The importance of master data modeling in data management
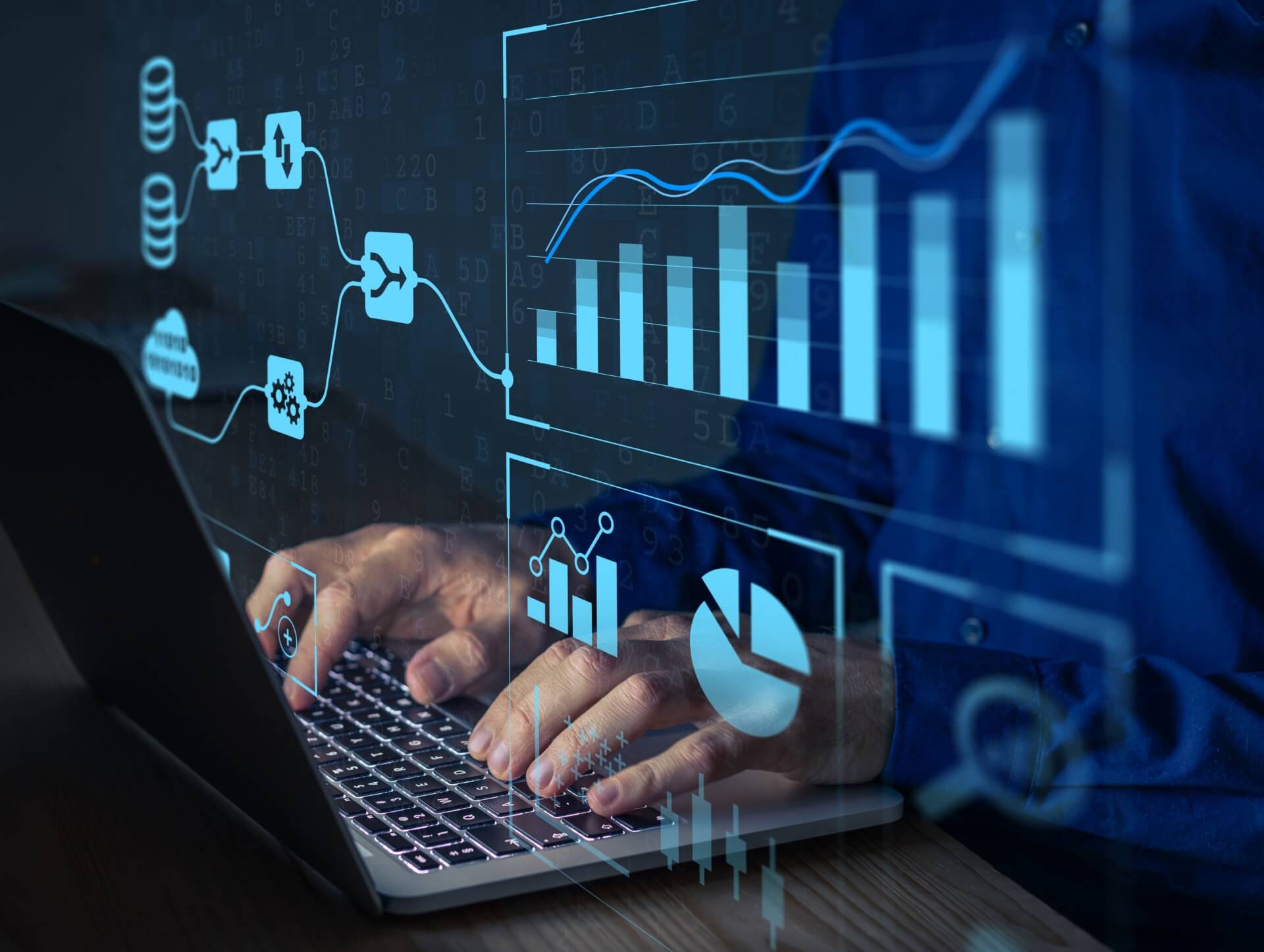
06/09/2023
In today's data-driven world, businesses are constantly faced with the challenge of managing and making sense of vast amounts of data. From customer information to product details, inventory records to financial data, organizations need to ensure that their data is accurate, consistent, and reliable. This is where master data management (MDM) comes into play. MDM is a comprehensive approach to data governance that focuses on creating and maintaining a single, trusted source of master data across an organization.
The Role of Master Data Modeling
At the heart of effective master data management lies master data modeling. Master data modeling involves the creation of a logical representation of an organization's master data entities, attributes, and their relationships. It provides a blueprint for organizing and structuring data in a way that is meaningful and easily accessible. By defining the structure, format, and relationships of master data, organizations can ensure consistency and integrity throughout their data management processes.
Benefits of Master Data Modeling
Implementing a robust master data modeling strategy can bring numerous benefits to organizations:
1. Data Consistency and Accuracy
By establishing a well-defined master data model, organizations can ensure that data is consistent and accurate across different systems and departments. This eliminates data duplication, reduces errors, and improves overall data quality. Consistent and accurate data is essential for making informed business decisions and driving operational efficiency.
2. Improved Data Governance
Master data modeling provides a foundation for effective data governance. It allows organizations to define data ownership, access controls, and data stewardship roles. With a clear understanding of the data's structure and relationships, organizations can implement robust data governance practices to ensure data integrity, security, and compliance.
3. Enhanced Data Integration
Master data modeling facilitates seamless data integration across different systems and applications. By mapping the relationships between different master data entities, organizations can easily consolidate and integrate data from various sources. This enables a holistic view of the data, enabling organizations to gain valuable insights and make data-driven decisions.
4. Increased Operational Efficiency
With a well-designed master data model, organizations can streamline their data management processes and improve operational efficiency. By eliminating data silos and ensuring data consistency, organizations can reduce manual data entry, minimize errors, and automate data integration tasks. This frees up valuable time and resources, allowing employees to focus on more strategic initiatives.
Master Data Modeling Strategies
When it comes to master data modeling, organizations can adopt different strategies based on their specific needs and requirements. Some common strategies include:
1. Entity-Relationship Modeling
Entity-Relationship (ER) modeling is a popular approach to master data modeling. It involves identifying the main entities in the data model and defining their attributes and relationships. Entities can represent various master data domains such as customers, products, suppliers, or employees. ER modeling provides a visual representation of the data structure, making it easier to understand and communicate.
2. Hierarchical Modeling
Hierarchical modeling focuses on representing the master data as a hierarchy or tree-like structure. This approach is particularly useful when dealing with data that has a natural hierarchical relationship, such as organizational structures or product categories. Hierarchical modeling allows for easy navigation and drill-down analysis of the data.
3. Dimensional Modeling
Dimensional modeling is commonly used in data warehousing and business intelligence applications. It involves organizing data into dimensions and facts. Dimensions represent the descriptive attributes of the data, while facts represent the numerical measures. Dimensional modeling enables efficient querying and analysis of large volumes of data.
4. Semantic Modeling
Semantic modeling focuses on capturing the meaning and context of the data. It involves defining concepts, relationships, and business rules that govern the data. Semantic models provide a common understanding of the data across the organization, enabling effective collaboration and data sharing.
Best Practices for Master Data Modeling
To ensure successful master data modeling, organizations should follow these best practices:
1. Understand Business Requirements
Before embarking on the master data modeling process, it is important to understand the business requirements and objectives. This includes identifying the key master data entities, their attributes, and the relationships between them. Engage with business stakeholders to gather their input and ensure that the model aligns with their needs.
2. Standardize Data Definitions
Standardizing data definitions is crucial for ensuring consistency and accuracy. Define clear and consistent naming conventions, data types, and formats for the master data attributes. This will help avoid confusion and misinterpretation of the data.
3. Establish Data Governance Framework
Data governance is essential for maintaining data quality and integrity. Establish a data governance framework that includes data ownership, data stewardship, and data quality management processes. Clearly define roles and responsibilities to ensure accountability and compliance.
4. Collaborate with IT and Business Teams
Effective master data modeling requires collaboration between IT and business teams. IT teams are responsible for implementing the technical aspects of the model, while business teams provide domain expertise and input. Foster open communication and collaboration to ensure that the model meets both technical and business requirements.
5. Regularly Update and Maintain the Model
Master data modeling is an ongoing process. As the business evolves and new data requirements emerge, the model needs to be updated and maintained. Regularly review and refine the model to ensure its relevance and accuracy.
Conclusion
Master data modeling plays a crucial role in effective data management and governance. By creating a logical representation of an organization's master data, organizations can ensure data consistency, accuracy, and integration. With the right strategies, tools, and best practices in place, organizations can unlock the full potential of their data and drive business growth. Invest in master data management solutions and embrace the power of master data modeling to stay ahead in today's data-driven world.
Contact us
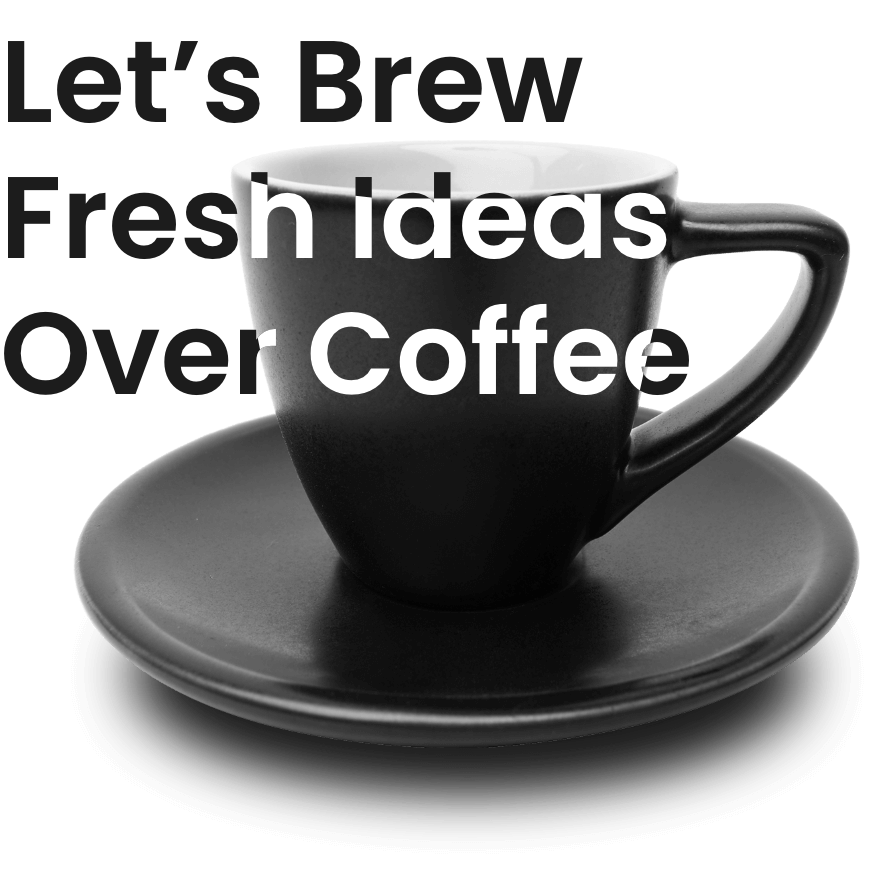
Spanning 8 cities worldwide and with partners in 100 more, we’re your local yet global agency.
Fancy a coffee, virtual or physical? It’s on us – let’s connect!