The role of data analytics in master data management
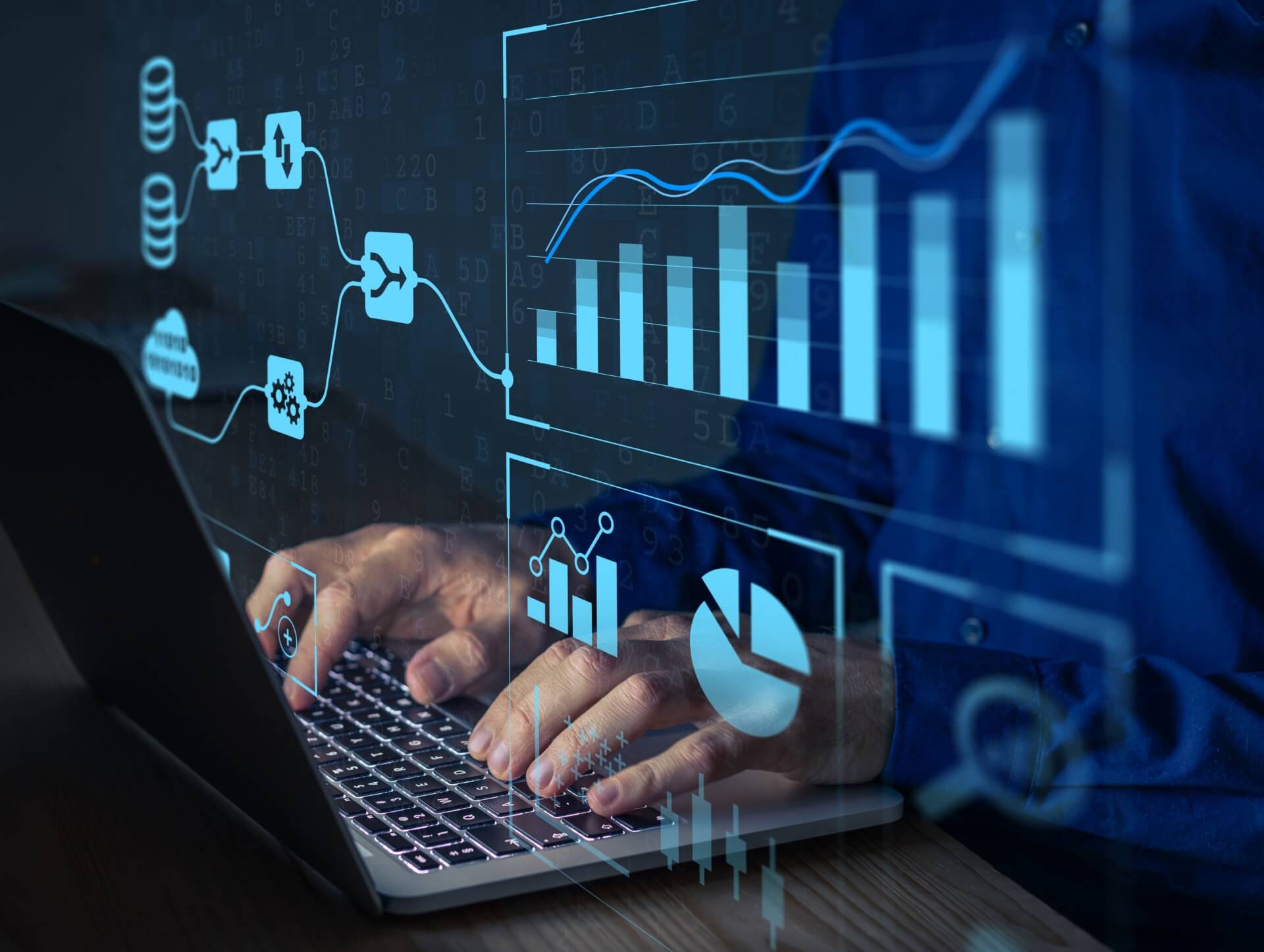
06/09/2023
Master Data Management (MDM) is a comprehensive approach to managing and ensuring the accuracy, consistency, and reliability of an organization's critical data. It involves the creation and maintenance of a single, trusted source of master data that can be shared across different systems, applications, and business processes.
MDM plays a crucial role in enabling organizations to make informed business decisions, improve operational efficiency, enhance customer experience, and comply with regulatory requirements. However, the success of MDM largely depends on the quality and accuracy of the data being managed. This is where data analytics comes into play.
Data analytics, also known as data analysis, is the process of examining, cleaning, transforming, and modeling data in order to discover useful information, draw conclusions, and support decision-making. When applied to MDM, data analytics helps organizations gain valuable insights from their master data, identify patterns and trends, and make data-driven decisions.
The Benefits of Data Analytics in Master Data Management
Data analytics brings several benefits to the field of master data management. Let's explore some of the key advantages:
1. Improved Data Quality
Data analytics techniques, such as data profiling and data cleansing, can help organizations identify and rectify data quality issues in their master data. By analyzing the data and identifying anomalies, duplicates, and inconsistencies, organizations can take corrective actions and improve the overall quality of their master data. This, in turn, leads to better decision-making, increased operational efficiency, and enhanced customer satisfaction.
2. Enhanced Data Governance
Data governance is a critical component of MDM that involves establishing policies, procedures, and controls to ensure the integrity, privacy, and security of data. Data analytics can play a significant role in supporting data governance efforts. By analyzing data usage patterns, access logs, and user behavior, organizations can identify potential data governance issues and take proactive measures to address them. This helps in maintaining data compliance and mitigating data-related risks.
3. Optimized Data Integration
Data integration is a key challenge in MDM, as organizations need to consolidate data from various sources and systems into a single, unified view. Data analytics can help organizations streamline the data integration process by identifying common data elements, mapping data relationships, and resolving data conflicts. This ensures that the master data is accurate, consistent, and up-to-date, enabling organizations to make more informed decisions and improve operational efficiency.
4. Improved Customer Experience
MDM plays a crucial role in managing customer data and ensuring a personalized and seamless customer experience. Data analytics helps organizations gain insights into customer behavior, preferences, and needs by analyzing customer data collected from various touchpoints. This enables organizations to deliver targeted marketing campaigns, personalized recommendations, and superior customer service, thereby improving the overall customer experience.
Data Analytics Techniques in Master Data Management
In order to leverage the benefits of data analytics in MDM, organizations need to employ various data analytics techniques. Let's explore some of the key techniques:
1. Data Profiling
Data profiling is the process of analyzing and assessing the quality, completeness, and consistency of data. It involves examining the structure, content, and relationships within the data to identify errors, anomalies, and inconsistencies. Data profiling helps organizations understand the data they have, identify data quality issues, and take appropriate actions to improve the quality of their master data.
2. Data Cleansing
Data cleansing, also known as data scrubbing or data cleaning, is the process of identifying and correcting or removing errors, duplicates, and inconsistencies in data. It involves techniques such as standardization, validation, and de-duplication to ensure that the data is accurate, consistent, and reliable. Data cleansing helps organizations improve the quality of their master data, eliminate data redundancies, and reduce the risk of making decisions based on incorrect or incomplete data.
3. Data Modeling
Data modeling is the process of creating a conceptual, logical, and physical representation of the data. It involves defining the structure, relationships, and constraints of the data in order to facilitate data integration, data storage, and data retrieval. Data modeling helps organizations understand their master data, identify data dependencies, and design databases and systems that can effectively store and manage the data.
4. Predictive Analytics
Predictive analytics is a branch of data analytics that uses statistical models and machine learning algorithms to predict future outcomes based on historical data. In the context of MDM, predictive analytics can be used to forecast customer behavior, identify potential data quality issues, and optimize data integration processes. By analyzing patterns and trends in the data, organizations can make data-driven predictions and take proactive measures to address potential challenges.
Challenges in Data Analytics for Master Data Management
While data analytics brings numerous benefits to MDM, it also poses certain challenges that organizations need to overcome. Let's discuss some of the key challenges:
1. Data Quality Management
Data quality management is a critical aspect of MDM that involves ensuring the accuracy, completeness, consistency, and timeliness of data. Data analytics can help organizations identify and rectify data quality issues, but it also requires significant effort and resources. Organizations need to invest in data quality tools, establish data quality metrics, and define data quality rules in order to effectively manage data quality in MDM.
2. Data Security and Privacy
Data security and privacy are major concerns in MDM, as organizations need to ensure that their master data is protected from unauthorized access, manipulation, and misuse. Data analytics involves analyzing and processing sensitive data, which increases the risk of data breaches and privacy violations. Organizations need to implement robust data security measures, such as encryption, access controls, and data masking, to safeguard their master data and comply with data protection regulations.
3. Data Integration Complexity
Data integration is a complex and time-consuming process in MDM, as organizations need to consolidate data from various sources and systems. Data analytics can help organizations streamline the data integration process, but it also requires expertise in data modeling, data mapping, and data transformation. Organizations need to invest in data integration tools and technologies, as well as train their staff in data integration best practices, to effectively manage the complexities of data integration in MDM.
4. Data Governance and Compliance
Data governance is a critical component of MDM that involves establishing policies, procedures, and controls to ensure the integrity, privacy, and security of data. Data analytics can support data governance efforts by providing insights into data usage, access patterns, and user behavior. However, organizations need to define data governance frameworks, assign data stewardship responsibilities, and implement data governance tools and technologies to ensure compliance with data governance policies and regulations.
Data Analytics in Action: Case Studies
Let's take a look at some real-world case studies that highlight the role of data analytics in MDM:
1. Company XYZ: Improving Customer Segmentation
Company XYZ, a leading retail organization, implemented a data analytics solution to improve their customer segmentation for targeted marketing campaigns. By analyzing customer data, transaction history, and online behavior, they were able to identify distinct customer segments and tailor their marketing strategies accordingly. This resulted in higher customer engagement, increased sales, and improved customer satisfaction.
2. Company ABC: Enhancing Data Quality
Company ABC, a global manufacturing company, faced data quality issues in their master data, which affected their operational efficiency and decision-making. They implemented a data analytics solution to analyze their master data, identify data quality issues, and take corrective actions. By improving the quality of their master data, they were able to streamline their business processes, reduce errors and redundancies, and improve overall operational efficiency.
3. Company DEF: Optimizing Data Integration
Company DEF, a financial services organization, struggled with data integration challenges as they had data scattered across multiple systems and departments. They implemented a data analytics solution to analyze their data sources, identify common data elements, and map data relationships. By streamlining their data integration processes, they were able to create a single, unified view of their master data, improve data accuracy, and enable faster and more informed decision-making.
Conclusion
Data analytics plays a vital role in master data management by helping organizations improve data quality, enhance data governance, optimize data integration, and improve customer experience. By leveraging data analytics techniques and tools, organizations can gain valuable insights from their master data, make data-driven decisions, and achieve their MDM goals. However, organizations must also address the challenges associated with data analytics, such as data quality management, data security, data integration complexity, and data governance. By overcoming these challenges and adopting best practices, organizations can maximize the benefits of data analytics in MDM and drive business success.
Contact us
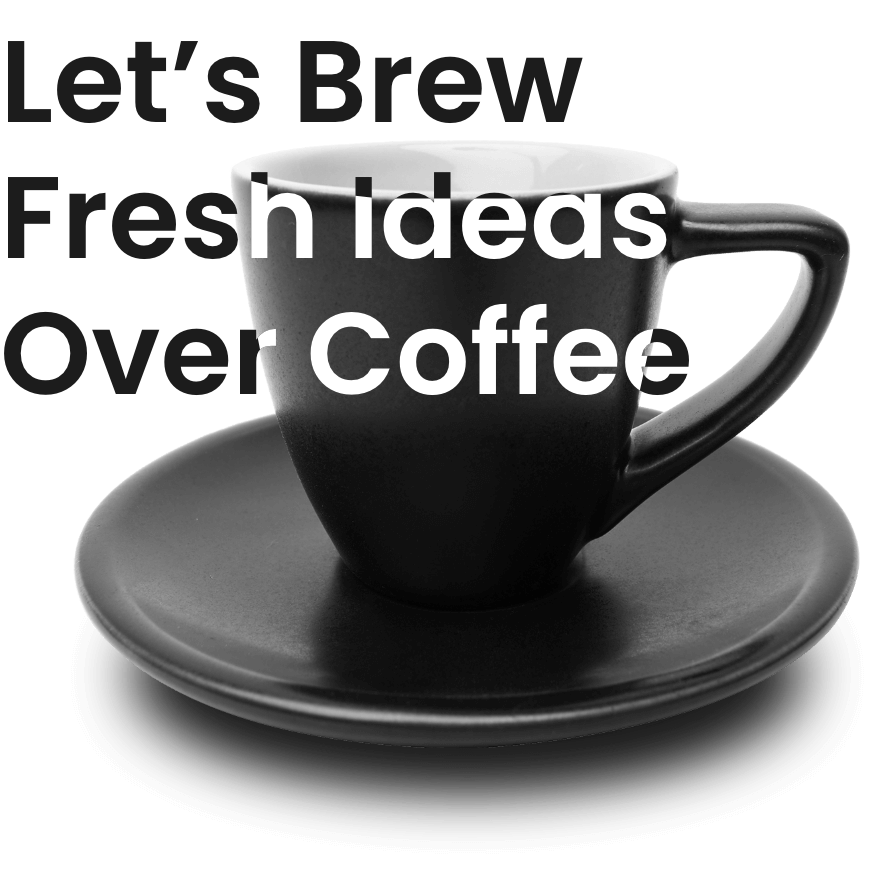
Spanning 8 cities worldwide and with partners in 100 more, we’re your local yet global agency.
Fancy a coffee, virtual or physical? It’s on us – let’s connect!