The importance of master data modeling in data-driven decision-making in the automotive industry
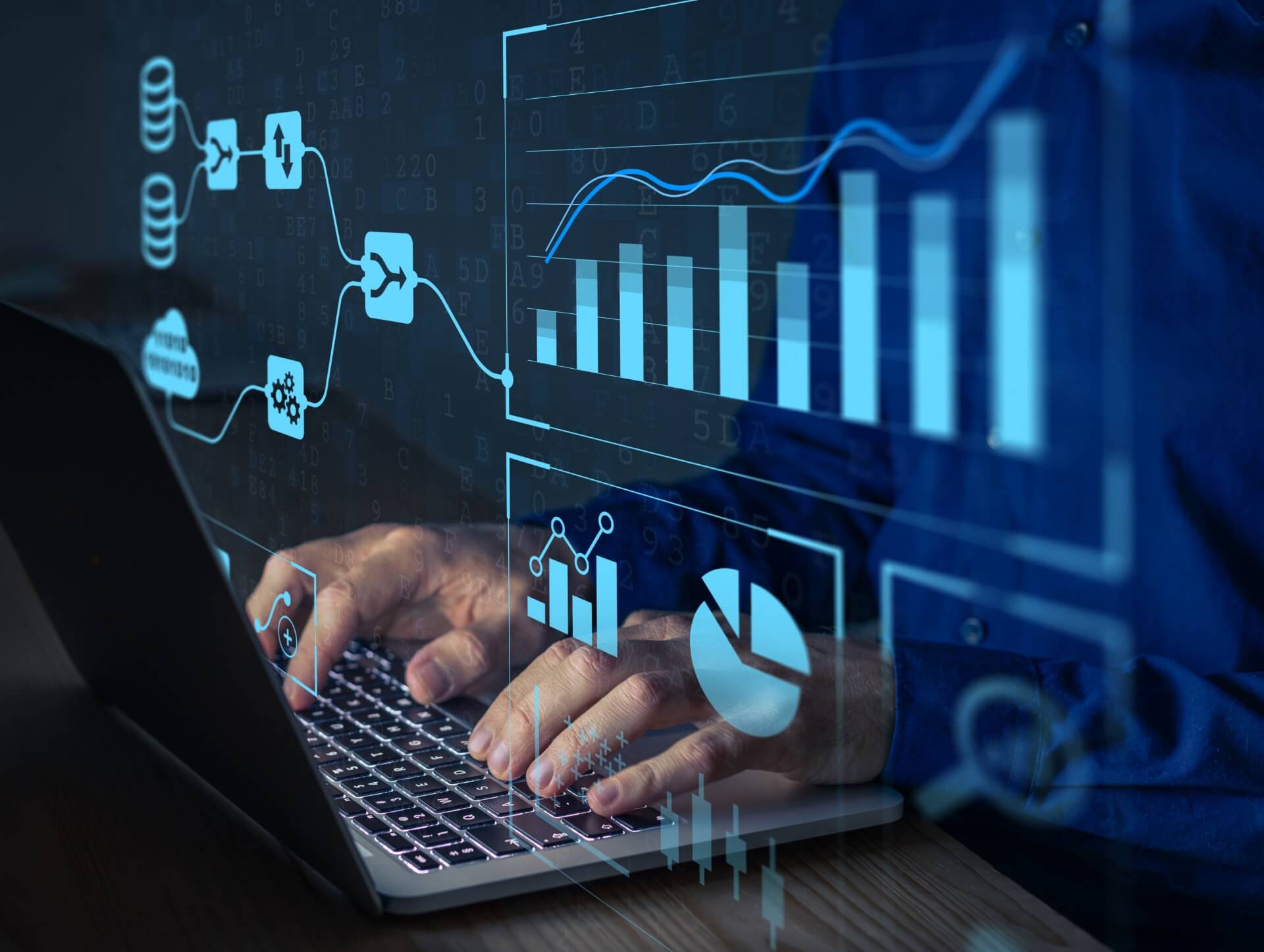
06/09/2023
In today's data-driven world, organizations across industries are realizing the importance of harnessing the power of data to make informed decisions. The automotive industry, in particular, has witnessed a significant shift towards data-driven decision-making to drive innovation, improve efficiency, and enhance customer experience. One crucial aspect of data-driven decision-making in the automotive industry is master data modeling.
What is Master Data Modeling?
Master data modeling is the process of creating a structured representation of an organization's critical data elements, called master data. Master data represents the core business entities, such as customers, products, suppliers, and locations, which are shared across different systems and departments within an organization. Master data modeling involves defining the attributes, relationships, and hierarchies of these entities to ensure consistency, accuracy, and integrity of data throughout its lifecycle.
The Role of Master Data Modeling in Data-Driven Decision-Making
Master data modeling plays a crucial role in data-driven decision-making in the automotive industry. Here are some key reasons why:
1. Improved Data Quality and Consistency
Master data modeling ensures that the master data is structured, standardized, and consistent across the organization. By defining the attributes and relationships of master data entities, organizations can establish data quality rules and validations to ensure that the data is accurate, complete, and up-to-date. This high-quality data serves as a solid foundation for data-driven decision-making, enabling organizations to make informed choices based on reliable information.
2. Enhanced Data Integration and Interoperability
In the automotive industry, data is generated and stored in various systems and databases, such as customer relationship management (CRM) systems, enterprise resource planning (ERP) systems, and supply chain management systems. Master data modeling provides a unified view of the master data across these systems, allowing for seamless data integration and interoperability. This integrated data ecosystem enables organizations to analyze and derive insights from the data holistically, leading to more accurate and comprehensive decision-making.
3. Facilitates Data Governance and Compliance
Data governance is essential in the automotive industry to ensure data privacy, security, and compliance with regulations such as General Data Protection Regulation (GDPR) and California Consumer Privacy Act (CCPA). Master data modeling helps establish data governance frameworks by defining data ownership, access controls, and data stewardship roles and responsibilities. This ensures that the data is managed and protected throughout its lifecycle, mitigating risks and ensuring compliance with industry standards and regulations.
4. Enables Advanced Analytics and Insights
Master data modeling provides a solid foundation for advanced analytics and insights in the automotive industry. By structuring and organizing the master data, organizations can leverage various analytics techniques, such as data mining, machine learning, and predictive analytics, to derive valuable insights from the data. These insights can help organizations identify trends, patterns, and anomalies, enabling them to make data-driven decisions that drive innovation, improve operational efficiency, and enhance customer experience.
Benefits of Master Data Modeling in the Automotive Industry
Implementing a robust master data modeling strategy in the automotive industry can yield several benefits for organizations. Here are some key advantages:
1. Improved Customer Experience
Master data modeling enables organizations to have a unified view of customer data, including contact information, purchase history, and preferences. This comprehensive view of customer master data allows organizations to personalize their interactions, provide targeted marketing campaigns, and deliver exceptional customer experiences. By understanding customer needs and preferences, organizations can tailor their products and services to meet customer expectations, driving customer loyalty and satisfaction.
2. Streamlined Supply Chain Management
Master data modeling helps organizations streamline their supply chain management processes by providing accurate and up-to-date information about suppliers, products, and inventory. With a comprehensive view of supplier master data, organizations can optimize their supplier selection, negotiate better contracts, and ensure timely delivery of goods. Additionally, by integrating product master data with supply chain management systems, organizations can track and manage inventory effectively, reducing stockouts and improving overall supply chain efficiency.
3. Enhanced Decision-Making and Innovation
Master data modeling enables organizations to make data-driven decisions based on reliable and accurate information. By having a unified view of master data, organizations can analyze and derive insights from the data to drive innovation and improve decision-making. For example, by analyzing product master data, organizations can identify opportunities for product enhancements, new product development, and market expansion. Similarly, by analyzing customer master data, organizations can identify customer preferences and trends, enabling them to develop targeted marketing strategies and improve customer satisfaction.
4. Increased Operational Efficiency
Master data modeling helps organizations improve operational efficiency by eliminating duplicate and inconsistent data, automating data entry processes, and reducing manual errors. By ensuring data accuracy and consistency, organizations can reduce the time and effort required for data cleaning and reconciliation. This allows employees to focus on value-added activities, such as data analysis and decision-making, leading to improved productivity and operational efficiency.
Implementing Master Data Modeling in the Automotive Industry
Implementing master data modeling in the automotive industry requires a well-defined strategy and a robust master data management (MDM) solution. Here are the key steps involved in the implementation process:
1. Define MDM Strategy and Goals
Before implementing master data modeling, organizations need to define their MDM strategy and goals. This involves identifying the critical master data entities, determining the required attributes and relationships, and establishing data quality and governance rules. Organizations should also align their MDM strategy with their overall business objectives to ensure that the master data modeling efforts contribute to the organization's success.
2. Select the Right MDM Solution
Choosing the right MDM solution is crucial for successful master data modeling implementation. Organizations can opt for on-premises MDM solutions, cloud-based MDM solutions, or a combination of both, depending on their specific requirements. It is essential to evaluate different MDM vendors and consider factors such as scalability, data security, integration capabilities, and user-friendliness before selecting the right MDM solution.
3. Design and Implement Master Data Model
Once the MDM strategy and solution are in place, organizations can proceed with designing and implementing the master data model. This involves identifying the attributes and relationships of master data entities, defining data quality rules, and establishing data governance processes. Organizations should also consider data integration requirements and ensure that the master data model aligns with other data models and systems within the organization.
4. Data Migration and Integration
After designing the master data model, organizations need to migrate and integrate the existing data into the new MDM solution. This involves data cleansing, data mapping, and data transformation processes to ensure that the data is accurately migrated and integrated. Organizations should also implement data quality checks and validations during the migration process to maintain data integrity and consistency.
5. Continuous Monitoring and Improvement
Master data modeling is an ongoing process that requires continuous monitoring and improvement. Organizations should establish data governance frameworks and processes to ensure that the master data remains accurate, consistent, and up-to-date. Regular data quality audits, data profiling, and data cleansing activities should be conducted to identify and rectify any data issues. Additionally, organizations should stay abreast of the latest MDM tools and technologies to leverage new features and functionalities that can further enhance their master data modeling efforts.
Challenges in Master Data Modeling
Implementing master data modeling in the automotive industry comes with its own set of challenges. Some common challenges include:
1. Data Complexity and Volume
The automotive industry generates vast amounts of data from various sources, including IoT devices, sensors, and connected vehicles. Managing and modeling this data can be challenging due to its complexity and volume. Organizations need to invest in scalable MDM solutions and data management technologies to handle the increasing data volumes effectively.
2. Data Security and Privacy
Data security and privacy are critical concerns in the automotive industry, especially with the rise in cyber threats and data breaches. Organizations need to implement robust data security measures, such as encryption, access controls, and user authentication, to protect the master data from unauthorized access and ensure compliance with data protection regulations.
3. Data Integration and Interoperability
The automotive industry relies on various systems and databases to store and manage data. Integrating and ensuring interoperability between these systems can be challenging. Organizations need to invest in MDM solutions that support seamless data integration and provide data connectors and APIs to connect with different systems and databases.
4. Data Governance and Stewardship
Establishing effective data governance and stewardship processes is crucial for successful master data modeling. Assigning data ownership, defining data governance policies, and training data stewards can be challenging tasks. Organizations need to invest in data governance tools and technologies that automate data governance processes and provide a clear framework for data stewardship.
Case Studies: Successful Implementation of Master Data Modeling in the Automotive Industry
Several organizations in the automotive industry have successfully implemented master data modeling to drive their data-driven decision-making. Here are two case studies:
1. Case Study 1: Company XYZ
Company XYZ, a leading automotive manufacturer, implemented a robust master data modeling strategy to improve its customer experience. By integrating customer master data from various systems, including sales, marketing, and customer service, Company XYZ gained a unified view of its customers. This enabled the organization to personalize its marketing campaigns, provide targeted offers, and deliver exceptional customer service. As a result, Company XYZ saw a significant increase in customer satisfaction and loyalty.
2. Case Study 2: Company ABC
Company ABC, a global automotive supplier, implemented a cloud-based master data management platform to streamline its supply chain management processes. By integrating product master data with its supply chain management system, Company ABC gained real-time visibility into inventory levels, demand forecasts, and supplier performance. This enabled the organization to optimize its inventory management, reduce stockouts, and improve overall supply chain efficiency. Company ABC also leveraged data analytics to identify opportunities for cost savings and process improvements.
Conclusion
Master data modeling plays a crucial role in data-driven decision-making in the automotive industry. By implementing a robust master data modeling strategy and leveraging the right master data management solutions, organizations can improve data quality and consistency, enhance data integration and interoperability, ensure data governance and compliance, and enable advanced analytics and insights. The benefits of master data modeling in the automotive industry include improved customer experience, streamlined supply chain management, enhanced decision-making and innovation, and increased operational efficiency. Despite the challenges, organizations can achieve significant ROI and value by effectively implementing master data modeling and embracing data-driven decision-making in the automotive industry.
Contact us
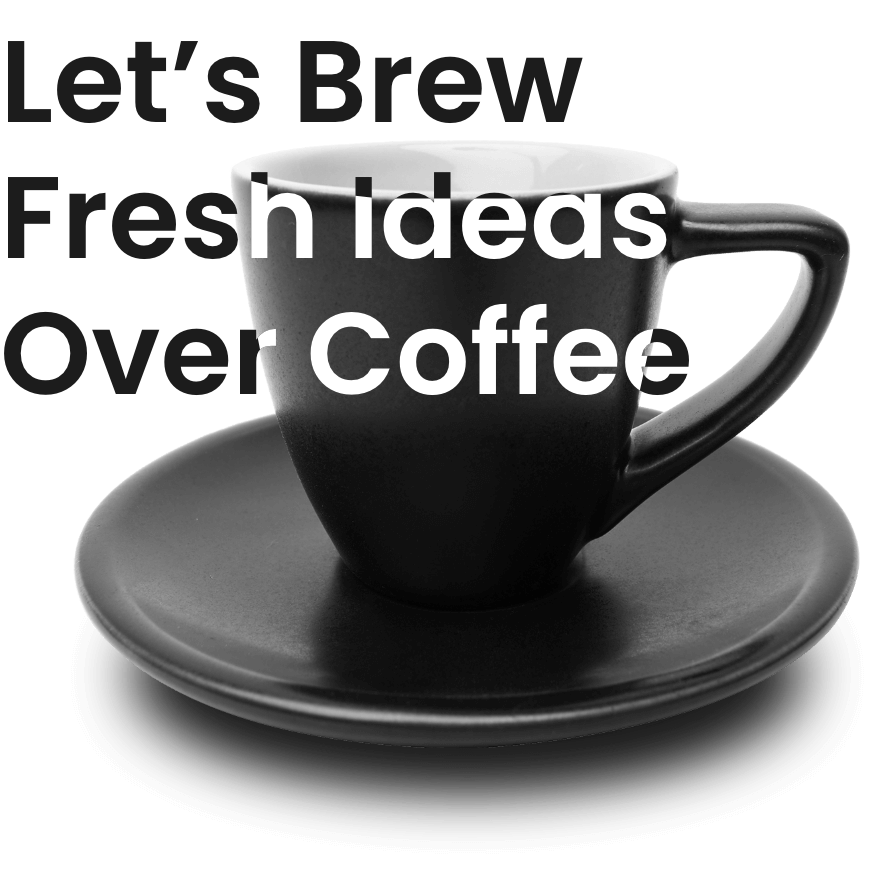
Spanning 8 cities worldwide and with partners in 100 more, we’re your local yet global agency.
Fancy a coffee, virtual or physical? It’s on us – let’s connect!