The impact of MDM on data synchronization and consistency in enterprise scalability
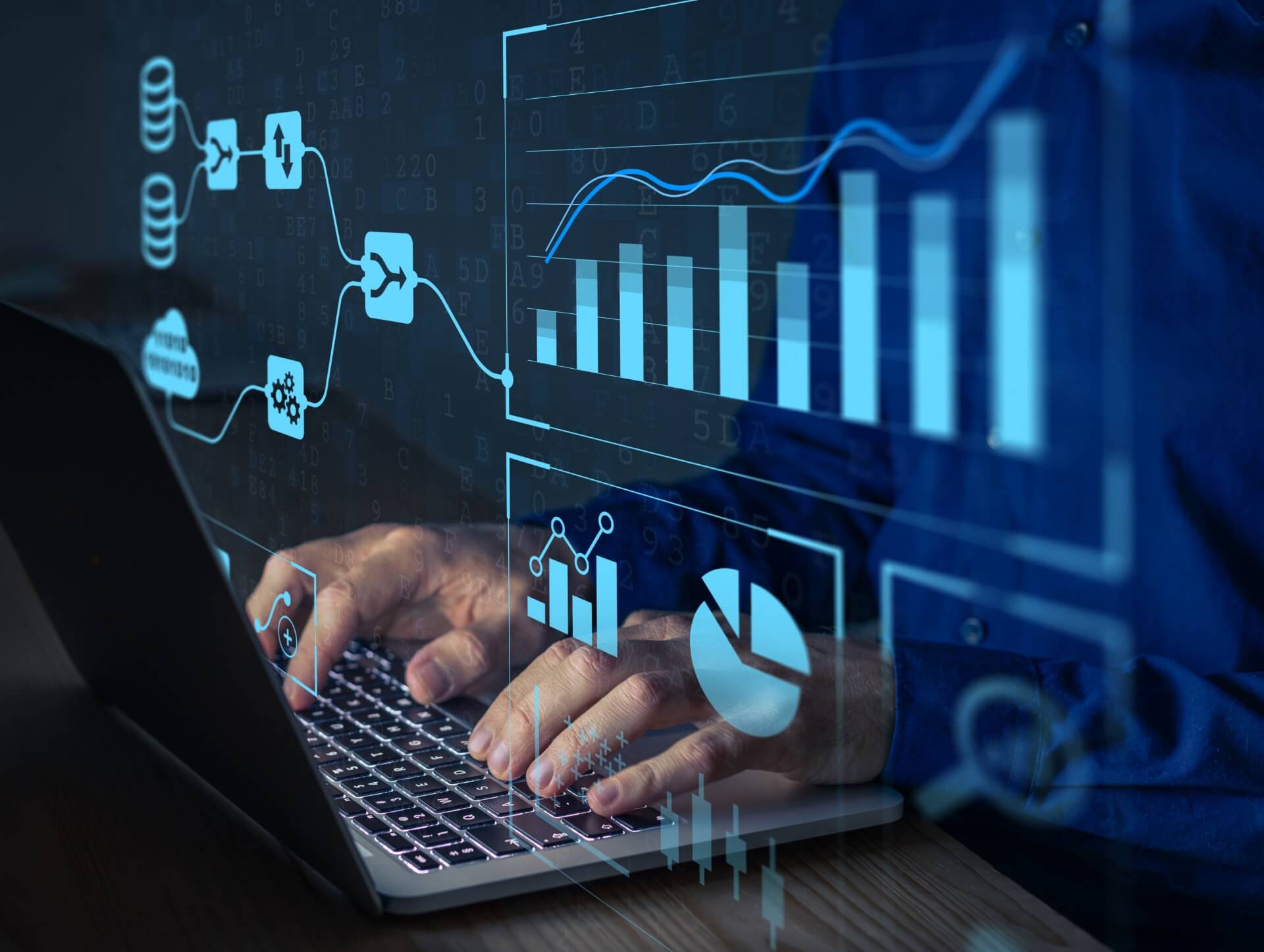
06/09/2023
Master Data Management (MDM) is a comprehensive approach to managing and harmonizing an organization's critical data assets. It involves creating a single, consistent, and accurate view of key data entities such as customers, products, and suppliers. MDM solutions provide businesses with the ability to improve data quality, streamline data integration, ensure data governance, and enable better decision-making.
The Benefits of MDM
Implementing a master data management strategy offers numerous benefits to organizations:
- Improved Data Quality: MDM ensures that data is accurate, complete, and consistent across various systems and processes. By establishing data quality management practices, organizations can trust the data they rely on for critical operations and decision-making.
- Enhanced Data Integration: MDM enables the integration of data from disparate sources into a centralized repository. This facilitates data sharing and collaboration across different departments and systems, leading to improved operational efficiency.
- Efficient Data Governance: MDM provides a framework for data governance, ensuring that data is managed and controlled effectively throughout its lifecycle. This includes defining data standards, roles and responsibilities, and implementing data policies and procedures.
- Better Decision-Making: With a single, unified view of key data entities, organizations can make more informed and accurate decisions. MDM enables businesses to analyze data holistically, identify trends, and uncover insights that drive strategic initiatives.
MDM Implementation Process
The implementation of an MDM solution involves several key steps:
- Assessment and Planning: Organizations need to assess their current data management practices, identify gaps and challenges, and define their MDM goals and objectives. This includes evaluating the existing data infrastructure, data quality, and data governance processes.
- Master Data Modeling: The next step is to define the data model for the MDM solution. This involves identifying the key data entities, their attributes, relationships, and hierarchies. A well-designed data model is crucial for establishing data consistency and synchronization.
- MDM Platform Selection: Organizations need to evaluate and select an MDM platform that aligns with their requirements and goals. Factors to consider include scalability, flexibility, integration capabilities, security features, and vendor support.
- Data Integration and Cleansing: Once the MDM platform is in place, organizations need to integrate and cleanse their data. This involves extracting data from various sources, transforming it to match the defined data model, and loading it into the MDM repository.
- Data Governance Implementation: Establishing data governance processes and practices is essential for ensuring the ongoing management and maintenance of the MDM solution. This includes defining data stewardship roles, implementing data quality rules, and monitoring data compliance.
- Change Management and User Adoption: Successful MDM implementation requires effective change management and user adoption strategies. Organizations need to communicate the benefits of the MDM solution, provide training to users, and address any resistance or concerns.
MDM Best Practices
Implementing MDM successfully involves following best practices:
- Start with a Clear Strategy: Define your MDM goals, objectives, and success criteria. Align your MDM strategy with your overall business objectives.
- Engage Stakeholders: Involve key stakeholders from different business units in the MDM implementation process. Their input and buy-in are crucial for the success of the initiative.
- Ensure Data Quality: Implement data quality management practices to ensure the accuracy, completeness, and consistency of your data. Define data quality rules and monitor data compliance.
- Establish Data Governance: Develop a comprehensive data governance framework to manage and control your data effectively. Define data standards, roles and responsibilities, and data policies and procedures.
- Implement Incrementally: Start small and gradually expand your MDM implementation. This allows for better control and reduces the risk of disruptions to critical business processes.
- Monitor and Measure: Continuously monitor the performance and effectiveness of your MDM solution. Establish key performance indicators (KPIs) to track progress and measure the ROI of your MDM investment.
Challenges in MDM
Implementing an MDM solution can be challenging due to various factors:
- Data Complexity: Organizations often have complex data landscapes with data scattered across multiple systems, departments, and formats. Consolidating and harmonizing this data can be a complex and time-consuming process.
- Data Governance: Establishing effective data governance practices requires organizational alignment, clear roles and responsibilities, and a culture of data stewardship. Lack of data governance can result in poor data quality and inconsistencies.
- Change Management: MDM implementation involves significant changes to data management processes and systems. Organizations need to manage change effectively and address resistance from users who are accustomed to existing data practices.
- Data Security: MDM involves consolidating and centralizing critical data assets, making data security a top concern. Organizations need to implement robust security measures to protect sensitive data from unauthorized access or breaches.
- Data Integration: Integrating data from disparate sources can be complex, especially when dealing with different data formats, structures, and systems. Ensuring data integration without loss of information or data quality is a significant challenge.
MDM and Enterprise Scalability
MDM plays a crucial role in enabling enterprise scalability by providing a foundation for efficient data management and integration:
- Centralized Data Repository: MDM creates a centralized repository for critical data entities, ensuring a single source of truth. This simplifies data management and reduces duplication, leading to improved scalability.
- Data Consistency and Synchronization: MDM ensures data consistency and synchronization across different systems and processes. This enables organizations to scale without sacrificing data accuracy or integrity.
- Streamlined Data Integration: MDM enables efficient data integration by providing a standardized data model and integration framework. This simplifies the process of integrating data from new systems or acquisitions, facilitating scalability.
- Improved Data Quality: By enforcing data quality rules and processes, MDM improves the overall quality of data. This ensures that organizations can rely on accurate and reliable data as they scale.
- Enhanced Data Governance: MDM establishes data governance processes and practices that scale along with the organization. This enables organizations to maintain control and compliance over their data as they grow.
MDM and Data Security
Data security is a critical aspect of MDM implementation:
- Data Privacy: MDM solutions need to comply with data privacy regulations and protect sensitive customer information. This includes implementing access controls, encryption, and anonymization techniques.
- Data Access Controls: MDM platforms should provide robust access controls to ensure that only authorized users can access and modify data. This includes role-based access controls and user authentication mechanisms.
- Data Encryption: MDM solutions should support data encryption to protect data at rest and in transit. Encryption ensures that even if data is compromised, it remains unreadable without the encryption keys.
- Data Masking: MDM platforms can employ data masking techniques to replace sensitive data with fictional or anonymized data for non-production environments. This minimizes the risk of unauthorized access to sensitive data.
- Data Auditing and Monitoring: MDM solutions should provide auditing and monitoring capabilities to track data access and modifications. This allows organizations to detect and investigate any suspicious activities.
MDM and Data Analytics
MDM provides a foundation for effective data analytics:
- Integrated Data: MDM enables the integration of data from disparate sources, creating a unified view of key data entities. This integrated data can be used for advanced analytics and reporting, enabling organizations to gain insights and make data-driven decisions.
- Improved Data Quality: MDM ensures data accuracy, completeness, and consistency, which are crucial for reliable data analytics. Clean and reliable data is the foundation for accurate and meaningful insights.
- Data Governance: MDM establishes data governance practices that ensure data is managed and controlled effectively. This includes defining data standards, enforcing data quality rules, and implementing data policies and procedures.
- Master Data Modeling: MDM involves defining a data model for key data entities. This data model provides a structure for data analytics, enabling organizations to perform advanced analytics and reporting on their master data.
- Data Integration: MDM simplifies the process of integrating data from different sources, making it easier to combine and analyze data for insights and decision-making.
Challenges in MDM
Implementing an MDM solution can be challenging due to various factors:
- Data Complexity: Organizations often have complex data landscapes with data scattered across multiple systems, departments, and formats. Consolidating and harmonizing this data can be a complex and time-consuming process.
- Data Governance: Establishing effective data governance practices requires organizational alignment, clear roles and responsibilities, and a culture of data stewardship. Lack of data governance can result in poor data quality and inconsistencies.
- Change Management: MDM implementation involves significant changes to data management processes and systems. Organizations need to manage change effectively and address resistance from users who are accustomed to existing data practices.
- Data Security: MDM involves consolidating and centralizing critical data assets, making data security a top concern. Organizations need to implement robust security measures to protect sensitive data from unauthorized access or breaches.
- Data Integration: Integrating data from disparate sources can be complex, especially when dealing with different data formats, structures, and systems. Ensuring data integration without loss of information or data quality is a significant challenge.
MDM Case Studies
Several organizations have successfully implemented MDM solutions to address their data management challenges:
- Company A: Company A, a global retailer, implemented an MDM solution to consolidate and manage customer data across its various sales channels. This enabled the company to provide a consistent and personalized customer experience, leading to increased customer satisfaction and loyalty.
- Company B: Company B, a healthcare organization, implemented an MDM solution to manage patient data across its multiple systems and departments. This improved data accuracy and accessibility, enabling better patient care and streamlined administrative processes.
- Company C: Company C, a financial services company, implemented an MDM solution to manage its product data. This enabled the company to streamline its product catalog, ensure data consistency across different channels, and launch new products more efficiently.
Conclusion
Master Data Management plays a critical role in ensuring data synchronization and consistency in enterprise scalability. By implementing an MDM solution, organizations can improve data quality, streamline data integration, ensure data governance, and enable better decision-making. MDM provides a foundation for efficient data management and integration, enabling organizations to scale without sacrificing data accuracy or integrity. However, implementing MDM also comes with its challenges, including data complexity, data governance, change management, data security, and data integration. By following best practices and learning from successful case studies, organizations can overcome these challenges and unlock the full potential of MDM.
Contact us
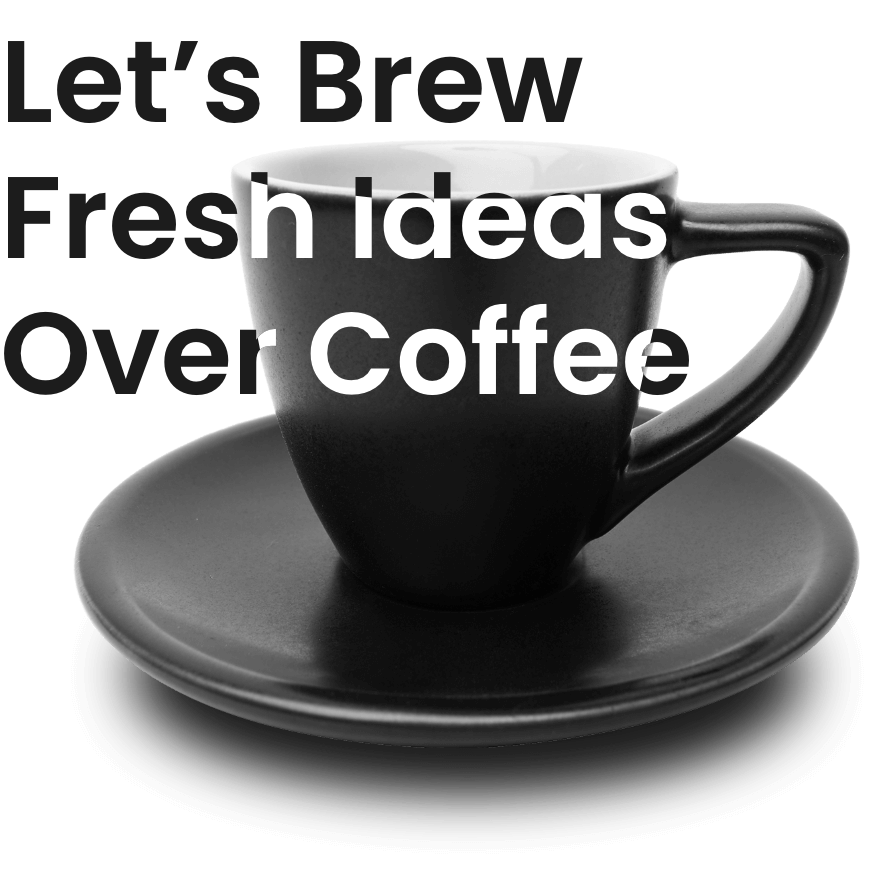
Spanning 8 cities worldwide and with partners in 100 more, we’re your local yet global agency.
Fancy a coffee, virtual or physical? It’s on us – let’s connect!