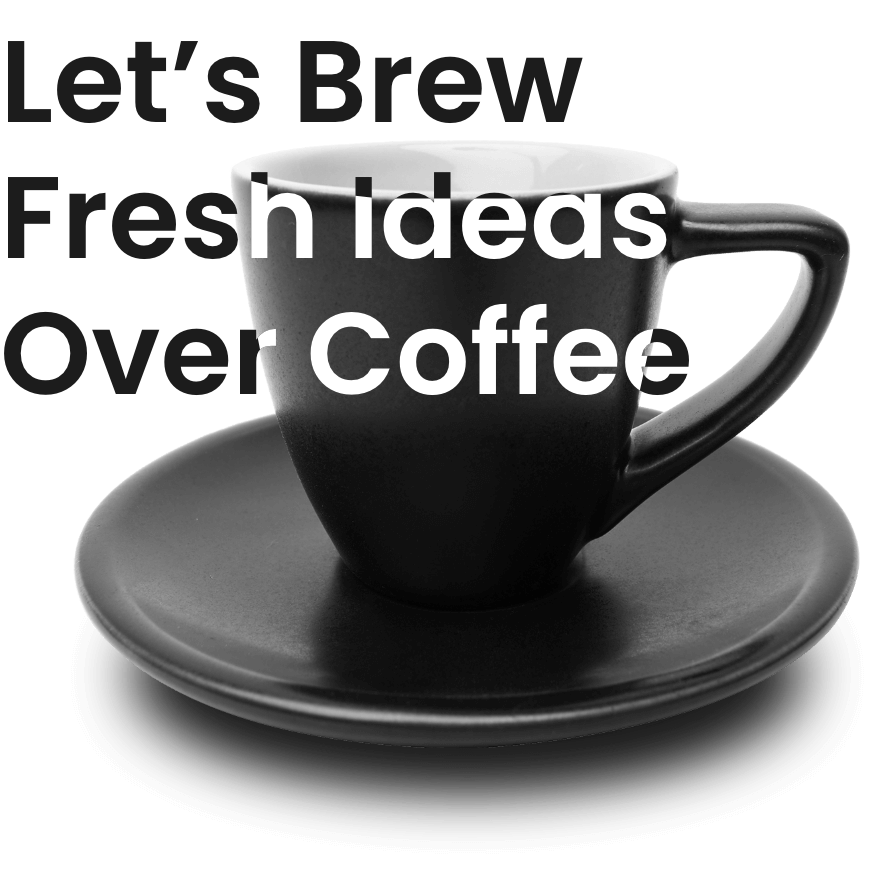
Spanning 8 cities worldwide and with partners in 100 more, we’re your local yet global agency.
Fancy a coffee, virtual or physical? It’s on us – let’s connect!