The impact of MDM on data lineage and data traceability in enterprise scalability
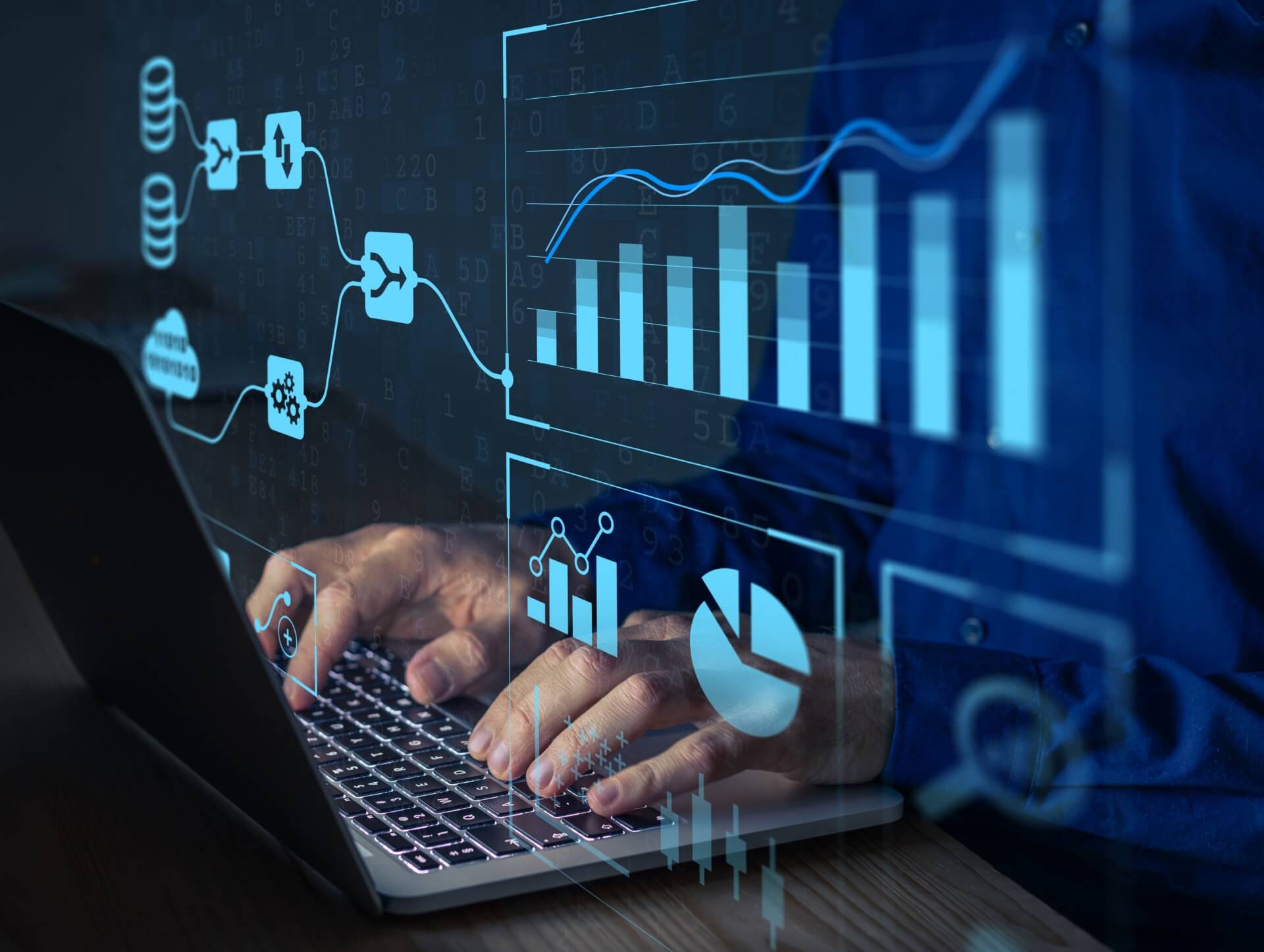
06/09/2023
Master Data Management (MDM) is a critical component in today's data-driven enterprises. It provides a comprehensive approach to managing and governing master data, which is the core data that is essential for the smooth functioning of business operations. MDM solutions offer a centralized platform for managing and maintaining data consistency, accuracy, and integrity across various systems and applications.
MDM strategy involves implementing processes, technologies, and policies to ensure that master data is accurate, up-to-date, and accessible to all relevant stakeholders. It helps organizations achieve a single version of truth and enables effective decision-making and analytics.
Benefits of Master Data Management
Implementing a robust MDM platform brings several benefits to organizations:
1. Improved data quality: MDM ensures that master data is accurate, complete, and consistent. It reduces data duplication, errors, and inconsistencies, leading to improved data quality and reliability.
2. Enhanced data governance: MDM enables organizations to establish data governance policies and procedures to ensure data integrity, security, and compliance. It provides a framework for data stewardship, data ownership, and data lifecycle management.
3. Increased operational efficiency: By centralizing and standardizing master data, MDM streamlines business processes and eliminates redundant data. It improves data accessibility, reduces data entry efforts, and enhances operational efficiency.
4. Better decision-making: MDM provides a single, reliable source of master data, enabling organizations to make informed and accurate decisions. It supports data-driven insights, analytics, and reporting, leading to better business outcomes.
5. Improved customer experience: MDM enables organizations to have a unified view of customers across multiple touchpoints. It helps in personalizing customer interactions, delivering consistent experiences, and improving customer satisfaction.
Data Governance in MDM
Data governance plays a crucial role in the success of MDM initiatives. It involves defining and implementing policies, processes, and controls to ensure the effective management and use of data. In the context of MDM, data governance focuses on managing master data throughout its lifecycle.
Key components of data governance in MDM include:
1. Data stewardship: Data stewards are responsible for ensuring the quality, integrity, and accuracy of master data. They define data standards, resolve data issues, and enforce data governance policies.
2. Data ownership: Data owners are accountable for the overall management and governance of master data. They define data access rights, approve data changes, and ensure compliance with data governance policies.
3. Data lifecycle management: MDM involves managing master data throughout its lifecycle, from creation to retirement. Data governance ensures that appropriate processes are in place for data creation, validation, integration, and archival.
4. Data compliance: MDM initiatives need to comply with relevant data regulations and industry standards. Data governance ensures that data privacy, security, and compliance requirements are met.
MDM Best Practices
Implementing MDM successfully requires following a set of best practices:
1. Clearly define goals and objectives: It is essential to have a clear understanding of the desired outcomes and benefits of MDM. Define specific goals and objectives that align with the organization's overall strategy.
2. Establish data governance framework: Develop a comprehensive data governance framework that defines roles, responsibilities, and processes for managing master data. Ensure buy-in from stakeholders and establish a governance council.
3. Conduct data quality assessment: Before implementing MDM, assess the quality of existing data. Identify data issues, data gaps, and data sources. Develop a data quality improvement plan and prioritize data cleansing and enrichment activities.
4. Plan data integration and migration: MDM involves integrating and migrating data from various source systems. Develop a data integration strategy and plan for data extraction, transformation, and loading. Test data integration processes and ensure data accuracy and consistency.
5. Engage business and IT stakeholders: MDM is a collaborative effort between business and IT teams. Involve key stakeholders from both sides in the MDM implementation process. Understand their requirements and ensure their active participation.
6. Invest in data governance tools and technologies: Choose the right MDM tools and technologies that align with your organization's requirements. Consider factors such as scalability, flexibility, ease of use, and integration capabilities.
7. Define data quality metrics and KPIs: Establish data quality metrics and key performance indicators (KPIs) to measure the effectiveness of MDM. Monitor data quality continuously and take corrective actions as required.
8. Provide training and support: MDM requires a shift in mindset and cultural change. Provide training and support to users and stakeholders to ensure smooth adoption of the MDM platform. Communicate the benefits and value of MDM to gain buy-in and support from all levels of the organization.
MDM and Data Security
Data security is a critical aspect of MDM. Organizations need to ensure that master data is protected from unauthorized access, manipulation, and breaches. MDM platforms incorporate various security measures to safeguard data:
1. Access controls: MDM platforms provide role-based access controls to restrict access to master data. Only authorized users with the necessary permissions can view, edit, or delete master data.
2. Data encryption: MDM platforms use encryption techniques to protect master data at rest and in transit. Encryption ensures that even if data is compromised, it remains unreadable without the decryption key.
3. Audit trails: MDM platforms maintain detailed audit trails of all activities related to master data. This includes data changes, data access, and data approvals. Audit trails help in tracking and investigating any unauthorized or suspicious activities.
4. Data masking: MDM platforms support data masking techniques to protect sensitive data. Data masking replaces sensitive data with realistic but fictitious data, ensuring that the original data cannot be identified.
5. Data backup and recovery: MDM platforms include mechanisms for regular data backup and recovery. This ensures that in case of data loss or system failure, organizations can restore master data to a previous state.
MDM and Data Analytics
MDM and data analytics go hand in hand. MDM provides the foundation for accurate and reliable data, which is essential for meaningful analytics and insights. By implementing MDM, organizations can:
1. Establish a single source of truth: MDM ensures that master data is consistent and accurate across various systems and applications. This provides a reliable source of data for analytics, eliminating data discrepancies and inconsistencies.
2. Improve data quality: MDM helps in improving data quality by eliminating duplicates, errors, and inconsistencies. Clean and reliable data is essential for accurate analytics and meaningful insights.
3. Enable data integration: MDM integrates data from various sources, enabling organizations to analyze data from different systems and applications. This allows for a comprehensive view of data and enables cross-functional and cross-system analytics.
4. Support advanced analytics: MDM provides the foundation for advanced analytics techniques such as data mining, predictive analytics, and machine learning. These techniques enable organizations to uncover patterns, trends, and insights from their data.
5. Facilitate self-service analytics: MDM platforms can provide self-service analytics capabilities, allowing business users to access and analyze master data without IT intervention. This empowers business users and reduces the dependency on IT teams for data analysis.
MDM and Customer Experience
MDM plays a crucial role in enhancing the customer experience. By providing a unified and consistent view of customer data, MDM enables organizations to:
1. Personalize customer interactions: MDM helps in creating a comprehensive customer profile by consolidating data from various touchpoints. This enables organizations to personalize customer interactions, offer relevant products and services, and deliver a superior customer experience.
2. Provide omni-channel experience: MDM ensures that customer data is consistent and up-to-date across all channels and touchpoints. This allows customers to seamlessly switch between channels and receive the same level of service and experience.
3. Enable accurate customer segmentation: MDM provides accurate and reliable customer data, enabling organizations to segment customers based on various attributes such as demographics, behavior, and preferences. This helps in targeting the right customers with the right offers and messages.
4. Improve customer service: MDM enables organizations to have a 360-degree view of customers, including their purchase history, interactions, and preferences. This enables customer service representatives to provide personalized and efficient service, leading to higher customer satisfaction.
5. Enhance customer loyalty: By delivering a consistent and personalized customer experience, MDM helps in building customer loyalty. Satisfied and loyal customers are more likely to repeat purchases, recommend the brand to others, and become brand advocates.
Challenges in MDM
Implementing and managing MDM comes with its own set of challenges:
1. Data complexity: Organizations deal with vast amounts of data from various sources and systems. Managing and integrating this data into a centralized MDM platform can be complex and challenging.
2. Data quality issues: Poor data quality is a common challenge in MDM. Data duplication, errors, and inconsistencies can affect the accuracy and reliability of master data.
3. Data governance: Establishing and maintaining effective data governance processes and policies can be challenging. Organizations need to ensure that data governance is an ongoing effort and not a one-time activity.
4. Organizational change: Implementing MDM requires a cultural shift and change in mindset. It may face resistance from employees who are accustomed to working in silos or have limited understanding of the value of MDM.
5. Integration with legacy systems: Integrating MDM with existing legacy systems can be challenging, especially if the systems are outdated or lack standardization. Organizations may need to invest in data integration tools and technologies.
6. Scalability: As organizations grow and expand, their data volumes and complexity increase. MDM platforms need to be scalable to handle large volumes of data and support future growth.
MDM Case Studies
Several organizations have successfully implemented MDM and realized significant benefits:
1. Company A: Company A, a global retail company, implemented MDM to address data quality issues and improve customer experience. By centralizing customer data and implementing data governance processes, they were able to provide a personalized and seamless customer experience across all channels. This resulted in increased customer satisfaction and loyalty.
2. Company B: Company B, a healthcare organization, implemented MDM to consolidate and manage patient data across multiple systems and locations. By implementing data governance policies and standardizing data processes, they were able to improve data accuracy, reduce duplication, and ensure compliance with data regulations. This resulted in improved patient care and operational efficiency.
3. Company C: Company C, a financial services company, implemented MDM to achieve a single view of customer data and improve cross-selling and upselling opportunities. By integrating customer data from various systems and applications, they were able to identify customer preferences, segment customers accurately, and target them with personalized offers. This resulted in increased revenue and customer satisfaction.
MDM in Multi-Cloud Environments
With the increasing adoption of cloud computing, organizations are deploying MDM platforms in multi-cloud environments. Multi-cloud environments offer several benefits, including:
1. Flexibility and scalability: Multi-cloud environments allow organizations to choose the best cloud services for their specific requirements. It provides flexibility to scale resources up or down based on demand.
2. Redundancy and resilience: By distributing MDM services across multiple cloud providers, organizations can achieve redundancy and resilience. In case of a cloud outage or failure, MDM services can continue to operate from other cloud providers.
3. Cost optimization: Multi-cloud environments enable organizations to leverage the cost advantages and pricing models of different cloud providers. It allows organizations to optimize costs by choosing the most cost-effective cloud services for MDM.
4. Vendor lock-in avoidance: By adopting a multi-cloud strategy, organizations can avoid vendor lock-in. It provides the flexibility to switch between cloud providers or use multiple cloud providers simultaneously.
MDM ROI and Value
Implementing MDM can deliver significant Return on Investment (ROI) and value to organizations:
1. Improved operational efficiency: MDM streamlines business processes, reduces data duplication, and eliminates redundant data. This leads to improved operational efficiency and cost savings.
2. Enhanced decision-making: MDM provides reliable and accurate master data, enabling organizations to make informed and data-driven decisions. This leads to better business outcomes and competitive advantage.
3. Increased customer satisfaction: MDM enables organizations to provide personalized and consistent customer experiences. This leads to increased customer satisfaction, loyalty, and repeat business.
4. Regulatory compliance: MDM helps organizations comply with data regulations and industry standards. This reduces the risk of non-compliance, penalties, and reputational damage.
5. Competitive advantage: By implementing MDM, organizations can gain a competitive advantage by leveraging accurate and reliable master data. It enables organizations to innovate, enter new markets, and differentiate themselves from competitors.
Data Governance in Lifecycle Management
Data governance is an essential component of data lifecycle management. It ensures that data is managed throughout its lifecycle, from creation to retirement. Key aspects of data governance in lifecycle management include:
1. Metadata management: Metadata provides context and information about data, such as its origin, structure, and meaning. Metadata management ensures that metadata is captured, stored, and maintained throughout the data lifecycle.
2. Data compliance: Data compliance involves ensuring that data is compliant with relevant regulations, policies, and standards. Data governance ensures that appropriate controls and processes are in place to achieve data compliance.
3. Data security: Data security is crucial in the data lifecycle. Data governance ensures that data is protected from unauthorized access, manipulation, and breaches throughout its lifecycle.
4. Automation: Data governance in lifecycle management can be enhanced through automation. Automation tools and technologies can streamline data governance processes, improve efficiency, and reduce manual efforts.
Challenges in Data Lifecycle Management
Data lifecycle management comes with its own set of challenges:
1. Data volume and complexity: Organizations deal with vast amounts of data from various sources and systems. Managing and processing this data throughout its lifecycle can be challenging.
2. Data quality issues: Poor data quality is a common challenge in data lifecycle management. Data duplication, errors, and inconsistencies can affect the accuracy and reliability of data.
3. Data storage and retention: Organizations need to store and retain data for specific periods based on legal, regulatory, and business requirements. Managing data storage and retention can be complex and costly.
4. Data privacy and security: Data privacy and security are critical aspects of data lifecycle management. Organizations need to ensure that data is protected from unauthorized access, manipulation, and breaches.
5. Compliance with regulations: Data lifecycle management needs to comply with various data regulations, industry standards, and internal policies. Organizations need to stay updated with regulatory requirements and ensure compliance.
Best Practices for Lifecycle Management
Implementing effective data lifecycle management requires following best practices:
1. Define data lifecycle stages: Clearly define the stages of the data lifecycle, including data creation, validation, integration, storage, retention, and retirement. Develop processes and policies for each stage.
2. Establish data governance: Data governance is critical in data lifecycle management. Develop a comprehensive data governance framework that defines roles, responsibilities, and processes for managing data throughout its lifecycle.
3. Implement data quality management: Data quality management is essential for accurate and reliable data throughout its lifecycle. Implement data cleansing, data validation, and data enrichment processes to improve data quality.
4. Invest in data lifecycle management tools and technologies: Choose the right tools and technologies for data lifecycle management. Consider factors such as scalability, flexibility, ease of use, and integration capabilities.
5. Automate data lifecycle processes: Automation can streamline data lifecycle processes and improve efficiency. Implement automation tools and technologies to automate data integration, data validation, and data retention processes.
6. Monitor and measure data lifecycle performance: Establish metrics and KPIs to measure the effectiveness of data lifecycle management. Continuously monitor data quality, data storage, and data retention processes and take corrective actions as required.
ROI of Effective Data Lifecycle
Implementing effective data lifecycle management can deliver significant ROI to organizations:
1. Cost savings: Effective data lifecycle management reduces storage costs by optimizing data storage and retention. It also reduces data duplication and data cleaning efforts, leading to cost savings.
2. Improved data quality: Data lifecycle management ensures that data is accurate, complete, and reliable throughout its lifecycle. Improved data quality leads to better decision-making, reduced errors, and improved operational efficiency.
3. Increased productivity: By streamlining and automating data lifecycle processes, organizations can improve productivity. Employees spend less time on manual data management tasks and more time on value-added activities.
4. Enhanced data security and compliance: Effective data lifecycle management ensures that data is protected from unauthorized access, manipulation, and breaches. It also helps organizations comply with data regulations and industry standards.
5. Improved data accessibility and availability: Data lifecycle management ensures that data is accessible and available when and where it is needed. This enables timely decision-making, improved collaboration, and better customer service.
Contact us
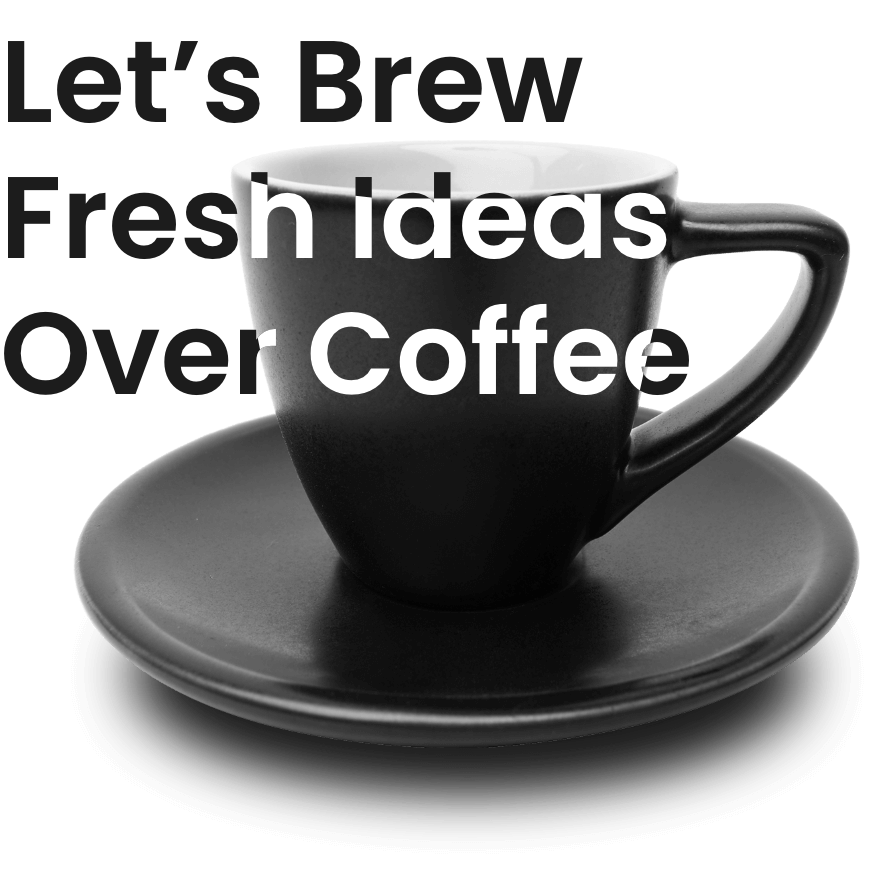
Spanning 8 cities worldwide and with partners in 100 more, we’re your local yet global agency.
Fancy a coffee, virtual or physical? It’s on us – let’s connect!