The importance of master data modeling in data-driven decision-making in the technology sector
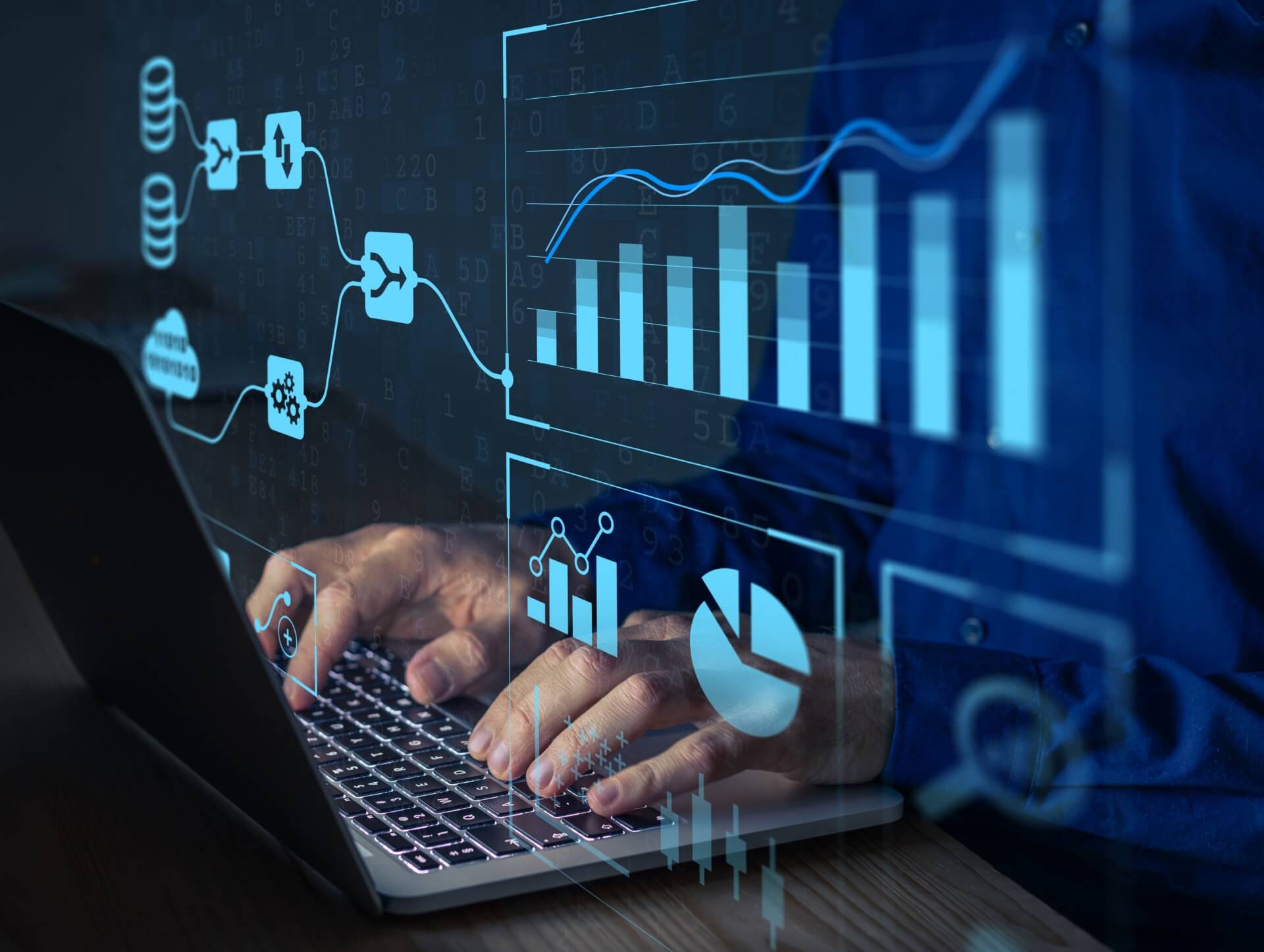
06/09/2023
In today's technology-driven world, data is the lifeblood of businesses. Organizations across industries are collecting vast amounts of data from various sources, including customer interactions, online transactions, and operational processes. However, the true value of data lies in its ability to drive informed decision-making. This is where master data modeling plays a crucial role.
What is Master Data Modeling?
Master data modeling is the process of creating a standardized and unified view of an organization's critical data elements. It involves identifying and defining the key data entities, attributes, and relationships that are important for business operations. By creating a comprehensive and consistent master data model, organizations can ensure that their data is accurate, reliable, and accessible across different systems and applications.
The Role of Master Data Management (MDM)
Master data modeling is closely tied to the concept of Master Data Management (MDM). MDM refers to the processes, technologies, and strategies that organizations implement to manage their master data effectively. It involves creating a single, authoritative source of truth for critical data elements such as customer information, product data, and financial records.
MDM solutions provide organizations with a centralized platform to manage and govern their master data. These solutions enable data integration, data quality management, and data governance, ensuring that organizations have reliable and consistent data to make informed decisions.
The Benefits of Effective Master Data Modeling
Effective master data modeling and management offer several benefits to organizations in the technology sector:
1. Improved Data Quality
With a well-defined master data model, organizations can ensure that their data is accurate, complete, and consistent. By implementing data quality management processes and tools, organizations can identify and resolve data issues, such as duplicate records, incorrect values, or missing information. Improved data quality leads to better decision-making and more reliable business insights.
2. Enhanced Data Governance
Data governance is a critical aspect of master data management. It involves establishing policies, processes, and controls to ensure data integrity, privacy, and compliance. By implementing a robust data governance framework, organizations can ensure that their master data is governed effectively throughout its lifecycle. This includes defining data ownership, establishing data stewardship roles, and enforcing data security and privacy measures.
3. Increased Operational Efficiency
Efficient data management is essential for organizations to streamline their operations and drive efficiency. With a well-designed master data model, organizations can eliminate data silos and ensure that data is shared seamlessly across departments and systems. This leads to improved collaboration, reduced manual data entry, and faster access to accurate information, ultimately increasing operational efficiency.
4. Better Decision-Making
Data-driven decision-making is a key driver of success in the technology sector. By leveraging accurate and up-to-date master data, organizations can make informed decisions based on real-time insights. Effective master data modeling enables organizations to analyze and visualize data more effectively, identify trends and patterns, and make data-driven decisions that lead to better business outcomes.
Key Considerations in Master Data Modeling
When implementing master data modeling in the technology sector, organizations should consider the following key factors:
1. Data Integration
Data integration is the process of combining data from different sources into a unified view. In the context of master data modeling, organizations need to ensure that their master data is integrated seamlessly across various systems and applications. This requires establishing data integration processes and leveraging integration technologies to ensure data consistency and accuracy.
2. Data Security
Data security is a top concern for organizations in the technology sector. When designing a master data model, organizations must consider data security measures to protect sensitive information from unauthorized access or breaches. This includes implementing encryption, access controls, and data masking techniques to ensure data security throughout its lifecycle.
3. Data Analytics
Data analytics is a powerful tool for extracting insights from data. When designing a master data model, organizations should consider how the data will be used for analytics purposes. This includes ensuring that the data model supports the necessary data structures and relationships for effective analysis and visualization.
4. Customer Experience
Customer experience is a critical factor in the success of technology companies. Effective master data modeling enables organizations to have a unified view of their customers, including their preferences, purchase history, and interactions. This allows organizations to deliver personalized and seamless customer experiences, leading to increased customer satisfaction and loyalty.
Challenges in Master Data Modeling
While master data modeling offers numerous benefits, organizations may face several challenges during the implementation process:
1. Data Quality Management
Ensuring data quality is a complex task, as organizations may have data stored in various formats and systems. Cleaning and standardizing data can be time-consuming and resource-intensive. Organizations need to invest in data quality management tools and processes to address these challenges effectively.
2. Data Governance
Establishing a robust data governance framework is essential for effective master data modeling. Organizations need to define data ownership, establish data stewardship roles, and ensure compliance with data privacy regulations. This requires a strong commitment from the leadership team and collaboration across departments.
3. Data Integration
Data integration can be a complex process, especially when organizations have multiple systems and applications. Ensuring that data is integrated seamlessly and accurately requires careful planning, system compatibility, and data mapping exercises. Organizations should consider leveraging integration technologies and expertise to overcome these challenges.
4. Change Management
Implementing master data modeling requires organizational change and alignment. Employees need to be trained on the new processes and tools, and their roles and responsibilities may change. Change management strategies should be implemented to ensure a smooth transition and maximize user adoption.
Conclusion
In conclusion, master data modeling plays a crucial role in data-driven decision-making in the technology sector. It enables organizations to create a unified and standardized view of their critical data, ensuring accuracy, reliability, and accessibility. By implementing effective master data management strategies, organizations can improve data quality, enhance data governance, increase operational efficiency, and make better-informed decisions. However, organizations must also address challenges such as data quality management, data governance, data integration, and change management to maximize the benefits of master data modeling.
Contact us
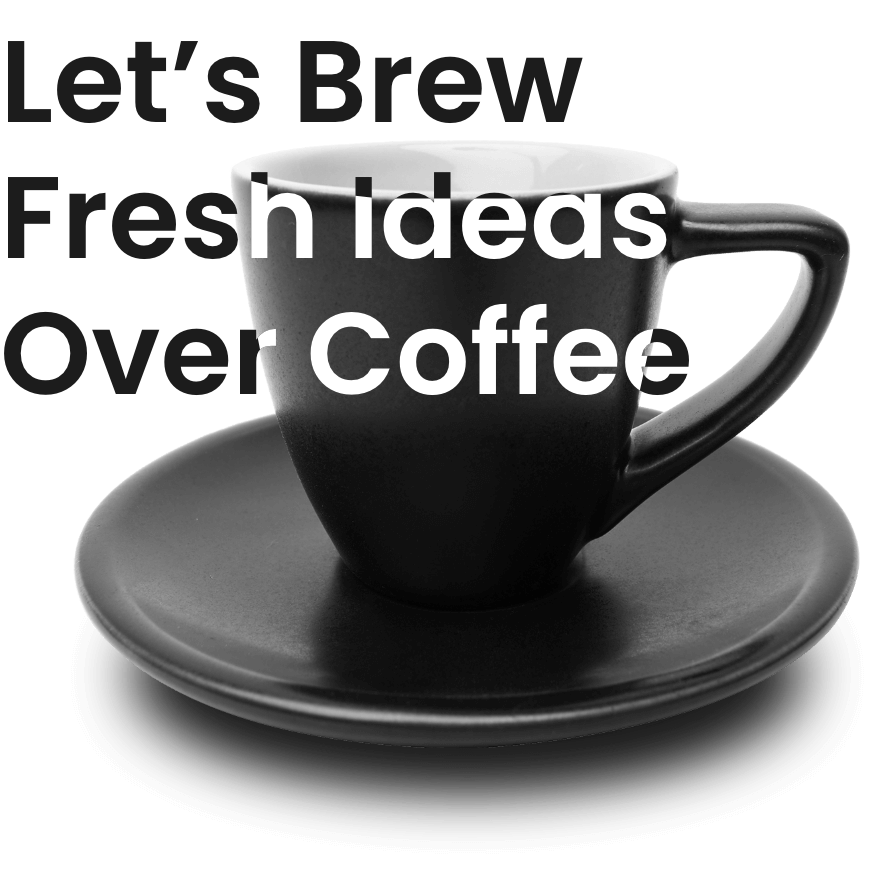
Spanning 8 cities worldwide and with partners in 100 more, we’re your local yet global agency.
Fancy a coffee, virtual or physical? It’s on us – let’s connect!