The importance of master data modeling in data-driven organizations
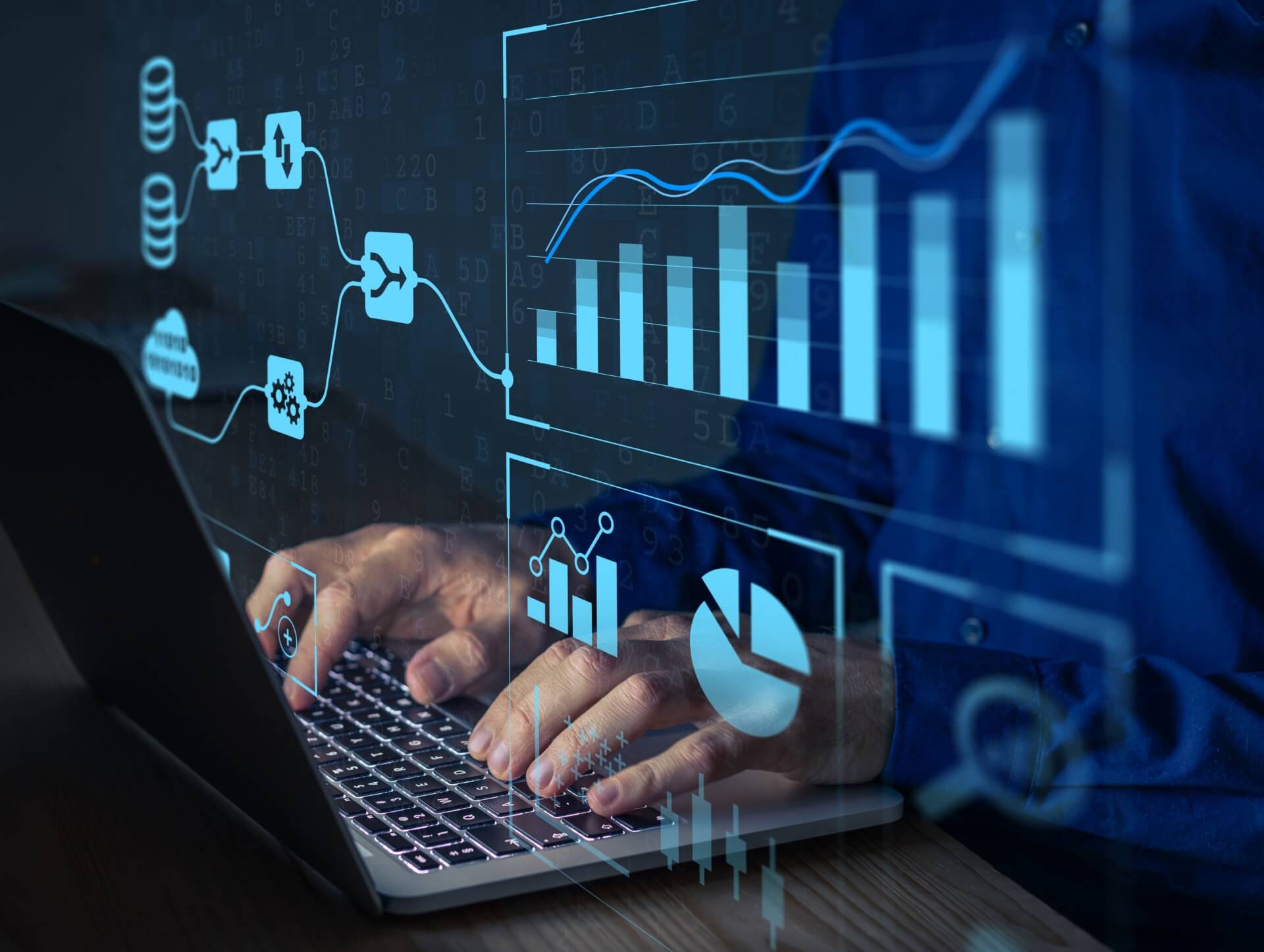
06/09/2023
In today's data-driven world, organizations are constantly collecting and analyzing large amounts of data to gain insights and drive decision-making. However, the value of data lies in its quality and accuracy. Without proper management and modeling of data, organizations may end up making decisions based on faulty or incomplete information. This is where master data modeling comes into play. Master data modeling is a crucial step in the process of master data management (MDM), which focuses on creating a unified and consistent view of an organization's data assets. In this article, we will explore the importance of master data modeling in data-driven organizations and its impact on various aspects of business operations.
Understanding Master Data Modeling
Master data modeling refers to the process of creating a logical representation of an organization's master data entities and their relationships. Master data includes critical information about customers, products, suppliers, employees, and other core entities that are shared across multiple systems and business processes. By creating a comprehensive and accurate model of this data, organizations can ensure consistency, integrity, and quality throughout their data ecosystem. Master data modeling involves identifying key data entities, defining their attributes, and establishing relationships between them. It also includes defining data hierarchies, classifications, and taxonomies to organize and categorize the data effectively.
The Benefits of Master Data Modeling
Master data modeling brings several benefits to data-driven organizations. Let's explore some of the key advantages:
1. Improved Data Quality
Master data modeling helps in improving the overall quality of data. By defining standard data elements, their attributes, and relationships, organizations can ensure data consistency and accuracy across various systems and processes. This, in turn, enhances the reliability and trustworthiness of the data, enabling organizations to make better-informed decisions.
2. Enhanced Data Governance
Effective master data modeling is a critical component of data governance in MDM. It enables organizations to establish data standards, policies, and procedures for managing and governing master data. With a well-defined master data model, organizations can enforce data quality rules, access controls, and data stewardship responsibilities. This ensures that the data is used and managed in a compliant and secure manner throughout its lifecycle.
3. Streamlined Business Processes
Master data modeling helps in streamlining business processes by providing a consistent and unified view of data across the organization. With a well-designed master data model, organizations can eliminate data silos and redundant data entry, leading to improved operational efficiency and productivity. It also enables seamless data integration and interoperability between different systems and applications, allowing for smooth data flow and exchange.
4. Improved Decision-Making
Accurate and reliable data is crucial for making informed business decisions. Master data modeling ensures that the data used for decision-making is consistent, up-to-date, and relevant. With a unified view of master data, organizations can analyze and interpret the data more effectively, leading to better insights and actionable intelligence. This, in turn, helps in driving strategic planning, resource allocation, and performance management.
Master Data Modeling Best Practices
To ensure the success of master data modeling initiatives, organizations should follow certain best practices. Here are some key recommendations:
1. Define Clear Objectives and Scope
Before starting the master data modeling process, organizations should clearly define their objectives and scope. This includes identifying the key master data entities, the attributes to be included, and the relationships to be established. It is essential to involve stakeholders from different business units to understand their requirements and ensure that the model aligns with the organization's strategic goals.
2. Establish Data Governance Framework
A robust data governance framework is crucial for successful master data modeling. Organizations should establish a data governance committee or council responsible for defining data standards, policies, and procedures. This includes data quality rules, data stewardship roles, and responsibilities, and access controls. Regular data governance meetings should be conducted to review and update the master data model based on changing business requirements.
3. Utilize Master Data Management Tools and Technologies
There are several master data management tools and technologies available in the market that can simplify and automate the master data modeling process. These tools provide features like data modeling, data profiling, data integration, and data quality management. Organizations should evaluate and select the right tools based on their specific requirements and budget. Open-source master data management platforms can be a cost-effective option for organizations with limited resources.
4. Ensure Data Quality and Data Security
Data quality management and data security are crucial aspects of master data modeling. Organizations should implement data quality checks and validations to ensure the accuracy, completeness, and consistency of the data. Data security measures like access controls, encryption, and data masking should be implemented to protect sensitive information. Regular data audits and assessments should be conducted to identify and address any data quality or security issues.
Master Data Modeling and Data Analytics
Master data modeling plays a critical role in enabling data analytics in organizations. By creating a unified and consistent view of master data, organizations can perform advanced analytics and derive valuable insights. Master data modeling enables the integration of data from multiple sources, enabling organizations to analyze data across different dimensions and hierarchies. This helps in identifying patterns, trends, and correlations that can drive business growth and innovation.
Master Data Modeling and Customer Experience
In today's customer-centric business landscape, delivering a seamless and personalized customer experience is essential for success. Master data modeling helps organizations in achieving this by creating a 360-degree view of customers. By consolidating customer data from various touchpoints and systems, organizations can gain a holistic understanding of customer preferences, behaviors, and needs. This enables them to tailor their products, services, and marketing strategies to meet individual customer requirements, leading to improved customer satisfaction and loyalty.
Challenges in Master Data Modeling
While master data modeling brings several benefits, it also comes with its own set of challenges. Here are some common challenges faced by organizations in master data modeling:
1. Data Complexity
Master data modeling becomes complex when dealing with large volumes of data and multiple data sources. Organizations often struggle to harmonize and integrate data from disparate systems and applications. Data inconsistencies, duplicates, and conflicts can arise, leading to inaccurate and unreliable data. It requires careful planning, data profiling, and data cleansing to address these challenges and ensure data accuracy and integrity.
2. Data Governance and Data Stewardship
Establishing effective data governance and data stewardship practices is a challenge for many organizations. It requires defining clear roles and responsibilities, ensuring data quality and security, and enforcing data governance policies and procedures. Lack of proper data governance can result in data silos, data duplication, and data inconsistencies, undermining the effectiveness of master data modeling.
3. Data Integration and Interoperability
Integrating and interoperating data from various systems and applications is a complex task. Organizations often struggle with data integration challenges like data mapping, data transformation, and data synchronization. It requires robust data integration tools and technologies to ensure smooth and seamless data flow between different systems and applications.
4. Change Management and User Adoption
Implementing master data modeling initiatives requires change management and user adoption. Organizations need to educate and train their employees on the importance and benefits of master data modeling. Resistance to change and lack of user adoption can hinder the success of master data modeling initiatives.
Case Studies: Real-World Examples
Let's look at some real-world examples of organizations that have successfully implemented master data modeling to drive business value:
1. Company XYZ: Improving Customer Data Quality
Company XYZ, a leading e-commerce company, was facing challenges in maintaining the quality and accuracy of its customer data. Duplicate customer records, incomplete information, and inconsistent data were hampering its marketing and customer service efforts. To address this, the company implemented a master data modeling strategy, which involved defining a comprehensive customer data model, establishing data quality rules, and implementing data cleansing processes. The result was a significant improvement in customer data quality, leading to personalized marketing campaigns, improved customer segmentation, and enhanced customer satisfaction.
2. Company ABC: Streamlining Supply Chain Operations
Company ABC, a global manufacturing company, was struggling with inefficiencies and delays in its supply chain operations. The lack of a unified view of product data and supplier data was causing delays in procurement, inventory management, and order fulfillment. To overcome these challenges, the company implemented a master data modeling initiative, which involved creating a centralized product data model and supplier data model. This enabled seamless integration and interoperability between different systems and applications, streamlining supply chain operations, reducing lead times, and improving overall operational efficiency.
Conclusion
In today's data-driven world, organizations cannot afford to overlook the importance of master data modeling. It is a critical step in the process of master data management, enabling organizations to create a unified and consistent view of their data assets. Proper master data modeling brings several benefits, including improved data quality, enhanced data governance, streamlined business processes, and improved decision-making. However, organizations should be aware of the challenges involved in master data modeling and adopt best practices to overcome them. By leveraging master data modeling effectively, organizations can harness the true potential of their data assets and gain a competitive edge in the market.
Contact us
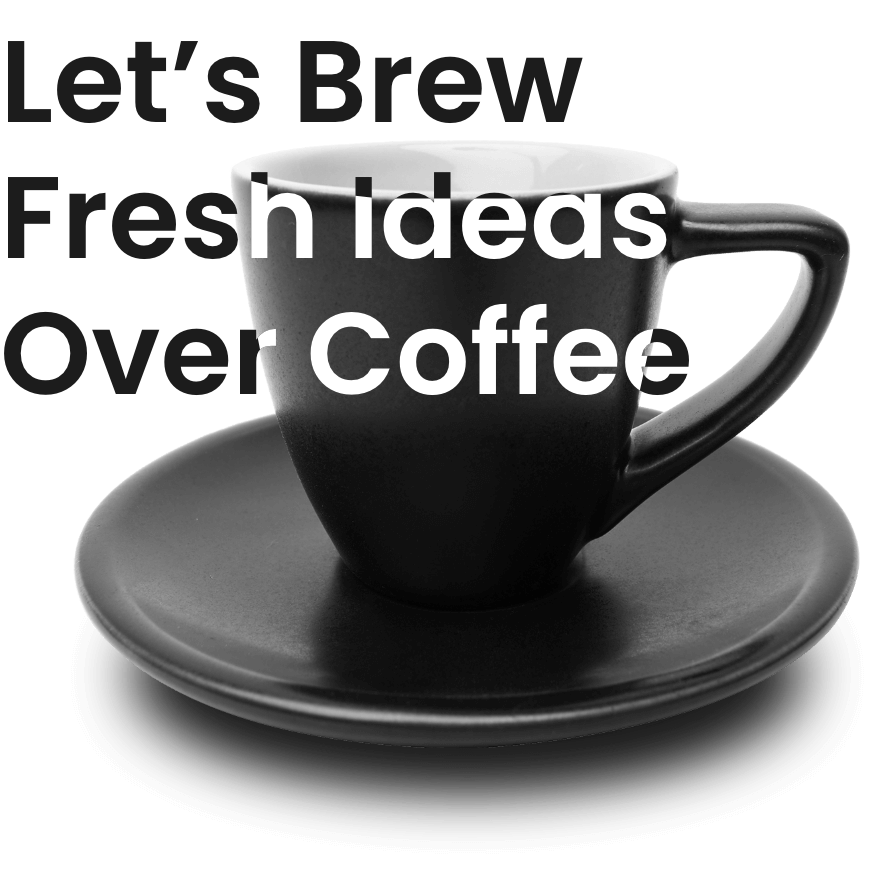
Spanning 8 cities worldwide and with partners in 100 more, we’re your local yet global agency.
Fancy a coffee, virtual or physical? It’s on us – let’s connect!