The impact of MDM on data quality metrics and KPIs
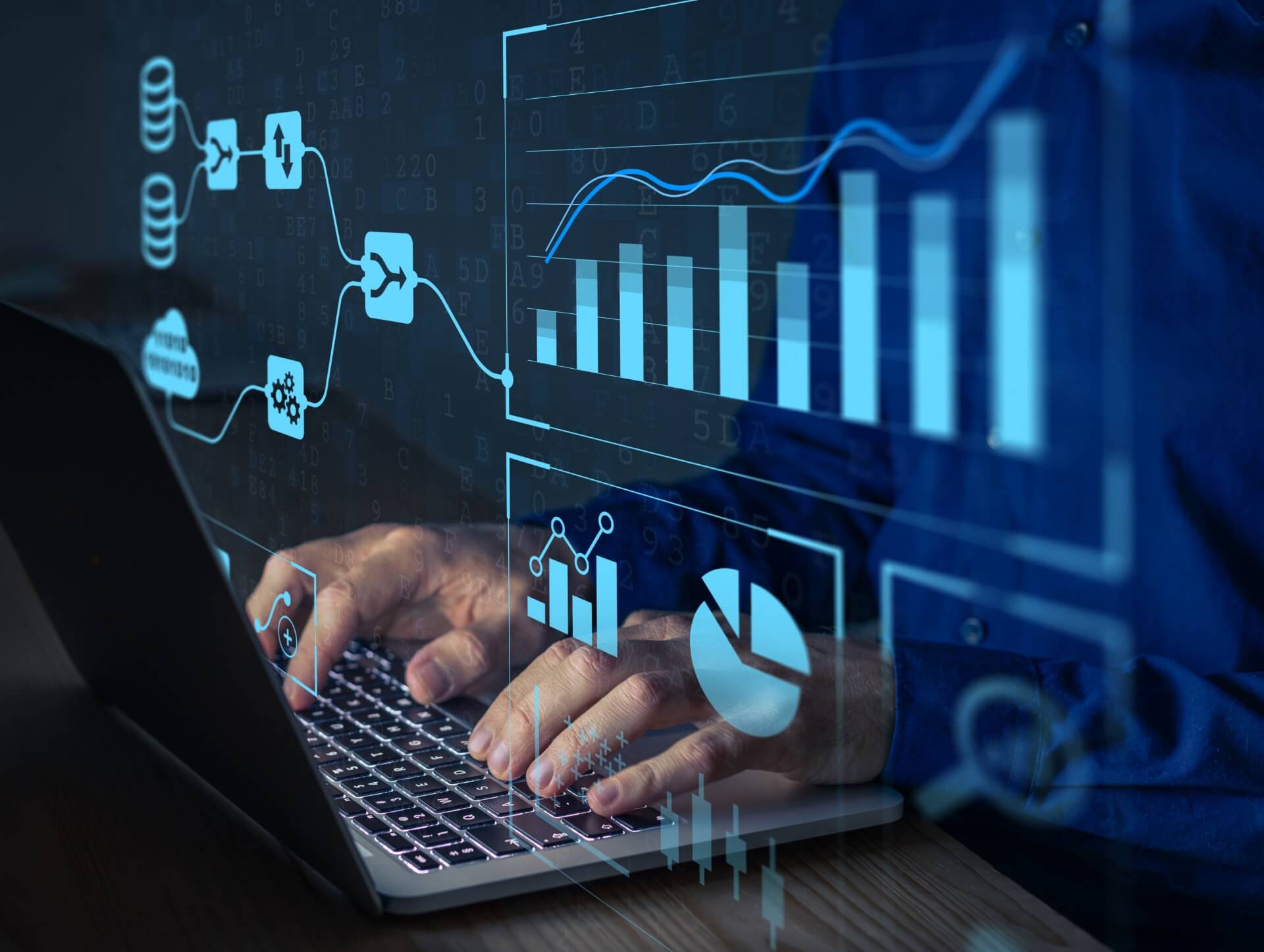
06/09/2023
Master Data Management (MDM) is a comprehensive approach to managing and governing an organization's critical data assets. It involves the creation, maintenance, and synchronization of a single, trusted version of data across multiple systems and business units. MDM plays a crucial role in ensuring data quality, which is essential for accurate reporting, decision-making, and compliance.
Benefits of Master Data Management
Implementing an effective MDM strategy can bring several benefits to an organization. Some of the key benefits include:
1. Improved Data Quality
MDM helps in improving data quality by ensuring that data is accurate, consistent, and up-to-date. By implementing data quality management processes and tools, organizations can identify and fix data issues, resulting in better data-driven decision-making and improved business outcomes.
2. Enhanced Data Governance
Data governance is an essential component of MDM. It involves defining policies, procedures, and rules for managing data assets. MDM provides a framework for implementing and enforcing data governance practices, ensuring data is managed in a consistent and controlled manner throughout its lifecycle.
3. Increased Operational Efficiency
By streamlining data management processes and eliminating duplicate or inconsistent data, MDM helps in increasing operational efficiency. With a single, trusted view of data, organizations can reduce data entry errors, eliminate data silos, and improve data integration and sharing across systems and departments.
4. Improved Customer Experience
Customer Master Data Management (CMDM) is a specialized area of MDM that focuses on managing customer data. By having a complete and accurate view of customer information, organizations can deliver personalized experiences, improve customer service, and enhance customer satisfaction and loyalty.
MDM and Data Quality Metrics
Data quality metrics are used to measure the accuracy, completeness, consistency, and timeliness of data. MDM plays a significant role in improving data quality metrics by:
1. Ensuring Data Accuracy
MDM ensures data accuracy by eliminating duplicate and inconsistent data. It provides mechanisms to identify and resolve data conflicts and discrepancies, ensuring that the data is correct and reliable.
2. Enforcing Data Consistency
MDM establishes data standards and rules to ensure data consistency across systems and processes. It helps in avoiding data discrepancies and conflicts that can arise from using multiple sources of data.
3. Enhancing Data Completeness
MDM ensures data completeness by consolidating data from various sources and systems. It helps in identifying missing data and filling the gaps to create a complete and comprehensive view of data.
4. Improving Data Timeliness
MDM facilitates real-time data integration and synchronization, ensuring that data is updated and available in a timely manner. It helps in avoiding delays in data availability and enables organizations to make timely decisions based on the most recent data.
KPIs for Data Quality in MDM
Key Performance Indicators (KPIs) are used to measure the performance and effectiveness of data quality initiatives in MDM. Some of the common KPIs for data quality in MDM include:
1. Data Accuracy Rate
This KPI measures the percentage of accurate and error-free data in the MDM system. It helps in assessing the effectiveness of data cleansing and validation processes.
2. Data Completeness Rate
This KPI measures the percentage of complete and comprehensive data in the MDM system. It helps in evaluating the success of data consolidation and integration efforts.
3. Data Consistency Rate
This KPI measures the level of consistency and uniformity in data across systems and processes. It helps in identifying and resolving data conflicts and discrepancies.
4. Data Timeliness Rate
This KPI measures the speed and accuracy of data updates and availability. It helps in assessing the efficiency of data synchronization and integration processes.
MDM Strategies for Data Quality Improvement
Implementing an effective MDM strategy is critical for achieving data quality improvement. Some of the best practices and strategies for data quality improvement in MDM include:
1. Establishing Data Governance Framework
Data governance is the foundation of data quality improvement in MDM. Establishing a data governance framework helps in defining data ownership, accountability, and stewardship roles and responsibilities.
2. Implementing Data Quality Management Processes
Implementing data quality management processes and tools helps in identifying and fixing data issues. This includes data profiling, data cleansing, data validation, and data enrichment processes.
3. Ensuring Data Integration and Synchronization
Integrating and synchronizing data across systems and processes is essential for maintaining data consistency and accuracy. This can be achieved through data integration tools and technologies.
4. Providing Data Security and Privacy
Data security and privacy are critical for ensuring data quality. Implementing data security measures, such as access controls, encryption, and data masking, helps in protecting sensitive data from unauthorized access and breaches.
Challenges in MDM and Data Quality
Implementing an MDM strategy for data quality improvement is not without its challenges. Some of the common challenges include:
1. Data Complexity
Managing and integrating complex and heterogeneous data sources can be challenging. Different data formats, structures, and semantics can pose difficulties in ensuring data quality.
2. Data Governance
Establishing and maintaining a robust data governance framework requires significant effort and resources. Lack of proper data governance can lead to inconsistent data quality across systems and processes.
3. Data Integration
Integrating data from various systems and sources can be complex and time-consuming. Data integration challenges can affect data quality and accuracy.
4. Data Security and Privacy
Ensuring data security and privacy is a critical challenge in MDM. Protecting sensitive data from unauthorized access and breaches requires robust security measures.
Conclusion
Master Data Management plays a crucial role in improving data quality metrics and KPIs. By implementing an effective MDM strategy, organizations can ensure data accuracy, consistency, completeness, and timeliness. This, in turn, leads to better decision-making, improved operational efficiency, enhanced customer experience, and compliance with data regulations. Despite the challenges, the benefits of MDM in improving data quality make it an essential investment for organizations seeking to leverage the power of their data.
Contact us
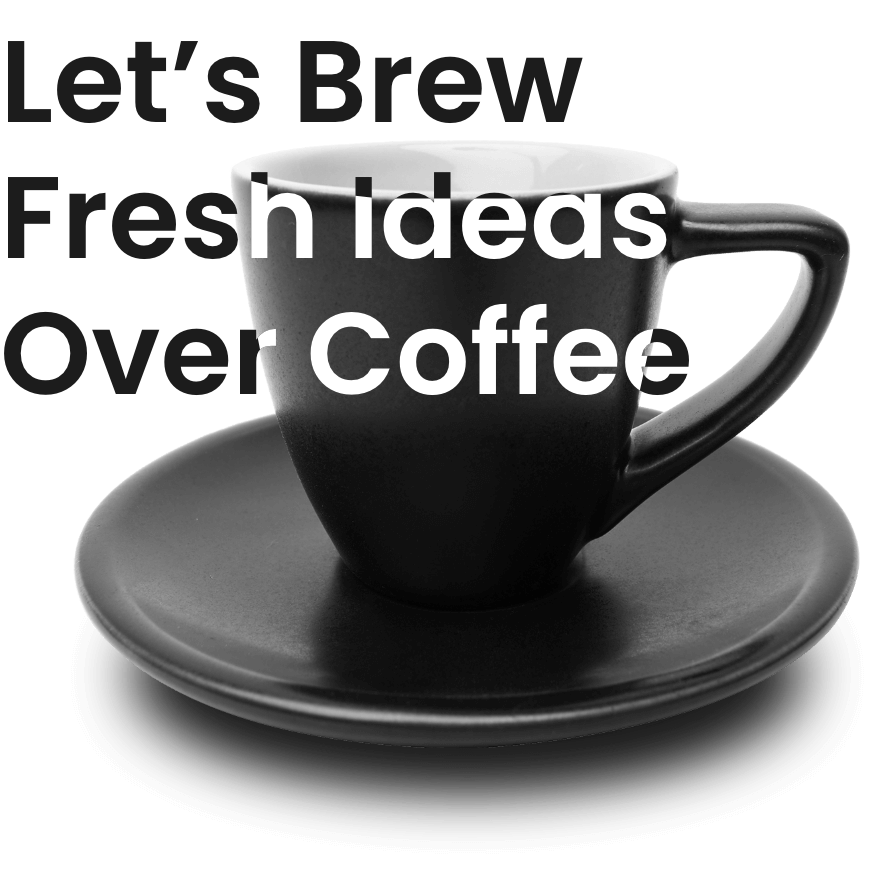
Spanning 8 cities worldwide and with partners in 100 more, we’re your local yet global agency.
Fancy a coffee, virtual or physical? It’s on us – let’s connect!